Unveiling Smartphone-based Deep Learning Models for Point-of-Care Malaria Diagnosis
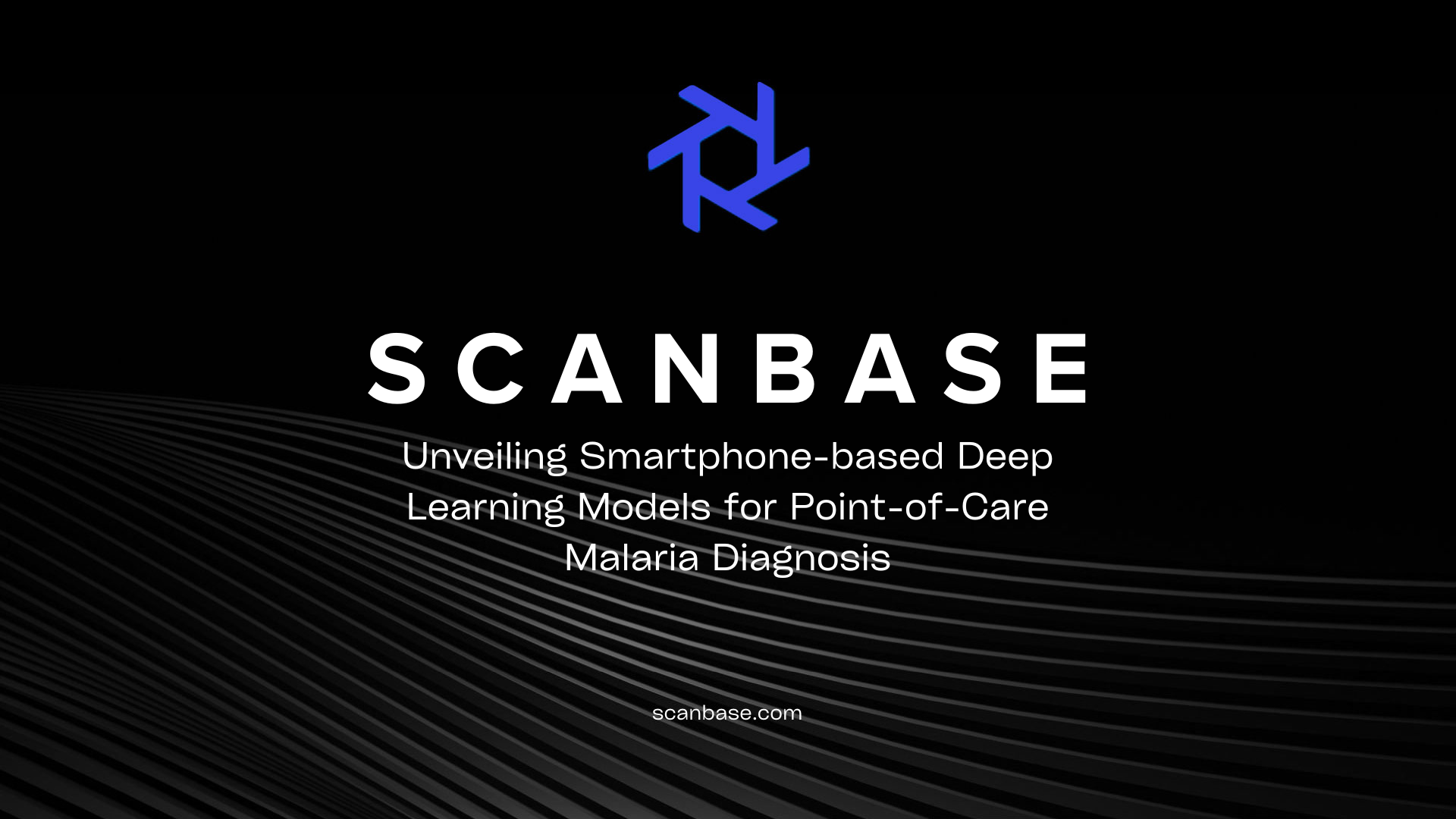
Malaria is a life-threatening disease that affects millions of people worldwide. Early detection and treatment of malaria are crucial in reducing its spread and mortality rates. Smartphone-based deep learning models for point-of-care malaria diagnosis have emerged as a game-changer in healthcare technology, enabling faster and more accurate diagnosis of malaria. These models utilize deep learning algorithms to analyze medical images and identify malaria parasites in blood samples.
The development and implementation of smartphone-based deep learning models have opened up new possibilities for point-of-care malaria diagnosis. This technology is revolutionizing healthcare by providing accessible, cost-effective, and real-time data collection, thereby improving early detection and treatment of malaria.
Key Takeaways:
- Smartphone-based deep learning models have emerged as a game-changer in healthcare technology for point-of-care malaria diagnosis.
- Deep learning algorithms utilized in these models provide faster and more accurate malaria diagnosis.
- This technology has opened up new possibilities for accessible, cost-effective, and real-time data collection in healthcare, thereby improving early detection and treatment of malaria.
Advancements in Mobile Health Technology
In recent years, mobile health technology has made significant strides in revolutionizing healthcare. With the widespread availability of smartphones, these devices have become highly accessible and are now being utilized for medical diagnosis and treatment.
One of the major advancements in mobile health technology is the development of smartphone-based diagnosis. With the use of deep learning algorithms, smartphones can now analyze medical images and provide accurate diagnoses in real-time. This technology has the potential to improve patient outcomes by enabling early detection and prompt treatment of diseases.
Additionally, the capabilities of smartphones, such as their high-resolution cameras and powerful processing capabilities, make them well-suited for medical diagnosis. The use of smartphones in medical settings also eliminates the need for specialized equipment, making healthcare more accessible and cost-efficient.
While mobile health technology and smartphone-based diagnosis have many potential benefits, there are also some limitations to consider. One concern is the accuracy of diagnoses, as this technology is still developing and may not be as reliable as traditional diagnosis methods. Additionally, patient privacy and data security must be addressed to ensure responsible use of this technology.
Overall, the advancements in mobile health technology and smartphone-based diagnosis are promising and have the potential to greatly improve healthcare outcomes. As this technology continues to develop, it will be important to maintain a balance between accessibility, accuracy, and ethical considerations.
Understanding Deep Learning Algorithms
Deep learning algorithms are a subset of machine learning that involves training artificial neural networks to recognize patterns in data. These algorithms are able to learn from large datasets and improve their performance with experience, similar to how humans learn from experience.
Deep learning algorithms are becoming increasingly prevalent in the field of medical diagnosis due to their ability to analyze complex medical images and identify patterns that may be difficult to detect by human doctors. In addition, these algorithms allow for rapid and accurate diagnosis, which can be critical in time-sensitive medical situations.
Artificial intelligence for medical diagnosis, including deep learning algorithms, has the potential to significantly improve healthcare outcomes by providing faster and more accurate diagnoses. In the case of malaria, this technology can aid in the early detection and treatment of the disease, which can be life-saving.
However, it is important to note that these algorithms are not infallible and may produce errors or false positives. It is crucial that they are used in conjunction with human doctors and that ethical considerations are taken into account when implementing this technology in medical settings.
The Need for Point-of-Care Malaria Diagnosis
Malaria is a life-threatening disease that affects millions of people globally, particularly in sub-Saharan Africa. Traditional diagnosis methods involve laboratory testing, which can be time-consuming, expensive, and often unavailable in remote areas. This delays the initiation of treatment and can result in severe complications and even death.
Point-of-care malaria diagnosis is crucial to provide timely and effective treatment. However, the challenges faced in traditional diagnosis methods can make it difficult to achieve this goal. Smartphone-based deep learning models are an innovative approach that can address these challenges and provide faster and more accurate diagnosis.
The use of smartphone-based diagnosis for malaria has the potential to revolutionize healthcare in many ways. The technology can provide widespread accessibility, especially in rural and remote areas, where traditional diagnosis methods are often unavailable. It can also improve cost-effectiveness, as deep learning algorithms can analyze medical images and provide accurate diagnoses without the need for extensive laboratory testing.
Moreover, real-time data collection enabled by smartphone-based diagnosis can provide valuable insights into the spread and severity of the disease, enabling healthcare professionals to develop more effective prevention and treatment strategies.
Despite these benefits, concerns about accuracy and privacy remain. It is important to develop guidelines and regulatory frameworks to ensure responsible use of this technology and protect patient privacy and data security.
Machine Learning for Malaria Diagnosis
Machine learning algorithms have shown great promise in improving the accuracy of malaria diagnosis. With the ability to analyze medical images, machine learning models can detect even the smallest traces of the malaria parasite in blood samples.
One study conducted in Thailand demonstrated the potential of machine learning models in identifying malaria parasites in blood samples with an accuracy rate of 93.7%. This is a significant improvement compared to traditional diagnosis methods, which have been shown to have an accuracy rate of around 75%.
Another advantage of machine learning for malaria diagnosis is the ability to detect malaria in its early stages. By analyzing changes in blood cells that occur before symptoms present, machine learning models can detect malaria in its asymptomatic stage, allowing for earlier treatment and prevention of severe complications.
- SEO keywords: machine learning for malaria diagnosis
Image Recognition for Medical Diagnosis
Image recognition is a technology that enables machines to analyze, understand, and interpret images. In the field of medical diagnosis, image recognition algorithms can be trained to identify specific features and patterns in medical images, such as X-rays, CT scans, and blood smears. This has significant implications for the accurate and prompt diagnosis of diseases like malaria.
In the case of malaria diagnosis, image recognition algorithms can be used to identify and count malaria parasites in blood samples. This is an important step in the traditional method of diagnosing malaria, which involves examining blood samples under a microscope. With the help of image recognition algorithms, the accuracy and speed of this process can be greatly improved, reducing the time and resources required for diagnosis.
Furthermore, image recognition algorithms can also be used to detect malaria parasites in images captured by smartphone cameras. By using an appropriate imaging device and software, a smartphone can capture high-quality images of a blood smear and transmit them to an image recognition algorithm. This enables the development of smartphone-based deep learning models for malaria diagnosis, which can be used at the point-of-care.
Overall, image recognition technology has the potential to revolutionize the way we diagnose and treat diseases like malaria, making diagnosis faster, more accurate, and more accessible.
Development and Implementation of Smartphone-based Deep Learning Models
Developing smartphone-based deep learning models for malaria diagnosis is a complex process that requires significant expertise and resources. The first step is to collect large datasets of blood smear images, annotate them, and then use them to train deep learning algorithms.
The next step involves optimizing the trained algorithm for use on mobile devices to enable real-time diagnosis. This requires reducing the algorithm's size while maintaining its accuracy. Once optimized, the algorithm is integrated into a smartphone application that is then validated in clinical settings to ensure its effectiveness.
Key Considerations | Challenges |
---|---|
Data collection: Collecting sufficient and diverse datasets of blood samples. | Access to high-quality datasets can be limited in some geographic regions. |
Algorithm optimization: Reducing the size of the algorithm while maintaining accuracy. | Optimization can be time-consuming and computationally expensive, requiring specialized skills. |
Clinical validation: Ensuring the algorithm's effectiveness in clinical settings. | Validating the algorithm requires large-scale clinical trials, which can be resource-intensive and time-consuming. |
Despite these challenges, there have been successful case studies of smartphone-based deep learning models for malaria diagnosis, demonstrating the potential of this technology to improve healthcare and increase accessibility to reliable diagnostic tools.
Benefits and Limitations of Smartphone-based Diagnosis
Smartphone-based diagnosis for malaria has several benefits and limitations that must be considered. One significant benefit is the potential for widespread accessibility. With the widespread use of smartphones worldwide, this technology could provide diagnosis in remote or underserved areas. Additionally, smartphone-based diagnosis has the potential to be cost-effective, eliminating the need for expensive laboratory equipment and specialized technicians.
Another benefit of smartphone-based diagnosis is the ability to collect real-time data. By utilizing deep learning algorithms, these models can continuously learn and improve accuracy over time, providing valuable insights into malaria diagnosis trends and outbreaks.
However, there are also limitations to smartphone-based diagnosis. One major concern is accuracy. While deep learning algorithms have shown promise in detecting malaria parasites in blood samples, there is still a risk of false positives or false negatives. Additionally, smartphones may not have the same level of sensitivity and specificity as traditional laboratory equipment, which could lead to inaccurate diagnosis.
Another limitation to consider is patient privacy and data security. As with any healthcare technology, there is a risk of data breaches or misuse of patient data. It is essential to establish guidelines and regulations to ensure responsible use of smartphone-based diagnosis and protect patient privacy.
In conclusion, smartphone-based diagnosis has the potential to revolutionize malaria diagnosis and improve healthcare accessibility worldwide. However, it is important to consider the limitations and challenges associated with this technology and work towards responsible implementation.
Future Implications and Potential Applications
The development of smartphone-based deep learning models for point-of-care malaria diagnosis has opened up new possibilities in healthcare. The potential for this technology to improve early detection and treatment of malaria is significant, and there are many other potential applications of this technology in healthcare.
Potential Applications
Smartphone-based deep learning models have the potential to be used in a variety of healthcare settings, from remote areas with limited access to medical facilities to hospitals and clinics. They can be used not only for the diagnosis of malaria but for other diseases as well, such as tuberculosis and cancer. Additionally, these models can be used for the analysis of medical images, allowing for more accurate diagnoses and treatment.
The use of deep learning algorithms in medical diagnosis can also improve the overall efficiency of healthcare systems. By providing faster and more accurate diagnosis, resources can be allocated more effectively, reducing costs and improving patient outcomes.
Challenges and Considerations
While the potential for smartphone-based deep learning models is significant, there are also challenges and considerations to be addressed. Ethical considerations, such as patient privacy and data security, must be addressed when using this technology. Additionally, regulatory frameworks must be developed to ensure responsible use of this technology.
There are also technological challenges to be addressed, such as ensuring that the models are accurate and reliable, and that they can be used in a variety of healthcare settings. Further research and development is needed to ensure that these models can be used safely and effectively.
Conclusion
The development of smartphone-based deep learning models has the potential to revolutionize healthcare by improving early detection and treatment of diseases such as malaria. While there are challenges and considerations to be addressed, the benefits of this technology are significant. Further research and development in this field is necessary to ensure that this technology can be used safely and effectively in healthcare settings around the world.
Ethical Considerations and Regulatory Frameworks
As with any new technology, smartphone-based diagnosis raises important ethical considerations and regulatory frameworks. One of the major concerns is patient privacy, particularly with the collection and storage of personal health data.
Healthcare providers must take appropriate measures to secure patients' sensitive information and ensure that it is not misused or shared without consent. Additionally, regulations must be in place to prevent discrimination against individuals based on their health status.
Another area of concern is the accuracy of smartphone-based diagnosis. While these models have shown promising results, it is crucial that they are thoroughly tested and validated before being implemented on a large scale.
Furthermore, regulations must be in place to ensure that the use of these models does not replace traditional medical diagnosis, but rather serves as a complementary tool for healthcare providers.
It is important for healthcare providers, researchers, and policymakers to work together to establish guidelines and regulations that promote responsible use of smartphone-based diagnosis.
The potential benefits of this technology, such as increased accessibility and cost-effectiveness, must be weighed against the potential risks and ethical concerns.
Conclusion
In conclusion, the development of smartphone-based deep learning models for point-of-care malaria diagnosis is a significant advancement in healthcare technology. The use of mobile health technology and deep learning algorithms has the potential to revolutionize the diagnosis and treatment of diseases, particularly in resource-limited settings.
The need for point-of-care malaria diagnosis is critical, and traditional diagnosis methods face significant challenges. Smartphone-based deep learning models offer a faster and more accurate alternative, with the potential for widespread accessibility and cost-effectiveness.
Although there are limitations and ethical considerations to be addressed, the potential applications of this technology are vast. The expansion of smartphone-based deep learning models to other diseases and healthcare settings is an exciting prospect. As such, it is essential to continue researching and developing this field to improve healthcare outcomes worldwide.
Stay Informed
To keep up with the latest advancements in technology and healthcare, stay tuned for more articles and updates on Smartphone-based Deep Learning Models for Point-of-Care Malaria Diagnosis.