Smartphone-based Deep Learning for Rapid Pathogen Detection
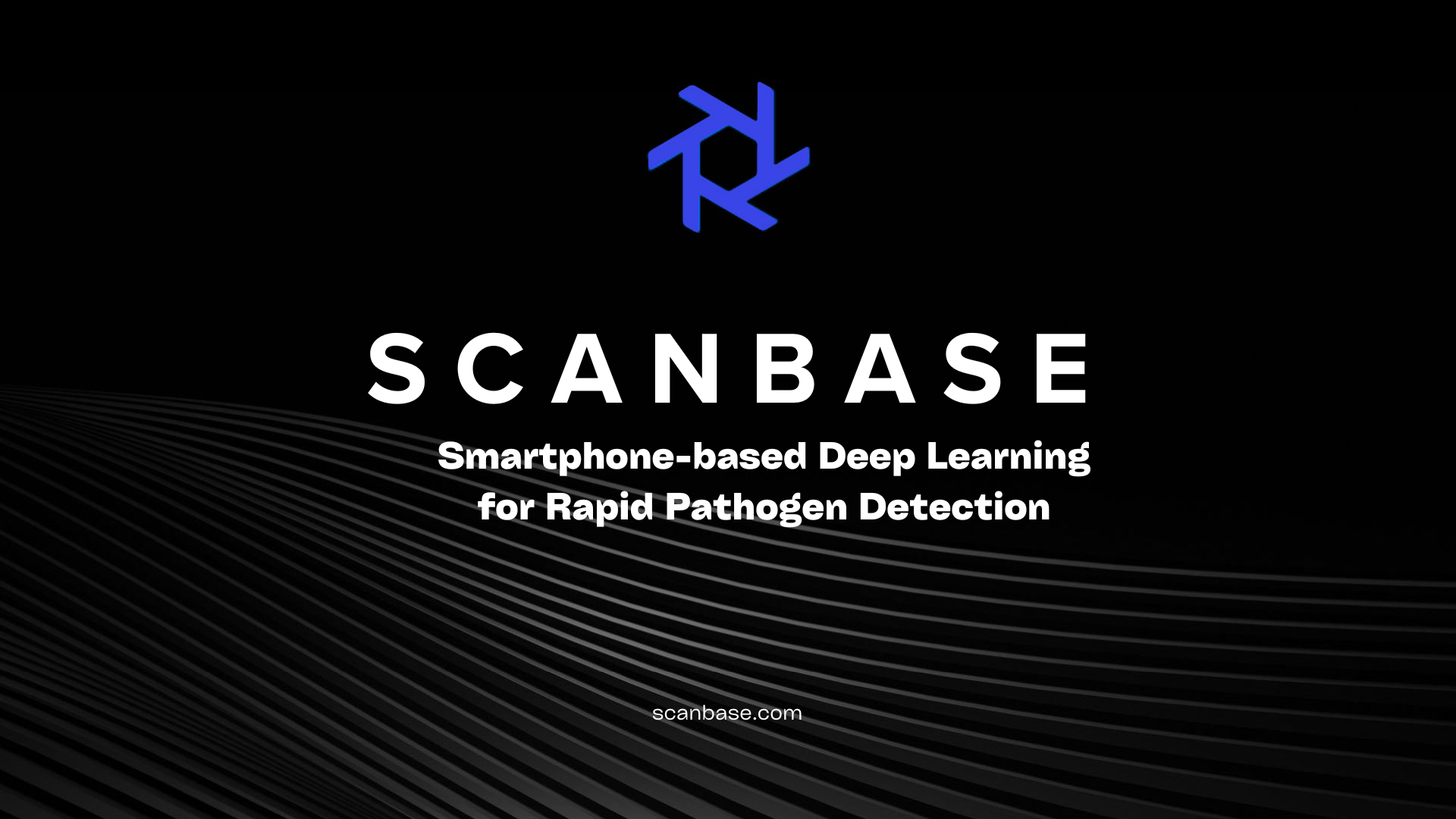
In recent years, the development of smartphone-based deep learning approaches for rapid pathogen detection has revolutionized the field of diagnostics. With its accuracy and speed, this technology has the potential to significantly improve disease control and public health surveillance.
By leveraging the computational power of smartphones, deep learning algorithms can quickly analyze large amounts of data and extract patterns to identify specific pathogens. This allows for faster and more accurate detection, enabling prompt decision-making for effective response.
Key Takeaways:
- Smartphone-based deep learning approaches offer a transformative potential for rapid pathogen detection.
- These approaches leverage the computational power and capabilities of smartphones to quickly analyze large amounts of data.
- Deep learning algorithms can identify specific pathogens, allowing for faster and more accurate detection.
The Importance of Rapid Pathogen Detection
With the increasing threat of infectious diseases, rapid pathogen detection has become a critical aspect of healthcare, food safety, and environmental monitoring. Delayed detection can lead to severe consequences, including the spread of disease, contamination of food or water sources, and environmental degradation. Early identification of pathogens is essential for prompt decision-making and effective response.
According to the Centers for Disease Control and Prevention, approximately 1 in 6 Americans fall ill due to foodborne pathogens each year, leading to an estimated 128,000 hospitalizations and 3,000 deaths. Prompt detection can prevent further spread of disease and save lives.
Understanding Deep Learning
Deep learning is a subfield of machine learning that involves training artificial neural networks to analyze and learn from large sets of data. These networks are designed to simulate the behavior of the human brain, allowing them to identify patterns and make predictions with a high degree of accuracy.
Deep learning algorithms can be used to analyze a wide range of complex data, including images, voice recordings, and text. In the field of pathogen detection, deep learning can be used to analyze images of pathogens and identify specific characteristics that distinguish them from other microorganisms.
The Role of Smartphones in Detection
Smartphones have become ubiquitous in daily life and are increasingly being utilized in various fields, including diagnostics. In the context of pathogen detection, smartphones offer several advantages over traditional laboratory-based methods.
Equipped with high-resolution cameras, sensors, and computing power, smartphones can function as portable laboratories, allowing for rapid analysis of pathogen samples. This capability is especially significant in remote or resource-limited settings, where access to traditional laboratory facilities may be limited or non-existent.
Moreover, the use of smartphones in pathogen detection can enable real-time analysis and prompt decision-making, which is essential for effective disease control and response. With the availability of wireless communication and cloud-based data storage, results can be shared quickly and efficiently, facilitating collaboration among healthcare professionals and public health officials.
Advantages of Smartphone-based Deep Learning
The utilization of smartphone-based deep learning approaches for pathogen detection provides several advantages over traditional diagnostic methods. One of the most significant advantages is affordability. Smartphones are widespread, and their cost is relatively low, making them an accessible tool for resource-restricted regions.
With the integration of deep learning approaches, smartphones can perform rapid detection and provide real-time analysis, enabling prompt decision-making for effective response. The accuracy and speed of this technology can significantly reduce the time and resources needed for pathogen identification, potentially saving lives in emergency situations.
In addition to affordability and accessibility, smartphone-based detection is non-invasive and has a less complex sample preparation process compared to other traditional diagnostic methods. The use of smartphones also reduces the need for expensive and bulky laboratory equipment, increasing portability and allowing for on-site testing in various settings.
Smartphone-based deep learning approaches for pathogen detection have the potential to revolutionize diagnostics, particularly in low-resource settings where access to traditional laboratory facilities may be limited. It also holds promise for achieving rapid and accurate detection of emerging infectious diseases such as the current COVID-19 pandemic.
Pathogen Identification with Smartphone-based Deep Learning
Smartphone-based deep learning approaches offer a promising solution for rapid pathogen identification. Pathogens can be identified by their unique characteristics, such as shape, color, and texture, which can be recognized by deep learning models. These models are trained on large datasets of pathogen images, allowing them to accurately identify pathogens from new images.
The process of pathogen identification using smartphone-based deep learning involves capturing an image of the suspected pathogen with the smartphone camera. The image is then analyzed by the deep learning model, which matches the image with known pathogen characteristics to identify the pathogen.
Advantages of Pathogen Identification with Smartphone-based Deep Learning |
---|
Accuracy: Deep learning models have shown high accuracy in detecting and identifying pathogens. |
Speed: The process of pathogen identification using smartphone-based deep learning is rapid, providing results in minutes. |
Portability: Smartphones are portable and can be taken to the location of suspected pathogen outbreaks, enabling prompt response. |
The ability to accurately and rapidly identify pathogens using smartphone-based deep learning approaches has significant implications for disease control and public health. It can enable early detection and response to pathogen outbreaks, preventing the spread of diseases and saving lives.
Furthermore, the portability and affordability of smartphone-based deep learning approaches make it accessible to a wider range of people, including those in low-resource settings. As the technology continues to improve and become more widespread, it has the potential to revolutionize diagnostics and disease control globally.
Overcoming Challenges in Smartphone-based Detection
Despite the potential benefits of smartphone-based detection, there are still some challenges that must be addressed. One of the main challenges is the limited computational resources of smartphones, which can affect the accuracy and speed of pathogen detection.
In addition, image quality can vary depending on factors such as lighting and camera resolution, which can further impact the performance of deep learning algorithms. To address these issues, researchers are exploring various approaches, such as optimizing algorithms for smartphone computing and developing standardized protocols for image acquisition.
Another challenge is the need for reliable data sets for training deep learning models. This is especially important for pathogen identification, as the models must be trained to recognize specific features of each pathogen. To ensure accurate detection, researchers are working to create diverse and representative data sets that can capture the full range of pathogen variability.
Despite these challenges, the potential benefits of smartphone-based detection make it a promising technology for pathogen identification. Ongoing efforts to improve the reliability and accuracy of this approach will likely lead to increased adoption and utilization in various fields.
Real-world Applications of Smartphone-based Deep Learning
Smartphone-based deep learning approaches have already demonstrated tremendous potential for rapid pathogen detection in real-world scenarios. Here are some examples of how this technology is being utilized:
Field | Application |
---|---|
Healthcare | Smartphone-based detection systems have been developed for various infectious diseases, such as HIV and tuberculosis. These systems can accurately diagnose diseases in resource-limited settings, where traditional laboratory-based methods may not be feasible. |
Public Health Surveillance | Smartphone cameras have been used to capture images of food samples taken from markets and restaurants. Deep learning algorithms analyze these images to detect potential pathogens, making it a valuable tool for monitoring food safety. |
Emergency Response | Smartphone-based detection systems can facilitate rapid response to disease outbreaks, natural disasters, and bioterrorism incidents. It can quickly identify the infectious agents and prevent further spread of the disease. |
These real-world applications demonstrate the versatility and efficacy of smartphone-based deep learning approaches for rapid pathogen detection. As technology advances, these systems will become even more accessible and widely adopted, with the potential to transform global health and disease control.
Future Directions and Potential Impact
Smartphone-based deep learning approaches for rapid pathogen detection offer immense potential for revolutionizing diagnostics. While this technology is still in its infancy, ongoing research and development efforts are focused on refining and improving its accuracy and reliability.
In the future, one potential direction for smartphone-based deep learning approaches is integration with other diagnostic tools, such as molecular assays and nucleic acid amplification tests. This could enable a more comprehensive and definitive diagnosis, particularly in cases where multiple pathogens are suspected. Additionally, advancements in machine learning algorithms and computational power could further enhance the speed and accuracy of pathogen identification.
Another potential impact of smartphone-based deep learning approaches is in their application to global disease control efforts. With its affordability and accessibility, this technology could enable rapid detection and response to outbreaks in resource-limited settings, where traditional diagnostic tools may not be available. This could have a significant impact on reducing the spread of infectious diseases and improving public health outcomes.
Overall, the potential impact of smartphone-based deep learning approaches for rapid pathogen detection is vast. As research and development continue, it is likely that this technology will become an increasingly important tool in the fight against infectious diseases.
Conclusion
Smartphone-based deep learning approaches for rapid pathogen detection have the potential to revolutionize diagnostics with their accuracy and speed. The importance of rapid pathogen detection in healthcare, food safety, and environmental monitoring cannot be overstated, and the benefits of early identification are significant.
Deep learning algorithms have shown tremendous potential in analyzing large amounts of data and extracting patterns, making them ideal for pathogen detection. Coupled with the computing power and high-resolution cameras of smartphones, these algorithms can be used to perform rapid analysis in real-time, enabling prompt decision-making for effective response.
While challenges associated with smartphone-based detection exist, such as limited computational resources and potential variation in image quality, ongoing efforts are being made to overcome these obstacles and improve the reliability of this technology.
The real-world applications of smartphone-based deep learning approaches for rapid pathogen detection are vast and widespread. From healthcare settings to public health surveillance and emergency response scenarios, this technology has shown promise in delivering accurate and timely results.
Looking ahead, the future of smartphone-based deep learning approaches for pathogen detection looks bright. Potential advancements in technology and integration with other diagnostic tools could further improve the accuracy and speed of detection. Ultimately, the impact of this technology on global health and disease control could be transformative.
In Conclusion:
Smartphone-based deep learning approaches for rapid pathogen detection have the potential to transform diagnostics, with their accuracy and speed. In healthcare, food safety, and environmental monitoring, early identification of pathogens is critical. The computing power, high-resolution cameras, and sensors of smartphones equip them for portable laboratory analysis. Overcoming challenges will make this solution affordable, accessible, and reliable for real-time analysis. The application of this technology is vast and varied, from public health surveillance to emergency response scenarios. With further research and technological developments, the impact of smartphone-based deep learning approaches for pathogen detection could be transformative for global health and disease control.