Point-of-Care Testing: A Review of Image Analysis Methods & Applications
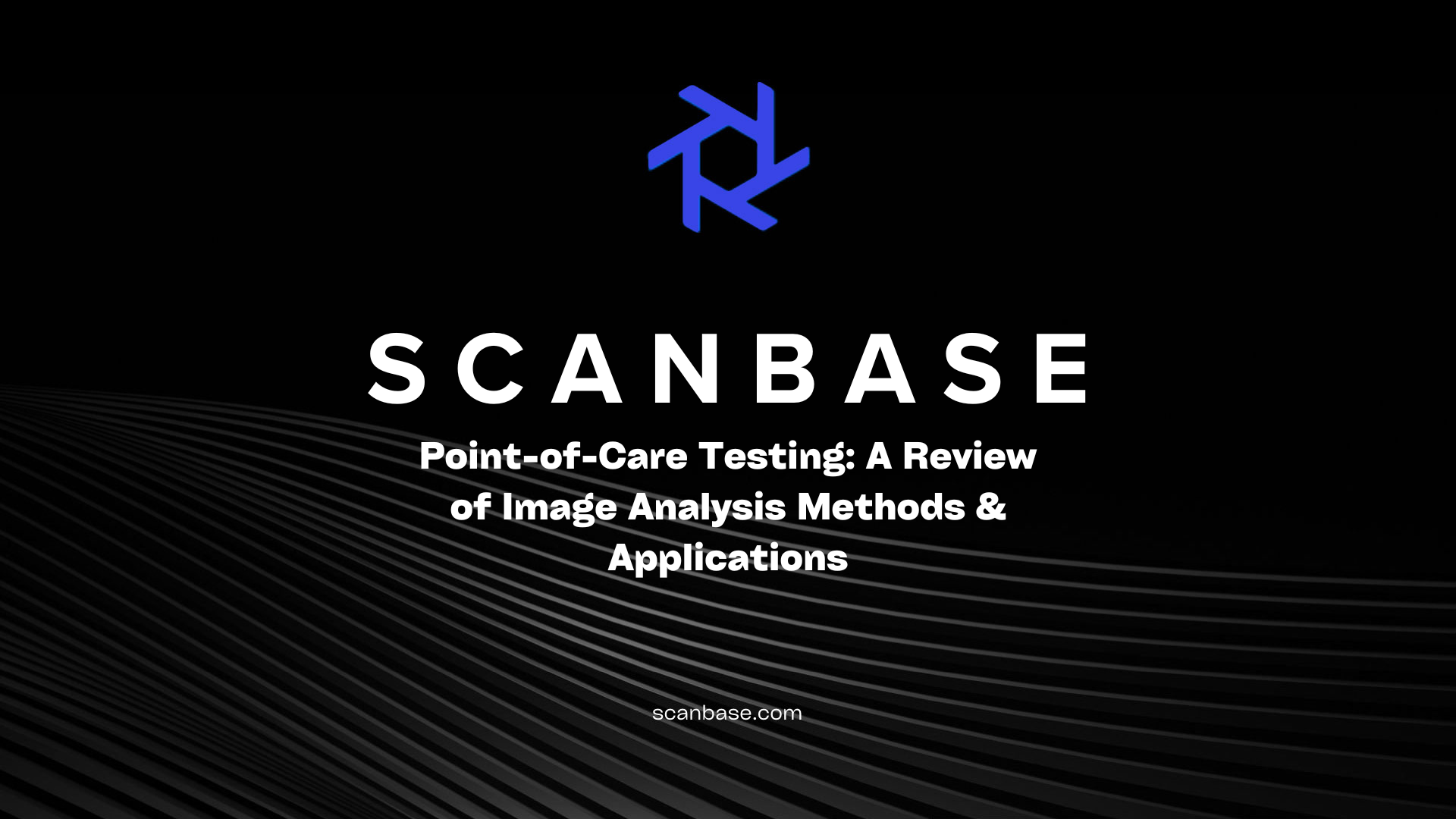
Point-of-care testing (POCT) has become an increasingly popular method for medical diagnosis due to its convenience and rapid results. POCT involves performing medical tests at or near the point of care, which eliminates the need for sending samples to a laboratory for analysis. This approach has the potential to improve patient outcomes, reduce costs, and enhance the efficiency of healthcare systems.
One critical aspect of POCT is image analysis, which plays a crucial role in the interpretation of medical images. In this article, we will review the different image analysis methods used in POCT and their applications in medical diagnostics.
Key Takeaways
- POCT involves performing medical tests at or near the point of care.
- Image analysis is a critical aspect of POCT and plays a crucial role in the interpretation of medical images.
- This article will review the different image analysis methods used in POCT and their applications in medical diagnostics.
Advancements in Point-of-Care Testing Technology
Point-of-care testing (POCT) has undergone significant advancements in recent years. With the development of portable devices and rapid testing methods, POCT has become more accessible and efficient compared to traditional laboratory testing. These technological advancements have improved healthcare delivery by enabling faster diagnosis and treatment of patients.
There are several benefits to POCT, including faster turnaround times, reduced costs, and improved patient outcomes. The ability to perform tests at the point of care can eliminate the need for additional appointments and reduce the risk of delayed diagnosis and treatment. This is particularly important in emergency situations where time is of the essence, and rapid decisions need to be made.
POCT devices come in various forms, from handheld devices to automated systems. They are designed to be user-friendly, with simple interfaces that require minimal training. There are also several testing methods available, including immunoassays, nucleic acid amplification, and microscopy. These methods allow for a wide range of diagnostic tests to be performed, from infectious disease testing to cancer diagnosis and monitoring.
Advancements in Point-of-Care Testing Technology
One of the most significant advancements in POCT technology is the development of biosensors. Biosensors are devices that use biological molecules to detect and measure specific substances in a sample. They are highly sensitive and specific, allowing for accurate and reliable test results.
Biosensor | Application |
---|---|
Glucose Biosensor | Diabetes monitoring |
Troponin Biosensor | Heart attack diagnosis |
Protein Biosensor | Cancer diagnosis and monitoring |
Another significant advancement is the development of microfluidic devices. Microfluidics involves the manipulation of small volumes of fluids in micrometer-sized channels or chambers. This technology allows for the integration of multiple analytical processes into a single device, reducing the need for complex laboratory equipment.
POCT technologies are constantly evolving, with ongoing research in areas such as nanotechnology and microelectromechanical systems. These advancements have the potential to revolutionize healthcare delivery by providing faster, more accurate, and more accessible diagnostic testing.
Introduction to Image Analysis in Point-of-Care Testing
As the demand for rapid and accurate medical diagnostics increases, image analysis has become an essential tool in point-of-care testing. Image analysis involves the processing and interpretation of images to extract relevant information for medical diagnosis and treatment monitoring.
In point-of-care testing, image analysis techniques are used to analyze images obtained from medical devices such as microscopes, scanners, and cameras. These images can provide valuable information about the presence and severity of medical conditions, allowing for timely and accurate diagnosis and treatment.
Image analysis techniques can be used for a variety of applications in the healthcare field, including identifying cancer cells, analyzing tissue samples, and monitoring the progression of diseases such as diabetes and Alzheimer's disease.
Common Image Analysis Methods in Point-of-Care Testing
Image analysis is a crucial component of point-of-care testing, as it enables healthcare providers to interpret test results accurately and efficiently. There are several common image analysis methods used in point-of-care testing:
Image Analysis Method | Description | Application in Point-of-Care Testing |
---|---|---|
Image Segmentation | The process of dividing an image into multiple segments to isolate regions of interest | Used to identify specific features such as cells or pathogens |
Feature Extraction | The identification and extraction of specific characteristics from an image | Used to measure features such as size or shape of cells or pathogens |
Pattern Recognition | The identification of patterns within an image | Used to identify specific cell or pathogen types |
Classification | The grouping of similar cells or pathogens based on shared characteristics | Used to differentiate between normal and abnormal cells or pathogens |
Image analysis methods are used to analyze various types of medical images, such as X-rays, MRIs, and ultrasound images. In point-of-care testing, these methods are used to analyze images obtained from portable testing devices, such as lateral flow assays and microfluidic chips.
The use of image analysis methods in point-of-care testing has several advantages, including the ability to provide rapid and accurate results, as well as the potential for remote diagnosis and monitoring. These methods also have the potential to reduce healthcare costs and improve patient outcomes.
Challenges in Image Analysis for Point-of-Care Testing
Although image analysis is a promising tool for point-of-care testing, there are several challenges that need to be addressed to ensure accurate and reliable results.
Image quality: One of the primary challenges is ensuring the quality of the images captured. Poor image quality can lead to inaccurate results and compromised diagnoses. Therefore, it is essential to develop strategies for improving image quality, such as incorporating noise reduction techniques and optimizing illumination conditions.
Noise reduction: Another challenge is dealing with noise that can arise during the image capture process. Noise can introduce artifacts into the image data and affect the accuracy of any subsequent analyses. Researchers are developing various algorithms to effectively reduce noise and improve image quality.
Standardization: There is a need for standardized image analysis techniques and protocols to ensure consistency and reproducibility in point-of-care testing. Standardization can minimize inter-operator variability and enable comparisons of results across different platforms.
Challenges in Image Analysis for Point-of-Care Testing:
"Poor image quality can lead to inaccurate results and compromised diagnoses."
- Image quality
- Noise reduction
- Standardization
Emerging Image Analysis Technologies in Point-of-Care Testing
As point-of-care testing continues to gain momentum in medical diagnostics, emerging image analysis technologies are poised to play a critical role in its advancement. These technologies are focused on developing more accurate and efficient methods of analyzing medical images, ultimately enhancing the accuracy and speed of diagnostic testing.
Deep learning is one such technology that has shown significant promise in the field of image analysis. It involves training a computer algorithm to recognize patterns in complex data sets, such as medical images. This technology has the potential to improve the accuracy of point-of-care testing by allowing for more detailed image analysis and identification of subtle abnormalities.
Artificial intelligence (AI) is another emerging technology that has the potential to revolutionize point-of-care testing. AI algorithms can analyze large data sets quickly and accurately, providing insights that may not be easily recognizable by human analysis. This technology can greatly improve the speed and accuracy of diagnostic testing.
Machine learning is a related technology that involves training a computer algorithm to improve its performance on specific tasks over time. This technology can be applied to medical image analysis to improve accuracy and speed.
Overall, these emerging image analysis technologies show great promise in the field of point-of-care testing. As research continues, it is likely that these technologies will become more prevalent in medical diagnostics, leading to faster and more accurate diagnoses for patients.
Applications of Image Analysis in Point-of-Care Testing
Image analysis techniques have numerous applications in point-of-care testing and have proven to be extremely helpful in the diagnosis and treatment of various medical conditions. Here are some examples:
Medical Condition | Image Analysis Technique | Application |
---|---|---|
Diabetic Retinopathy | Image Segmentation | Identification of microaneurysms and hemorrhages in retinal images |
Malaria | Color Analysis | Identification of malaria parasites in blood smears |
Cancer | Pattern Recognition | Detection and classification of cancer cells in histopathology images |
Alzheimer's Disease | Texture Analysis | Quantification of hippocampal atrophy in MRI scans |
As seen from these examples, image analysis has proven to be a valuable tool in point-of-care testing, allowing for efficient and accurate diagnosis of various medical conditions. Ongoing research and development in this field hold great promise for the future of medical diagnostics.
Future Directions and Opportunities in Image Analysis for Point-of-Care Testing
As image analysis continues to play an important role in point-of-care testing, there are several future directions and opportunities that can further enhance the field. Some of these include:
- Improving image quality: One of the key challenges in image analysis for point-of-care testing is the quality of the images produced. Future advancements in technology can focus on improving image quality and reducing noise to enhance the accuracy of test results.
- Standardization: There is a need for standardization in image analysis methods and techniques to ensure consistency and accuracy across different devices and test settings.
- Integration with electronic health records (EHRs): Integrating image analysis with EHRs can help streamline the diagnostic process and improve patient outcomes by providing a more comprehensive view of patient health.
- Research collaborations: Collaboration between researchers from different fields, such as computer science and medicine, can help bring innovative ideas and solutions to the forefront.
- Development of novel technologies: Emerging technologies such as deep learning, artificial intelligence, and machine learning show promise in enhancing image analysis for point-of-care testing. Further development and refinement of these technologies can revolutionize medical diagnostics.
Overall, the future of image analysis in point-of-care testing is bright, with opportunities for ongoing research and development. As technology continues to advance, there is potential for improved accuracy, efficiency, and patient outcomes in medical diagnostics.
Conclusion
Point-of-care testing has become an essential tool in medical diagnostics, offering rapid and accurate results that can significantly impact patient outcomes. Image analysis plays a crucial role in point-of-care testing, allowing for the detection and monitoring of various medical conditions and diseases.
As highlighted in this article, numerous image analysis methods and technologies are currently in use or under development, offering exciting opportunities for advancement in the field. However, challenges such as image quality and standardization must be addressed to ensure accurate and reliable results.
Overall, the future of image analysis in point-of-care testing is promising, with ongoing research and development supporting the potential for significant improvements in medical diagnostics. Continued collaboration between technology developers, medical professionals, and researchers is crucial in driving progress and enhancing the capabilities of point-of-care testing.