Unlocking Rapid Diagnostic Test's with Advanced Machine Learning Techniques for Smartphones
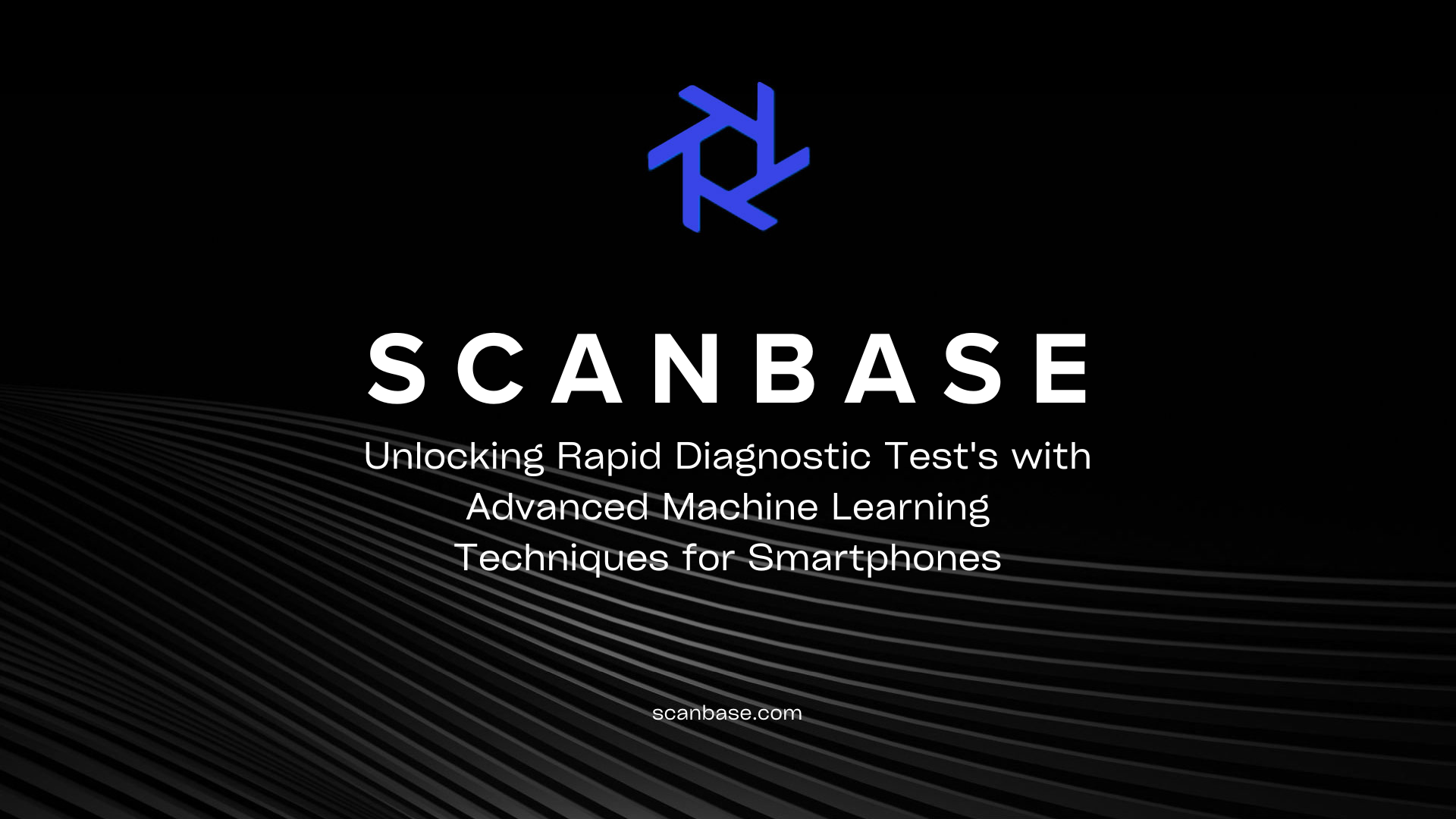
In today's rapidly changing world, new technologies are being developed every day to improve healthcare services. One of the most exciting advancements is the use of advanced machine learning techniques for smartphone interpretation of rapid diagnostic tests (RDT's). With the help of AI algorithms and deep learning approaches, smartphones can now accurately interpret diagnostic tests, providing instant results and faster decision-making for healthcare professionals.
This article will explore the potential benefits of using advanced machine learning techniques for smartphone RDT interpretation. We will discuss the various machine learning techniques available, including AI algorithms and deep learning approaches, and how they can revolutionize the accuracy and speed of diagnostic tests. We will also explore the challenges associated with implementing these technologies and potential future directions in smartphone RDT interpretation.
Key Takeaways:
- Advanced machine learning techniques are being used to interpret rapid diagnostic tests (RDT's) on smartphones.
- AI algorithms and deep learning approaches can improve the accuracy and speed of diagnostic tests on smartphones.
- The use of AI-powered RDT interpretation can bring accurate and reliable diagnostic capabilities to remote and resource-limited settings.
- The challenges associated with implementing advanced machine learning techniques for smartphone RDT interpretation can be overcome with proper data privacy, algorithm accuracy, and bias considerations.
The Power of Advanced Machine Learning Techniques for Smartphone Interpretation of RDT's
Advanced machine learning techniques are rapidly transforming the field of diagnostic testing, with smartphones emerging as a powerful platform for interpreting rapid diagnostic tests (RDT's). With the ability to process vast amounts of data and analyze patterns at lightning speed, smartphones are poised to revolutionize the accuracy, speed, and convenience of diagnostic testing.
Machine learning techniques can help smartphones accurately interpret RDT's by identifying subtle patterns and correlations that may be missed by human analysis. AI algorithms and deep learning approaches enable smartphones to learn from previous test results and continuously improve their accuracy over time.
Smartphone-based interpretation of RDT's can dramatically reduce the time and cost associated with traditional diagnostic testing. Real-time analysis on smartphones enables healthcare professionals to quickly make informed decisions about patient care, leading to improved outcomes and better patient experiences.
The Role of AI Algorithms in Smartphone RDT Interpretation
AI algorithms are a critical component of smartphone-based RDT interpretation. These algorithms are trained and optimized to accurately interpret test results, even in complex or ambiguous cases. By leveraging the power of deep learning, AI algorithms can quickly identify patterns and correlations that may be missed by human analysis.
One of the key advantages of AI-powered RDT interpretation is its ability to continuously learn and improve over time. By analyzing large datasets of previous test results, AI algorithms can refine their interpretation capabilities and enhance diagnostic accuracy.
The Power of Deep Learning for RDT Interpretation
Deep learning is another cutting-edge technology that is transforming smartphone diagnostics for RDT's. By analyzing complex patterns in test results, deep neural networks enable smartphones to make accurate and reliable diagnoses. These networks can quickly identify even subtle variations in test results and provide real-time feedback to healthcare professionals.
Deep learning algorithms are particularly useful in cases where multiple RDT's are being performed simultaneously. By analyzing the results of multiple tests and their interrelationships, deep learning networks can provide a more comprehensive and accurate assessment of a patient's condition.
As the field of machine learning continues to evolve, the potential applications for smartphone-based RDT interpretation are virtually limitless. From improving point-of-care testing in remote or resource-limited settings to accelerating the pace of medical research, advanced machine learning techniques are poised to transform the future of diagnostic testing.
The Power of Advanced Machine Learning Techniques for Smartphone Interpretation of RDT's
Advanced machine learning techniques have the potential to unlock rapid diagnostic tests (RDT's) with the help of smartphones. This is particularly relevant in remote and resource-limited settings, where access to traditional diagnostic systems may be limited.
Smartphone interpretation of RDT's is made possible by cutting-edge machine learning techniques, such as AI algorithms and deep learning approaches. These techniques empower smartphones to analyze and accurately interpret test results in real-time, revolutionizing the speed and accuracy of diagnostic testing.
Through the use of AI-powered RDT interpretation, healthcare professionals can make faster decisions and provide reliable diagnoses, even in areas with limited access to medical equipment and expertise.
Furthermore, deep learning techniques enable smartphones to analyze complex patterns in test results, leading to more accurate interpretations. With advanced machine learning techniques, smartphones are capable of providing accurate and reliable diagnostic capabilities in point-of-care testing settings.
While there are challenges to implementing advanced machine learning techniques for smartphone interpretation of RDT's, such as data privacy and algorithm accuracy, these hurdles can be overcome by leveraging cutting-edge technology and thoughtful planning.
The future of smartphone diagnostic testing looks promising, with emerging technologies and trends expected to further enhance the capabilities of smartphones in interpreting RDT's. Overall, the transformative potential of advanced machine learning techniques for smartphone interpretation of RDT's is an exciting development in the field of diagnostic medicine.
AI Algorithms for RDT's: Unleashing the Power of Machine Learning
When it comes to interpreting rapid diagnostic tests (RDT's) on smartphones, advanced machine learning techniques are critical to achieving accuracy and reliability. One of the most prominent techniques is AI algorithms, which can be specifically trained to analyze and interpret RDT results.
AI algorithms for RDT interpretation work by analyzing large datasets of previous test results to identify patterns and associations between different factors. Based on this data, the algorithms can accurately predict the results of future tests in a matter of seconds.
These algorithms are powered by deep learning approaches, which enable smartphones to "learn" from previous test results and continuously improve their accuracy over time. As more and more data is fed into the system, the algorithms become even more sophisticated and reliable.
One of the most significant advantages of AI-powered RDT interpretation is its speed. By providing instant results, technicians and healthcare professionals can make faster decisions about patient care and treatment. Additionally, this can save time and reduce costs associated with traditional laboratory-based testing.
However, utilizing AI algorithms for RDT interpretation also requires addressing certain challenges. One of the key challenges is ensuring the accuracy of the algorithms themselves. Inaccurate algorithms can lead to incorrect diagnoses and compromised patient care. Therefore, it's critical to continuously monitor and improve the algorithms to ensure their accuracy and reliability.
Moreover, it's essential to address potential biases in the data used to train the algorithms. Biases can occur when the training data is not representative of the population being tested, leading to inaccurate results and diagnoses. Therefore, it's important to use diverse and representative datasets to train the algorithms and minimize potential biases.
Overall, AI algorithms for RDT interpretation are a powerful tool in the field of smartphone-based diagnostics. As technology continues to evolve, these algorithms will undoubtedly become even more sophisticated and reliable, further advancing the capabilities of smartphones in diagnostic testing.
Deep Learning for RDT's: Enhancing Smartphone Diagnostics
The use of deep learning in smartphone diagnostics has revolutionized the accuracy of RDT interpretation. Deep neural networks can analyze complex patterns in test results and provide more reliable diagnoses, giving healthcare professionals better tools to provide high-quality care.
Deep learning algorithms can help overcome the challenges associated with interpreting RDT's on smartphones, including detecting faint lines and small variations in color. These algorithms can learn to recognize the subtle differences between healthy and diseased samples and provide more accurate results.
The benefits of deep learning for smartphone RDT interpretation are clear. It has the potential to improve the accuracy of diagnoses and reduce the need for additional testing, saving time and resources for both patients and healthcare providers.
Moreover, the use of deep learning has enabled the development of more advanced diagnostic tests that can detect multiple diseases using a single test. This is particularly useful in resource-limited settings where access to multiple diagnostic tests may be difficult.
Overall, the integration of deep learning into smartphone diagnostics is a significant advancement in the field of point-of-care testing, and it has the potential to improve patient outcomes and revolutionize healthcare delivery.
AI-Powered RDT Interpretation: Advancing Point-of-Care Testing
The use of advanced machine learning techniques for smartphone interpretation of rapid diagnostic tests (RDT's) has the potential to revolutionize point-of-care testing. With the power of AI algorithms and deep learning approaches, smartphones can provide accurate and reliable diagnostic capabilities even in resource-limited settings.
The benefits of AI-powered RDT interpretation are clear. Real-time analysis on smartphones allows for instant results and faster decision-making for healthcare professionals. This can lead to faster diagnoses, earlier interventions, and ultimately better patient outcomes.
One of the key advantages of smartphone-based interpretation is its portability. Compared to traditional laboratory-based testing, smartphones are much more accessible, especially in remote and rural areas. This can be particularly important for diseases such as malaria or HIV, where timely diagnosis is critical for effective treatment.
The use of AI algorithms for RDT interpretation is also highly efficient. These algorithms are trained and optimized to accurately interpret test results, and can continuously learn and improve over time. This means that as more data is collected and analyzed, the accuracy of smartphone-based diagnosis will only continue to improve.
Deep learning approaches are also enhancing smartphone diagnostics for RDT's. By analyzing complex patterns in test results, deep neural networks can provide more accurate interpretations and reliable diagnoses. This is especially important for diseases with a wide range of symptoms and manifestations, such as tuberculosis or dengue fever.
Overall, the advances in advanced machine learning techniques for smartphone interpretation of RDT's are transforming point-of-care testing. By overcoming the challenges of data privacy, algorithm accuracy, and potential biases, we are unlocking the full potential of these technologies to improve global health outcomes.
Advanced ML for Smartphone RDT Interpretation: Overcoming Challenges.
As with any emerging technology, there are challenges to implementing advanced machine learning techniques for smartphone interpretation of RDT's. However, these hurdles can be overcome by strategic planning and careful considerations.
One of the main challenges is ensuring the accuracy of the AI algorithms used for RDT interpretation. Since these algorithms are trained on datasets, the quality and quantity of data used to train them can impact the accuracy of the results. It is essential to ensure that the datasets are diverse and representative of the population being tested.
Another challenge is data privacy. With the use of smartphones for RDT interpretation, there is both the potential for data breaches and the concern of unintentionally sharing personal information. It is crucial to implement robust security protocols and adhere to privacy regulations to address these concerns.
Potential biases in the design and implementation of machine learning models can also be a challenge. In the context of RDT interpretation, this could manifest in inaccurate diagnoses for specific subpopulations. It is necessary to consider diversity and inclusivity in the design and testing of algorithms to avoid these biases.
Despite these challenges, the potential benefits of advanced machine learning techniques for smartphone interpretation of RDT's make the effort well worth it. By confronting these challenges head-on, healthcare providers can improve diagnostic speed and accuracy while expanding access to reliable diagnostics for underserved communities.
Future Directions in Smartphone RDT Interpretation
The field of smartphone-based interpretation of RDT's is rapidly evolving, with new technologies and approaches constantly emerging. Here are some potential future directions in this area:
Increased Integration of AI Algorithms
As AI algorithms become more sophisticated, they have the potential to further enhance the accuracy and reliability of smartphone-based interpretation of RDT's. As these algorithms continue to learn and adapt to new data, smartphone diagnostics may become even more effective and efficient.
Wearable Technology for Remote Monitoring
As wearable technology becomes more widespread, there is potential to use it for remote monitoring of diagnostic results. This could allow healthcare professionals to monitor patients' health in real-time and provide rapid interventions when necessary.
Integration with Telemedicine
Telemedicine has the potential to overcome barriers to healthcare access and improve patient outcomes. By integrating smartphone-based interpretation of RDT's with telemedicine, healthcare professionals can diagnose and treat patients remotely, improving access to care and reducing healthcare costs.
Improved Privacy and Security
As with any technology that involves sensitive patient data, ensuring privacy and security is paramount. As smartphone-based interpretation of RDT's becomes more widespread, there will be a need for improved privacy and security measures to protect patient data.
Continued Research and Development
The field of smartphone-based interpretation of RDT's is still relatively new, and there is much to be discovered and developed. Ongoing research and development will be necessary to further advance the capabilities of this technology and bring it to more patients around the world.
Conclusion
As we have seen, advanced machine learning techniques have the potential to revolutionize the way we interpret rapid diagnostic tests using smartphones. These cutting-edge technologies, including AI algorithms and deep learning approaches, can enhance the speed and accuracy of diagnostic testing, allowing for real-time analysis and faster decision-making for healthcare professionals.
By leveraging the power of smartphones, we can bring reliable and accurate diagnostic capabilities to remote and resource-limited settings, advancing the field of point-of-care testing. However, implementing these advanced machine learning techniques is not without challenges, such as ensuring data privacy and algorithm accuracy while avoiding potential biases.
Looking to the future, we can expect the continued evolution of smartphone RDT interpretation, with emerging technologies and trends further enhancing the capabilities of these devices in diagnostic testing. With the potential benefits in mind, it's clear that advanced machine learning techniques for smartphone interpretation of RDT's represent an exciting and transformative field.