Unlocking Explainable AI for Clear Rapid Diagnostic Test Interpretation on Smartphones
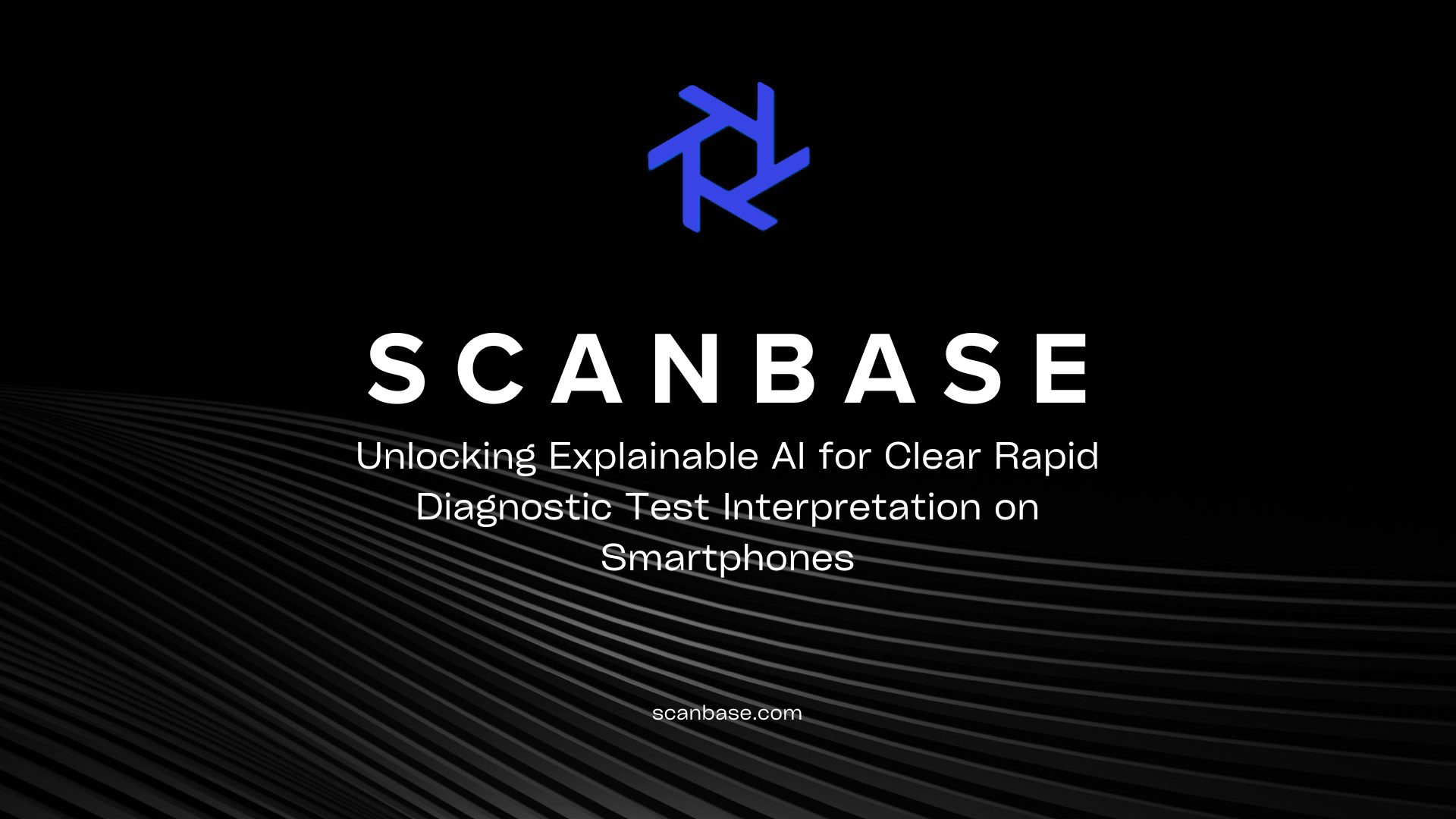
In recent years, the field of healthcare has greatly benefited from advances in artificial intelligence (AI). One of the areas where AI has shown great potential is in the interpretation of rapid diagnostic tests (RDTs), which can provide valuable insights into a patient’s health status. However, the use of AI in RDT interpretation raises questions regarding transparency and interpretability.
Enter explainable AI. By exploring explainable AI for transparent interpretation of RDTs on smartphones, healthcare professionals and users can trust the results they receive and make informed decisions about their health.
Key Takeaways
- Exploring explainable AI can provide transparent interpretation of RDTs on smartphones
- A transparent interpretation is necessary for accurate and trustworthy diagnostics
- Explainable AI ensures healthcare professionals and users can understand the decision-making process of AI models
The Significance of AI Transparency and Interpretability in RDTs
As AI becomes increasingly integrated into healthcare services, ensuring transparency and interpretability of AI models is essential for building trust and ensuring accurate diagnoses. Explainable artificial intelligence (XAI) and interpretable machine learning (IML) are two techniques that can enhance AI transparency and interpretability.
XAI algorithms provide clear explanations for why an AI model made a specific decision, while IML models are designed to be easily understandable by humans. By incorporating these techniques into AI models for rapid diagnostic tests (RDTs), healthcare professionals can gain deeper insights into the decision-making process of these models and increase their trust in the results.
Transparency and interpretability are crucial in the context of RDTs, where accurate diagnoses are essential for effective treatment. With the potential to democratize healthcare access and improve diagnostic accuracy, the significance of AI transparency and interpretability in RDTs cannot be overstated.
Leveraging Explainable AI for Transparent RDT Interpretation
Rapid diagnostic tests (RDTs) are an effective tool for quick and accurate diagnosis, but their interpretation can be challenging, often requiring trained professionals. With the rise of smartphone technology and artificial intelligence (AI), there is an opportunity to democratize access to diagnostic services by leveraging smartphone AI for RDT interpretation. However, to ensure trustworthy interpretation, it is important to incorporate transparent AI algorithms.
Explainable AI can play a critical role in achieving transparent RDT interpretation on smartphones. By designing AI algorithms that provide clear explanations for their diagnostic decisions, healthcare professionals and patients can better understand and trust the results. Transparent AI algorithms enable users to see why a particular diagnosis was made and how the algorithm arrived at that decision.
Through the use of interpretable machine learning techniques, explainable AI can provide insights into the decision-making process of AI models, allowing for greater transparency and accountability. Smartphone AI can leverage these transparent algorithms to provide accessible and convenient RDT interpretation for users on the go.
Transparent AI algorithms can also improve the interpretability of AI models, making the diagnostic process more understandable and accountable. By incorporating explainability in AI systems, users can easily comprehend the diagnostic results they receive and make informed decisions regarding their health.
The Benefits of Transparent AI Algorithms for RDT Interpretation on Smartphones
The benefits of transparent AI algorithms in RDT interpretation on smartphones are numerous. By providing a clear and understandable diagnostic process, users can trust the diagnoses they receive and make informed decisions regarding their health. Moreover, transparent AI algorithms enable healthcare professionals to better understand the decision-making process of AI models, leading to improved diagnostic accuracy and increased confidence in RDT interpretation.
In the next section, we will delve deeper into the role of transparent AI algorithms in RDT interpretation, exploring how they improve the interpretability of AI models in the context of rapid diagnostics.
The Role of Transparent AI Algorithms in RDT Interpretation
Transparent AI algorithms play a critical role in improving the interpretability of AI models, making the diagnostic process more understandable and accountable. It is essential to incorporate explainability in AI systems to provide clear and reliable diagnostic results.
Explainable artificial intelligence and interpretable machine learning techniques offer insights into the decision-making process of AI models, enabling healthcare professionals and users to understand and trust the results. These algorithms show how the AI arrived at a decision, providing transparency that can help identify potential biases or errors in the diagnostic process.
With transparent AI algorithms, healthcare professionals and users can understand how and why a particular diagnostic decision was made. This understanding helps to build trust in the accuracy and reliability of the diagnostic results.
Benefits of Transparent AI Algorithms |
---|
Improved trust in diagnostic results |
Reduction of potential biases or errors in the diagnostic process |
Greater interpretability of AI models |
Identification of discrepancies in the decision-making process |
It is crucial to design AI systems with interpretable machine learning techniques to ensure that users can easily understand and trust the diagnostic results they receive. This approach will help to maximize the potential of AI and democratize access to accurate diagnostic services.
Exploring the Potential of Smartphones in RDT Interpretation
Smartphones have become an integral part of our lives, and their potential in transforming healthcare cannot be ignored. With the advancements in smartphone technologies and algorithm development, they have emerged as a promising platform for RDT interpretation using AI.
The use of smartphones in RDT interpretation has several advantages, including accessibility, convenience, and cost-effectiveness. With the increasing availability of smartphones, users can receive quick and accurate diagnostic results from the comfort of their homes, without the need for specialized equipment or medical expertise.
Smartphones can also enable the deployment of AI algorithms for RDT interpretation, thanks to the high processing power of modern smartphones. These algorithms can provide accurate and rapid results, enabling healthcare professionals to make informed decisions about treatment and care.
The use of smartphone AI for RDT interpretation has the potential to democratize access to healthcare services, particularly in remote areas or areas with limited access to medical facilities. With the widespread availability of smartphones and the ease of use of AI algorithms, users can receive accurate and trustworthy diagnostic results, irrespective of their location or socio-economic status.
In conclusion, smartphones have immense potential in RDT interpretation using AI, and their use can transform healthcare delivery and accessibility. The development of robust AI algorithms and the integration of interpretable machine learning techniques can enable transparent and trustworthy diagnostic results for users, improving healthcare outcomes and patient care.
Implementing Explainable AI for User-Friendly RDT Interpretation
As discussed in the previous sections, explainable AI plays a crucial role in transparent RDT interpretation on smartphones. However, designing interpretability into AI systems is no easy feat, and requires a thoughtful approach to model development and implementation.
Interpretable machine learning techniques are central to the development of user-friendly RDT interpretation algorithms. These techniques incorporate features such as feature importance, decision boundaries, and counterfactual explanations to provide clear and concise explanations for AI model decisions. This information is critical to building trust in the algorithm and ensuring that users understand the rationale behind the diagnostic decision.
Moreover, it is essential to design RDT interpretation algorithms with users in mind. This means that the algorithm should be intuitive, accessible, and user-friendly. The AI system should be able to interpret RDT results with easy-to-understand language, providing users with actionable insights that they can use to make decisions about their health.
Further, the use of smartphones as a platform for RDT interpretation is particularly exciting given the increasing ubiquity of smartphones across the globe. However, the design of AI systems for smartphones must take into account the limitations of the platform, such as limited processing power, storage capacity, and screen size.
In conclusion, implementing explainable AI for user-friendly RDT interpretation is pivotal to the success of RDTs on smartphones. Interpretable machine learning techniques are at the forefront of making such interpretation possible, and designing AI systems with users in mind is essential to ensure that RDT interpretation is accessible to all. By exploring explainable AI, RDTs on smartphones have the potential to revolutionize healthcare access and treatment.
Addressing Challenges in Transparent RDT Interpretation on Smartphones
While the use of smartphones for transparent RDT interpretation holds great promise, there are some challenges that need to be addressed to ensure accurate and reliable results.
One of the main challenges is the limited processing power of smartphones, which can impact the speed and accuracy of AI algorithms. To address this issue, AI models need to be optimized for mobile devices, taking into account factors such as battery life and available memory.
Data privacy concerns also need to be addressed. With sensitive health information being transmitted and stored on smartphones, it is crucial that appropriate security measures are in place to protect user privacy.
Additionally, the need for robust and adaptable algorithms is paramount. Various types of RDTs exist, and AI models must be capable of interpreting results from a wide range of tests accurately. Furthermore, these algorithms need to be designed to explain their decision-making process in a clear and meaningful way to users.
Overall, addressing these challenges is critical to unlocking the full potential of transparent RDT interpretation on smartphones, ensuring accessible and accurate diagnostic services for all.
Advancements and Future Directions in Explainable RDT Interpretation
As the field of AI continues to evolve, so do the advancements and future directions in explainable RDT interpretation on smartphones. Exploring explainable AI and interpretable machine learning techniques is crucial in ensuring accurate and transparent diagnostic results.
Ongoing research efforts are focused on optimizing the processing power of smartphones to handle the demands of transparent AI algorithms, while also addressing data privacy concerns. The development of robust algorithms that can handle different types of RDTs and generate interpretable explanations for their diagnostic decisions is also a key area of focus.
The potential of smartphones in democratizing access to accurate diagnostic services is immense. With explainable AI algorithms, users can receive quick and accurate diagnoses, even in resource-limited settings. It is envisioned that in the near future, smartphones will be equipped with advanced AI systems that provide real-time interpretation of RDTs, helping to improve healthcare outcomes globally.
Overall, the future of explainable RDT interpretation on smartphones is bright. With the constant evolution of AI technologies and innovative research, we can continue to unlock the potential of transparent AI systems for clear and trustworthy RDT diagnoses.
Conclusion
Unlocking explainable AI for clear RDT interpretation on smartphones is a critical step towards making diagnostics more accessible and accurate for all. The significance of AI transparency and interpretability in RDTs cannot be overstated, as it enables healthcare professionals and users to trust and understand the diagnostic results they receive.
Leveraging explainable AI for transparent RDT interpretation has enormous potential, and smartphones can serve as a vital platform for delivering these services. However, challenges such as limited processing power and data privacy concerns need to be addressed to ensure that these services are viable and sustainable.
Looking to the future
Despite these challenges, advancements in the field of explainable RDT interpretation on smartphones are promising. Ongoing research and development efforts are continuously improving AI transparency and interpretability, paving the way for accurate and understandable RDT diagnoses for all.
As we continue to explore the potential of explainable AI, it is crucial to design AI systems with interpretable machine learning techniques that prioritize the needs of users and healthcare professionals. By doing so, we can democratize access to accurate diagnostic services and ensure that everyone receives the care they need.
With the potential to usher in a new era of accessible, transparent, and accountable diagnostics, the future of explainable RDT interpretation is exciting and full of possibilities.