Smartphone-Based Computer Vision for Rapid Antimicrobial Susceptibility Testing
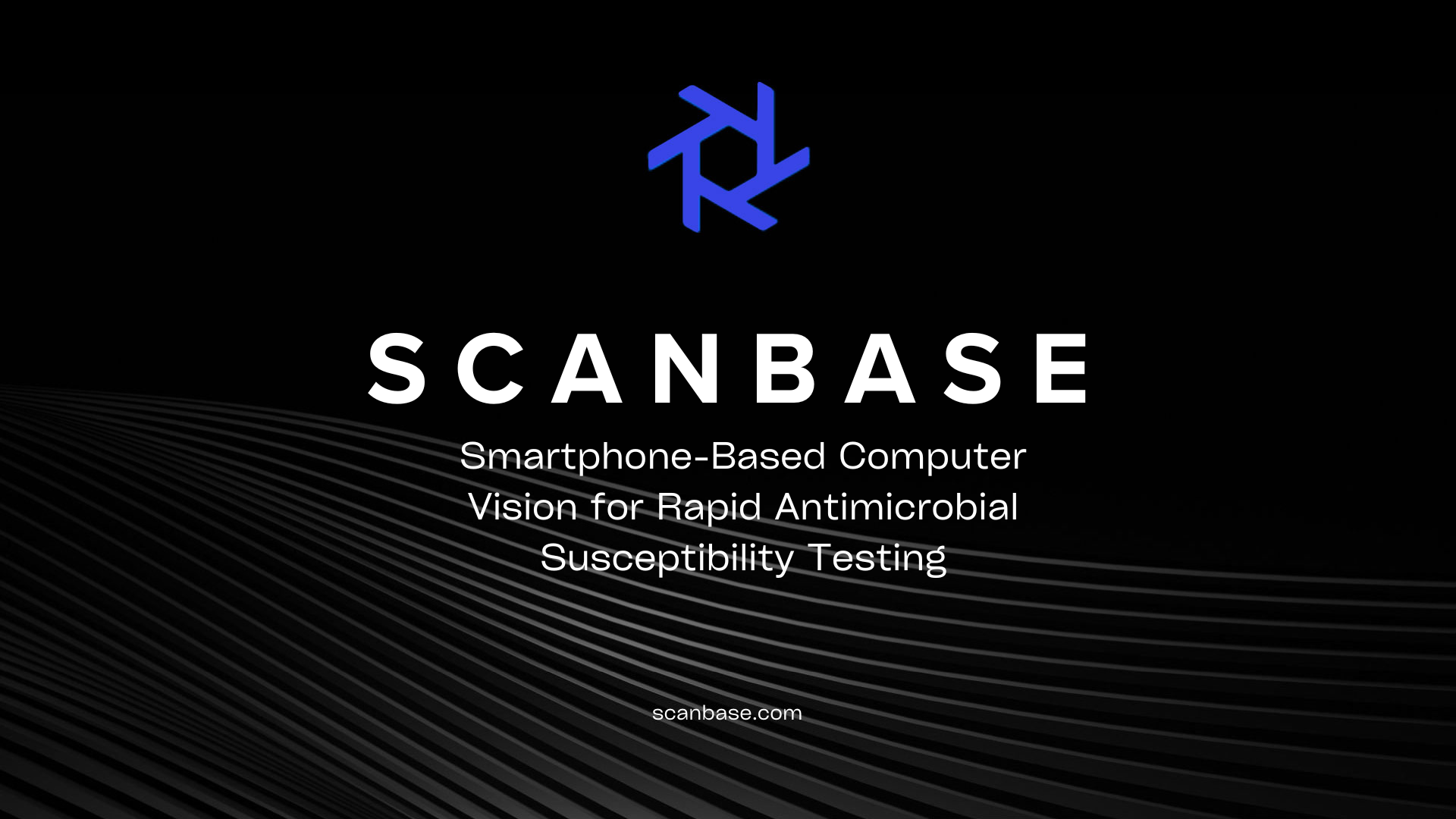
The rapid spread and emergence of drug-resistant pathogens have raised concerns worldwide. Antimicrobial resistance undermines the effectiveness of antibiotics, leading to increased morbidity and mortality rates. In this context, rapid antimicrobial susceptibility testing is crucial in guiding appropriate antibiotic treatment, reducing healthcare costs, and improving patient outcomes.
However, traditional culture-based methods for antimicrobial susceptibility testing are time-consuming, taking up to several days to generate results. This delay can lead to inappropriate antibiotic prescribing, exacerbating the problem of antibiotic resistance.
Smartphone-based computer vision for rapid antimicrobial susceptibility testing represents an innovative solution to this problem. The combination of computer vision technology with smartphone cameras has the potential to revolutionize diagnostics by providing quick and accurate results at the point-of-care.
Key Takeaways:
- Rapid antimicrobial susceptibility testing is crucial in combating antibiotic resistance.
- Traditional culture-based methods for antimicrobial susceptibility testing are time-consuming, leading to inappropriate antibiotic prescribing.
- Smartphone-based computer vision for rapid antimicrobial susceptibility testing represents an innovative solution to this problem.
Understanding Antimicrobial Resistance and its Impact
Antimicrobial resistance (AMR) is a global health threat that occurs when bacteria, viruses, fungi, and parasites become resistant to antimicrobial drugs used to treat infections. The overuse and misuse of antibiotics in humans, animals, and agriculture have accelerated the development of AMR, making it harder and more expensive to treat infections, leading to prolonged illnesses, disability, and death.
According to the World Health Organization (WHO), AMR is one of the top 10 global public health threats facing humanity, and it is estimated to cause 700,000 deaths annually worldwide. If current trends continue, it is predicted that AMR will cause 10 million deaths per year by 2050, and will cost the global economy $100 trillion in lost productivity and healthcare costs.
Effective diagnostic tools that can quickly identify the bacteria causing an infection and determine its susceptibility to antibiotics are essential to combat this problem. Rapid antimicrobial susceptibility testing (AST) can guide appropriate antibiotic treatment and help reduce the use of broad-spectrum antibiotics, which contribute to the development of antimicrobial resistance.
The Significance of Rapid Antimicrobial Susceptibility Testing
Rapid antimicrobial susceptibility testing is essential in guiding appropriate antibiotic treatment and reducing the spread of antimicrobial resistance. Traditionally, laboratory-based testing methods can take up to several days to produce results, which can delay effective treatment and increase the risk of complications for patients.
Point-of-care testing, which can provide quick and accurate results at the patient's bedside, has become increasingly important in clinical practice. Rapid antimicrobial susceptibility testing at the point-of-care can lead to early and appropriate antibiotic therapy, improve patient outcomes, and reduce healthcare costs.
Introduction to Smartphone-Based Computer Vision
Computer vision is a field of artificial intelligence that focuses on enabling computers to interpret and analyze digital images and videos. This technology has significant applications in healthcare, where it can be used for medical imaging, disease diagnosis, and treatment planning. Smartphones equipped with high-quality cameras can capture digital images for analysis using computer vision technology, making them an increasingly popular tool for diagnostics.
The camera and display quality of modern smartphones has reached impressive levels, making them ideal for capturing and analyzing images. Using smartphones for diagnostics enables point-of-care testing, making it faster and easier to obtain results without requiring sophisticated laboratory equipment.
Digital Image Analysis and Machine Learning Algorithms
Digital image analysis is the process of extracting meaningful information from digital images using mathematical algorithms. In the context of smartphone-based computer vision for rapid antimicrobial susceptibility testing, this process involves capturing digital images of bacterial cultures and analyzing them to determine the susceptibility of the bacteria to different antibiotics.
Machine learning algorithms play a crucial role in automating the analysis of these images. By training algorithms on large datasets of images labeled with known antibiotic susceptibility profiles, machine learning can identify patterns and features in new images, allowing for accurate and rapid identification of bacterial susceptibility.
This approach has several benefits. First, it is highly accurate, with studies demonstrating excellent agreement with traditional culture-based methods. Second, it is much faster, with results available in as little as 30 minutes, compared to several days for traditional methods. Finally, it is highly scalable, with the potential to process large numbers of samples in parallel using inexpensive and widely available hardware.
"Digital image analysis and machine learning algorithms have the potential to revolutionize diagnostics by enabling faster and more accurate identification of antimicrobial susceptibility profiles."
Advantages of Smartphone-Based Diagnostics
Smartphone-based diagnostics have significant advantages over traditional diagnostic methods. Firstly, smartphone-based diagnostics are highly accessible. With over 3 billion smartphone users worldwide, the technology is widespread and readily available. A smartphone-based diagnostic test can be performed anywhere, anytime, with no need for specialized equipment or facilities.
Secondly, smartphone-based diagnostics are affordable, making them an ideal solution for resource-limited settings. The cost of a smartphone is significantly lower than that of traditional diagnostic equipment, making it a cost-effective option for healthcare providers and patients alike.
Thirdly, smartphone-based diagnostics are portable, enabling patients to receive timely diagnoses even in remote or underserved areas. By leveraging the power of smartphone technology, healthcare providers can extend diagnostic services to populations that were previously difficult to reach.
In addition to these advantages, smartphone-based diagnostics are also highly customizable. Developers can create apps that are tailored to specific diagnostic needs, allowing for a wide range of diagnostic tests to be performed on a single device.
The potential of smartphone-based diagnostics in healthcare technology is vast. With the ability to perform a wide range of diagnostic tests quickly, affordably, and accurately, smartphone-based diagnostics have the potential to revolutionize healthcare delivery worldwide.
Development and Validation of Smartphone-Based Computer Vision Systems
The development of smartphone-based computer vision systems for rapid antimicrobial susceptibility testing requires a multidisciplinary approach involving experts in computer vision, microbiology, and clinical practice. The process involves several stages, including image acquisition, processing, analysis, and interpretation.
Stage | Description |
---|---|
Image acquisition | High-quality images of bacterial colonies are captured using a smartphone camera or a portable microscope attachment. |
Processing | The raw image data undergoes digital processing to enhance contrast, correct for lighting and color variations, and remove noise and artifacts. |
Analysis | The processed images are analyzed using machine learning algorithms that have been trained to recognize patterns and features of bacterial growth and drug resistance. |
Interpretation | The results of the analysis are translated into actionable information, such as the identity of the microorganism, the type and level of antimicrobial resistance, and the recommended treatment options. |
The validation of smartphone-based computer vision systems involves rigorous testing and benchmarking against gold standard methods and reference laboratories. The validation should assess the accuracy, sensitivity, specificity, precision, and reproducibility of the results, as well as the usability, feasibility, and acceptability of the technology in different clinical settings.
Standardization is vital for ensuring the reliability and comparability of the results across different devices, platforms, and locations. The development of international standards and guidelines for smartphone-based diagnostics can facilitate the adoption and implementation of this technology in clinical practice.
Case Studies and Success Stories
Smartphone-based computer vision for rapid antimicrobial susceptibility testing has already shown promising results in clinical settings. Several studies have demonstrated the efficacy of this technology in guiding appropriate antibiotic treatment and improving patient outcomes.
Case Study 1: Rapid Diagnosis of Urinary Tract Infections
In a study conducted by researchers at the University of California, Los Angeles, smartphone-based computer vision technology was used to rapidly diagnose urinary tract infections (UTIs). The system was able to accurately detect and classify bacteria in urine samples with a sensitivity and specificity of 96% and 99%, respectively. This technology has the potential to significantly reduce the time and cost associated with traditional laboratory testing for UTIs.
Case Study 2: Rapid Identification of Tuberculosis
In a study conducted by researchers at the University of California, Berkeley, smartphone-based computer vision technology was used to rapidly identify tuberculosis (TB) in sputum samples. The system was able to accurately detect TB with a sensitivity and specificity of 96% and 94%, respectively. This technology has the potential to significantly improve the diagnosis of TB in resource-limited settings where traditional laboratory testing may not be available.
Success Story: Detecting Antibiotic Resistance in Ghana
In a successful pilot program, smartphone-based computer vision technology was used to detect antibiotic resistance in Ghana. The system was able to rapidly identify antibiotic-resistant bacteria in patient samples and guide appropriate antibiotic treatment. This technology has the potential to improve antibiotic stewardship and combat the growing problem of antibiotic resistance in low-resource settings.
"Smartphone-based computer vision has the potential to revolutionize diagnostics and improve patient outcomes, particularly in resource-limited settings."
Future Directions and Challenges
As smartphone-based computer vision for rapid antimicrobial susceptibility testing continues to evolve, there are many potential future directions for this technology. One possibility is the development of multi-drug susceptibility testing to provide a more comprehensive analysis of antimicrobial resistance. The integration of artificial intelligence and deep learning algorithms may also enhance the accuracy and efficiency of digital image analysis.
However, there are challenges to be addressed before widespread adoption of this technology. Standardization of testing protocols and data analysis methods will be crucial to ensure accurate and reliable results. Additionally, data privacy and security must be carefully considered to protect patient information.
Regulatory considerations must also be addressed to ensure that smartphone-based computer vision systems comply with local and international regulations and guidelines. This will require collaboration between healthcare providers, researchers, and regulatory bodies to establish a clear regulatory framework.
Integration into Clinical Practice
The integration of smartphone-based computer vision systems into clinical practice has the potential to revolutionize diagnostics and antimicrobial stewardship. To achieve successful implementation, collaboration between healthcare providers, researchers, and technology developers is crucial.
One of the main advantages of smartphone-based diagnostics is their accessibility, affordability, and portability. These features are particularly important in resource-limited settings, where traditional laboratory-based diagnostics may not be available or feasible.
Advantages of Smartphone-Based Diagnostics |
---|
Accessibility |
Affordability |
Portability |
Furthermore, the use of rapid antimicrobial susceptibility testing can guide appropriate antibiotic treatment, reducing the risk of antibiotic resistance. This approach can also help healthcare providers to optimize antibiotic therapy and reduce treatment costs.
However, the integration of smartphone-based computer vision systems into clinical practice also poses some challenges. One of the main challenges is standardization, as there is currently no standardized protocol for digital image acquisition and analysis.
"The development of standardized protocols and guidelines is necessary to ensure the accuracy and reliability of smartphone-based computer vision systems for rapid antimicrobial susceptibility testing."
Other challenges include data privacy and regulatory considerations, particularly in light of the General Data Protection Regulation (GDPR) implemented in the European Union. Therefore, it is essential that healthcare providers and technology developers work together to address these challenges.
The potential benefits of smartphone-based computer vision for rapid antimicrobial susceptibility testing are significant, but successful implementation requires collaboration and careful consideration of the challenges and limitations. By working together, healthcare providers and technology developers can harness the full potential of this innovative technology to improve patient outcomes and combat antibiotic resistance.
Conclusion
Smartphone-based computer vision for rapid antimicrobial susceptibility testing has the potential to revolutionize diagnostics and combat antibiotic resistance. By combining computer vision technology with smartphone cameras, healthcare providers can obtain quick and accurate results for effective antibiotic treatment.
The global impact of antimicrobial resistance highlights the need for efficient diagnostic tools to combat this problem. Rapid antimicrobial susceptibility testing is crucial in guiding appropriate antibiotic treatment and can be achieved through point-of-care testing.
Smartphones equipped with high-quality cameras can capture digital images for analysis. Through digital image analysis and the use of machine learning algorithms, accurate and reliable results can be obtained. The potential benefits of smartphone-based diagnostics include accessibility, affordability, and portability, particularly in resource-limited settings.
Developing and validating smartphone-based computer vision systems for rapid antimicrobial susceptibility testing is essential for ensuring accuracy and reliability. Successful case studies and success stories highlight the impact of these systems on patient outcomes and antibiotic stewardship.
Future directions of smartphone-based computer vision for rapid antimicrobial susceptibility testing include standardization, data privacy, and regulatory considerations. Collaboration between healthcare providers, researchers, and technology developers is crucial for integrating these systems into clinical practice.