Accuracy of Smartphone-based Computer Vision in RDT Interpretation Explained
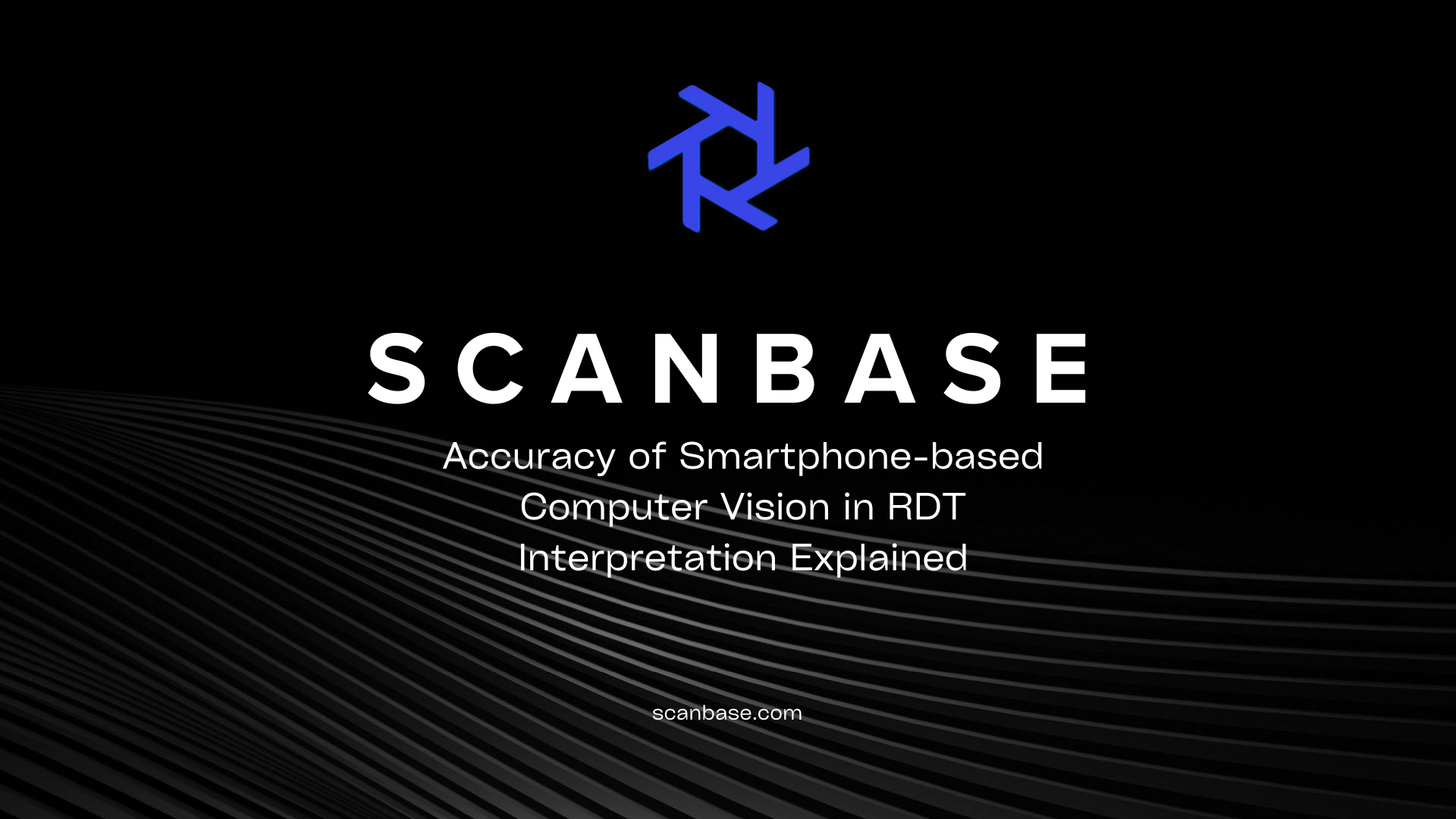
In today's fast-paced world, healthcare professionals face numerous challenges when it comes to diagnostic testing. Rapid diagnostic tests (RDTs) offer a quick and cost-effective solution, but accurate interpretation remains of utmost importance. Smartphone-based computer vision technology is a solution that can enhance the accuracy of RDT interpretation, making it a valuable tool for healthcare diagnostics.
With the help of computer vision algorithms, smartphones can analyze images captured from RDTs and provide accurate interpretations. This technology can provide a convenient and cost-effective way to improve diagnostic capabilities, particularly in remote or resource-limited settings where traditional laboratory-based testing may not be available.
Key Takeaways:
- Accuracy of RDT interpretation is crucial in the healthcare industry.
- Smartphone-based computer vision technology can enhance the accuracy of RDT interpretation.
- Computer vision algorithms can analyze RDT images for accurate interpretation.
- Smartphone-based RDT interpretation is a cost-effective and convenient solution for healthcare diagnostics.
Understanding Smartphone-based Computer Vision for RDT Analysis
Smartphone-based computer vision technology has emerged as a potential game-changer in the field of medical diagnostics. It involves using the camera and processing power of a smartphone to analyze images and data, enabling accurate interpretation of rapid diagnostic tests (RDTs).
Computer vision algorithms are at the heart of this technology, providing the ability to detect and interpret visual information from RDTs. These algorithms are designed to recognize patterns and features in the images captured by the smartphone camera and make informed decisions based on the identified data.
By leveraging smartphone technology, clinicians and healthcare professionals can rapidly and accurately analyze RDTs using a portable, accessible, and cost-effective tool. The technology has the potential to revolutionize healthcare delivery, particularly in remote or low-resource settings where traditional laboratory-based diagnostic testing may be unavailable or impractical.
How Smartphone Computer Vision Technology Works
Smartphone-based computer vision technology works by taking advantage of the powerful cameras and processing capabilities of modern smartphones. The process involves capturing an image of the RDT using the smartphone camera and analyzing the image using computer vision algorithms.
The algorithms are trained to detect specific features of the RDT, such as the presence of antibodies, antigens, or other biomarkers. The technology can then use this information to identify and interpret the test results, providing accurate and reliable diagnostic information.
Computer vision technology also allows for real-time analysis of RDTs, enabling healthcare professionals to quickly and efficiently interpret test results without the need for specialized equipment or resources. This aspect of smartphone-based computer vision technology is particularly advantageous in emergency or critical care settings where time is of the essence.
Improving RDT Interpretation with Computer Vision
The accuracy of RDT interpretation is crucial for proper diagnosis and treatment of diseases. Unfortunately, healthcare professionals may struggle with RDT interpretation due to various factors such as inadequate training, poor lighting conditions, and subjective interpretation. However, recent advancements in computer vision technology can help improve the accuracy and efficiency of RDT interpretation.
Computer vision algorithms can analyze RDT images to accurately detect and diagnose diseases. By using smartphones as the platform for this technology, healthcare professionals can easily capture and process RDT images in real-time. This improves the rapidity of diagnosis, allowing for earlier intervention and treatment.
Moreover, computer vision algorithms can decrease the subjectivity in RDT interpretation by providing an objective analysis of the test results. This reduces the potential for errors caused by human bias or misinterpretation of the test results.
By leveraging computer vision technology, healthcare professionals can improve the accuracy and reliability of RDT interpretation, leading to better patient outcomes and reducing the burden on healthcare systems.
The Role of Smartphone-based Computer Vision in Healthcare
While smartphone-based computer vision technology has shown great potential in interpreting rapid diagnostic tests (RDTs), its applications in healthcare extend beyond this. As a cost-effective, widely accessible platform for imaging and analysis, smartphones have the potential to revolutionize healthcare diagnostics and monitoring.
One key benefit of smartphone-based computer vision is its potential for remote patient monitoring. By leveraging the processing power of smartphones, healthcare providers can monitor patients' vital signs and symptoms from afar, reducing the need for in-person appointments and increasing accessibility for patients in remote or underserved areas.
In addition, smartphone-based computer vision technology has the potential to improve the accuracy and efficiency of telemedicine. With the ability to capture high-quality images and analyze them using computer vision algorithms, healthcare providers can diagnose and treat patients remotely with greater accuracy and confidence.
Beyond diagnostics, smartphone-based computer vision technology can also aid in medical research and drug development. By enabling high-throughput screening and analysis of large datasets, this technology has the potential to accelerate the discovery and development of new treatments for various diseases and conditions.
As smartphone-based computer vision technology continues to evolve and improve, its applications in healthcare are likely to expand even further. From remote patient monitoring to drug development, this innovative approach has the potential to transform the healthcare industry and improve patient outcomes.
Challenges and Limitations of Smartphone-based Computer Vision
While smartphone-based computer vision technology holds immense potential in improving RDT interpretation, it is not without its challenges and limitations. The reliability and accuracy of the technology depend on various factors that can impact its performance. Here are some of the challenges and limitations of smartphone-based computer vision:
Challenge/Limitation | Description |
---|---|
Lighting Conditions | The accuracy of computer vision algorithms can be impacted by the lighting conditions in which images of RDTs are captured. Poor lighting, shadows, or glare can affect the quality of the image, potentially leading to inaccurate interpretation. |
Image Quality | The quality of the image captured by the smartphone camera can also impact the accuracy of the interpretation. Factors such as clarity, focus, and resolution can impact the ability of the algorithm to analyze the image accurately. |
Algorithm Accuracy | The accuracy of the computer vision algorithms themselves can also be a limitation. While constantly improving, these algorithms may not always detect subtle variations or changes in color that could affect the interpretation of the RDT. |
Despite these challenges and limitations, smartphone-based computer vision technology continues to evolve and improve in accuracy and reliability. As technology advances, these challenges are expected to be overcome, making smartphone-based RDT interpretation a more widely used and efficient tool in healthcare diagnosis.
Recent Innovations in Smartphone-based Computer Vision
As smartphone-based computer vision technology evolves, researchers and developers are exploring new ways to improve RDT interpretation and enhance healthcare diagnostics. Here are some recent innovations that are generating excitement in the field:
Innovation | Description |
---|---|
Deep Learning Algorithms | Advancements in deep learning algorithms are enabling smartphones to analyze RDTs with higher accuracy and speed, improving diagnostic capabilities in low-resource settings. |
Cloud-based Image Processing | Cloud-based image processing allows for more complex algorithms to be used in RDT interpretation, increasing accuracy and expanding the range of tests that can be performed with smartphone-based computer vision technology. |
Integration with Wearable Devices | Some researchers are exploring the potential for integrating smartphone-based RDT interpretation with wearable devices, such as smartwatches, to enable remote patient monitoring with greater accuracy and ease. |
Another area of innovation is the use of spectral imaging, which involves capturing the entire spectrum of light reflected by an RDT to detect subtle changes in color that may not be visible to the naked eye. This technique has shown promise in increasing the sensitivity and specificity of RDT interpretation, particularly in cases where the test line is faint or difficult to discern.
Overall, these recent innovations in smartphone-based computer vision hold promise for improving diagnostic capabilities, expanding access to healthcare, and ultimately improving health outcomes for individuals and populations around the world.
Success Stories and Case Studies
Smartphone-based computer vision technology has already shown promising results in improving the accuracy and efficiency of RDT interpretation. Here are a few success stories and case studies that demonstrate the potential impact of this innovative approach in healthcare settings:
Case Study 1: Malaria Diagnosis in Cameroon
In Cameroon, where malaria is one of the leading causes of death, health workers are using smartphone-based computer vision technology to diagnose the disease in remote rural areas. In a study conducted by researchers at the University of California, Berkeley, the accuracy of the smartphone-based RDT interpretation was found to be comparable to that of trained medical professionals.
"The system we’re developing can help address the gap in malaria diagnosis in remote settings where laboratory tests are not available," said Laura Waller, professor of electrical engineering and computer science at UC Berkeley.
This technology has the potential to revolutionize malaria diagnosis in resource-limited settings, where access to trained medical professionals and laboratory tests may be scarce.
Case Study 2: HIV Testing in Rwanda
In Rwanda, researchers have developed a smartphone-based system that can diagnose HIV in minutes using a cheap, disposable RDT and a smartphone camera. The system uses computer vision algorithms to analyze the test results and provide an accurate diagnosis.
According to a study published in the Journal of Medical Internet Research, the smartphone-based RDT interpretation had a sensitivity of 92.6% and a specificity of 99.2%, making it a reliable option for HIV testing in low-resource settings.
Success Story: COVID-19 Testing in India
During the COVID-19 pandemic, India faced a shortage of trained healthcare professionals and laboratory testing facilities. To address this issue, researchers at the Indian Institute of Technology (IIT) Delhi developed a smartphone-based RDT interpretation system for COVID-19.
The system, which used computer vision algorithms to analyze the RDTs, was found to have an accuracy rate of 96.7% in a study published in the Journal of Medical Systems. This technology has the potential to improve access to COVID-19 testing in remote and underserved areas.
These success stories and case studies demonstrate the potential of smartphone-based computer vision technology in improving healthcare outcomes, particularly in low-resource settings where access to trained medical professionals and laboratory tests may be limited.
The Future Implications and Potential for Smartphone-based Computer Vision
Smartphone-based computer vision technology has the potential to revolutionize healthcare diagnostics by enabling accurate and efficient interpretation of rapid diagnostic tests. As technology continues to advance, the implications and potential for this innovative approach are vast and promising.
One of the emerging trends in smartphone-based computer vision is the integration of machine learning techniques. By enabling algorithms to learn from large amounts of data, machine learning can improve the accuracy and efficiency of RDT interpretation. Additionally, advancements in image processing algorithms can further enhance the reliability of smartphone-based computer vision technology.
Beyond RDT interpretation, smartphone-based computer vision technology offers potential applications in remote patient monitoring and telemedicine. By enabling healthcare professionals to remotely monitor and analyze patient data, this technology can improve access to medical care and reduce healthcare costs.
Despite the challenges and limitations discussed in previous sections, ongoing research is focused on addressing these issues and further advancing smartphone-based computer vision technology. As a result, the potential for this innovative approach to healthcare diagnostics is vast and exciting.
Conclusion
In conclusion, smartphone-based computer vision technology has the potential to revolutionize the way we approach diagnostic testing in healthcare. By leveraging machine learning algorithms and image processing techniques, this technology can enhance the accuracy and efficiency of RDT interpretation, leading to better patient outcomes and more effective healthcare management.
While there are still challenges and limitations to be addressed, recent innovations and success stories demonstrate the immense potential of smartphone-based computer vision in healthcare. As the technology continues to evolve and improve, we can expect to see further advancements in RDT interpretation and other areas of medical diagnostics.
Ultimately, the future looks bright for this innovative approach to healthcare diagnostics. By integrating smartphone-based computer vision technology into our healthcare systems, we can improve the quality and accessibility of medical care for all.