Optimizing Machine Learning Approaches for Smartphone-based Point-of-Care Testing
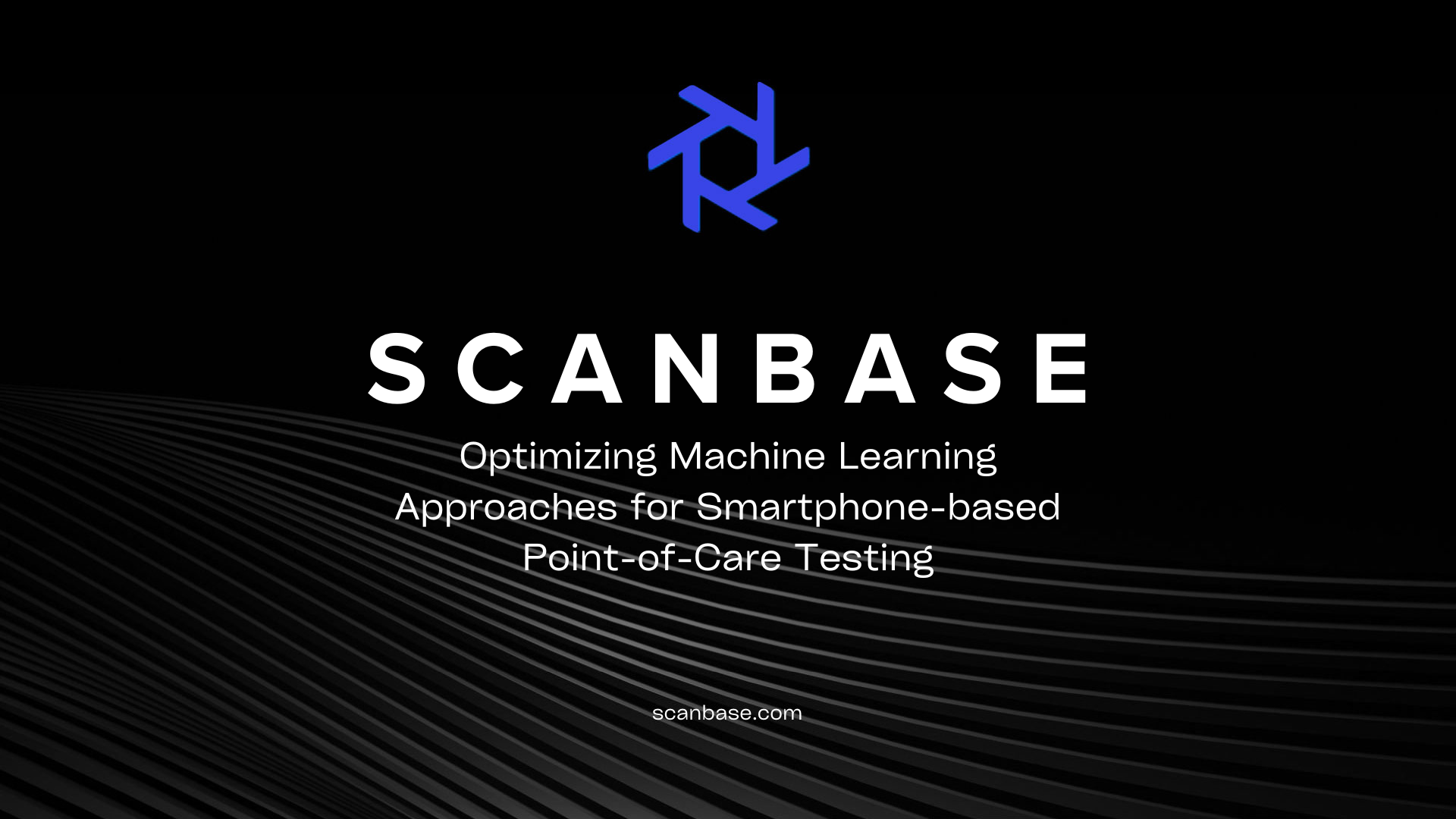
In recent years, there has been a growing interest in smartphone-based point-of-care testing due to its potential to revolutionize healthcare. With the introduction of machine learning approaches, the possibilities are endless. By optimizing these approaches, we can improve the speed and accuracy of diagnostics and enhance overall patient care. In this section, we will explore the significance of machine learning approaches for smartphone-based point-of-care testing and their impact on healthcare technology.
Key Takeaways:
- Machine learning approaches can revolutionize smartphone-based point-of-care testing in healthcare.
- Optimizing these approaches can enhance the accuracy and speed of diagnostics, providing valuable insights for patient care.
Understanding Smartphone-based Point-of-Care Testing
Smartphone-based point-of-care testing is a type of medical testing that can be conducted using a smartphone or other mobile device. These tests are designed to provide rapid and convenient diagnostic information at the point of care, without the need for a laboratory or specialized equipment.
Point-of-care testing is an increasingly important aspect of modern healthcare, as it allows for faster and more accurate diagnoses, leading to better patient outcomes and improved treatment options. Smartphone-based point-of-care testing takes this idea one step further, by providing the same benefits in a mobile and easily-accessible format.
Smartphone-based point-of-care testing can be conducted using a variety of methods, including physical attachments that connect to the device, or through specialized health applications that use the device's camera and other built-in features. These tests can cover a range of medical conditions, from basic health measurements like blood pressure and heart rate, to more complex medical procedures like blood glucose monitoring or infectious disease testing.
The benefits of smartphone-based point-of-care testing are numerous. It provides a more convenient and accessible option for patients who may not have access to traditional medical facilities, as well as those who require frequent testing or monitoring. Additionally, it can speed up the diagnostic process, leading to faster treatment and better patient outcomes.
Example of Smartphone-based Point-of-Care Testing
One example of smartphone-based point-of-care testing is the use of a mobile app for diagnosing skin cancer. The app uses the device's camera to capture images of moles or other skin lesions, and then analyzes them using machine learning algorithms to determine the likelihood of cancer. This provides a fast and convenient way for patients to receive initial diagnostic information, without the need for a specialist visit or biopsy.
Smartphone-based point-of-care testing is a rapidly growing field, with new tests and applications being developed all the time. As this technology continues to advance, it has the potential to revolutionize healthcare by providing faster, more accurate, and more accessible diagnostic information to patients around the world.
Introduction to Machine Learning in Healthcare
In recent years, machine learning has emerged as a powerful tool in healthcare technology. Machine learning algorithms can be used to analyze vast amounts of data and provide valuable insights for diagnostics and patient care.
Machine learning involves training computer programs to recognize patterns and make predictions based on data. In healthcare, these algorithms can be used to identify disease symptoms, predict treatment outcomes, and even detect potential outbreaks. With the rise of mobile health and smartphone-based point-of-care testing, machine learning approaches have become increasingly relevant in healthcare.
Machine Learning Algorithms for Smartphone-based Point-of-Care Testing
Machine learning algorithms can be applied to smartphone-based point-of-care testing to provide accurate and fast diagnostics. These algorithms learn from the data collected through smartphone applications and provide valuable insights for healthcare providers.
There are several machine learning techniques that can be applied to point-of-care testing. These include classification algorithms, clustering algorithms, and regression algorithms.
Classification algorithms are used to categorize data into specific classes. In the case of point-of-care testing, these algorithms can classify patient data into different diseases or conditions.
Clustering algorithms group data points into similar groups, allowing healthcare providers to identify patterns and trends in patient data. This can help with early detection and treatment of diseases.
Regression algorithms are used to predict future outcomes based on historical data. In the case of point-of-care testing, these algorithms can predict the likelihood of a patient developing a specific disease or condition.
The choice of machine learning algorithm depends on the type of data and the specific diagnostic needs. By utilizing these algorithms, healthcare providers can optimize the accuracy and speed of point-of-care testing.
Advantages of Machine Learning in Point-of-Care Testing
There are several advantages of utilizing machine learning techniques in point-of-care testing. Firstly, it can improve the accuracy of diagnostics. Machine learning algorithms can analyze large amounts of data from smartphone-based point-of-care testing and provide more precise and targeted results. This can lead to better treatment plans and outcomes for patients.
Secondly, machine learning can speed up the diagnostic process. Traditional testing methods can take days or even weeks to produce results. With smartphone-based point-of-care testing and machine learning algorithms, results can be obtained in a matter of minutes. This saves time for healthcare professionals and improves the overall efficiency of the healthcare system.
Finally, the implementation of machine learning in point-of-care testing can enhance overall patient care. By providing more accurate and rapid results, patients can receive timely and appropriate treatment plans. The convenience and accessibility of smartphone-based point-of-care testing can also encourage patients to seek medical attention sooner, leading to better health outcomes.
Overall, the advantages of machine learning in point-of-care testing are clear. With the development of smarter algorithms and improved healthcare technologies, we can look forward to a future of more accurate, efficient, and patient-centered healthcare.
Challenges and Limitations of Machine Learning Approaches
While machine learning approaches for smartphone-based point-of-care testing offer numerous benefits, there are also several challenges and limitations that must be addressed.
Data Privacy
One of the biggest concerns in the implementation of machine learning approaches for healthcare is data privacy. As smartphones collect sensitive patient data, it is crucial to ensure that the data is kept safe and confidential. The use of encryption and other security measures can help alleviate these concerns.
Algorithm Bias
Another challenge in the application of machine learning approaches is algorithm bias. If the training data sets are not diverse enough, the algorithms may produce biased results, which can impact the accuracy of diagnoses. Therefore, it is essential to ensure that data sets used for training are representative of the population being tested.
Validation Studies
The accuracy and reliability of machine learning approaches for point-of-care testing must be validated through rigorous studies. These studies should include diverse populations and take into account factors such as age, ethnicity, and socioeconomic status to ensure the algorithms are effective for all patients.
Despite these challenges and limitations, the potential benefits of machine learning approaches for smartphone-based point-of-care testing are immense. Through ongoing research and development efforts, these approaches can help transform healthcare and improve patient outcomes.
Implementation and Integration of Machine Learning in Healthcare
Implementing and integrating machine learning in healthcare requires a robust infrastructure to support the analysis of vast amounts of data generated through smartphone diagnostics. This includes data management systems, secure data sharing protocols, and reliable wireless connections.
One of the key benefits of machine learning in point-of-care testing is the potential to improve healthcare access and reduce costs. By enabling patients to conduct tests from the comfort of their homes, healthcare providers can save time and resources on hospital visits.
However, challenges such as data privacy and algorithm bias must be addressed to ensure that machine learning approaches are effective and equitable for all patients. Validation studies are necessary to ensure that machine learning algorithms provide accurate and unbiased results.
Integrating machine learning into healthcare also requires collaboration between health professionals, software developers, and data scientists. Multidisciplinary teams can work together to optimize machine learning approaches and develop applications that meet the specific needs of patients and healthcare providers.
As smartphone-based point-of-care testing becomes more widespread, machine learning will play an increasingly important role in improving patient care. By leveraging this technology, healthcare providers can harness the power of data to provide more accurate and efficient diagnostics, leading to better health outcomes for patients.
Future Directions and Potential Applications
The potential applications of machine learning approaches for smartphone-based point-of-care testing are extensive. As the technology behind machine learning continues to evolve, we can expect to see significant advancements in mobile health and diagnostics.
Personalized Medicine
One exciting area of potential application is personalized medicine. By leveraging machine learning algorithms, healthcare professionals can use smartphone-based point-of-care testing to tailor treatment plans to individual patients. This approach can lead to improved patient outcomes and a more effective use of healthcare resources.
Remote Monitoring
Machine learning can also enhance remote monitoring of patients. By using smartphone-based point-of-care testing to collect data, machine learning algorithms can provide real-time analysis of patient health indicators. This approach can improve patient care by enabling healthcare providers to intervene early in disease progression or to make necessary adjustments to treatment plans.
Early Disease Detection
Machine learning approaches for smartphone-based point-of-care testing also have the potential to improve early disease detection. By analyzing data collected from smartphone health applications, machine learning algorithms can detect subtle changes in health indicators that may not be visible to the naked eye. This approach can lead to earlier diagnosis and treatment, ultimately improving patient outcomes.
Improved Data Analysis
Finally, machine learning approaches can improve data analysis in healthcare. As data collection continues to increase, it can be difficult to make sense of the vast volumes of information generated. Machine learning can help to identify patterns and trends in the data, providing valuable insights for diagnostics and treatment.
The future of healthcare technology looks promising, and machine learning approaches for smartphone-based point-of-care testing will play a crucial role in improving patient care worldwide.
Case Studies and Success Stories
Machine learning approaches and smartphone-based point-of-care testing have already demonstrated their immense potential in improving healthcare technology. Here are a few success stories and case studies that showcase their impact:
Case Study | Success Story |
---|---|
Cancer Detection | Researchers at Stanford University used a smartphone-based point-of-care testing system that uses machine learning to detect cancerous lesions on the skin with an accuracy rate of 90%. This system can be used in remote areas with limited access to medical facilities, where skin cancer is often overlooked and undertreated. |
Diabetes Management | Smartphone-based point-of-care testing systems that use machine learning algorithms can also help manage diabetes. A study conducted at the University of California, Berkeley, developed a system that uses machine learning to predict blood glucose levels and provides insulin dosage recommendations. The system was found to be more accurate than other traditional diabetes management methods. |
Infectious Disease Diagnosis | Researchers at the University of Minnesota used a smartphone-based point-of-care testing system that uses machine learning algorithms to diagnose infectious diseases such as HIV and syphilis. The system was found to be highly accurate and provided results in a matter of minutes, allowing for immediate treatment and reducing the burden on healthcare facilities. |
These success stories highlight the potential of machine learning approaches in smartphone-based point-of-care testing to transform healthcare technology. As more research and development is conducted, we can expect to see even more innovative applications that enhance patient care and improve health outcomes.
Conclusion
The potential of machine learning approaches for smartphone-based point-of-care testing is immense. By optimizing these approaches, we can revolutionize healthcare, making diagnostics more accurate, convenient, and accessible to people around the world.
Machine learning algorithms have the ability to analyze data collected from smartphone-based point-of-care testing, providing valuable insights for diagnostics. They can improve accuracy, speed up diagnostics, and enhance overall patient care.
Of course, there are challenges associated with machine learning approaches, such as data privacy, algorithm bias, and the need for validation studies. But with proper implementation and integration, these challenges can be overcome.
As we look to the future of healthcare technology, machine learning approaches combined with smartphone-based point-of-care testing are poised to play a crucial role in improving patient care worldwide.
The Future of Machine Learning in Healthcare
The potential applications of machine learning in healthcare are endless. As more and more healthcare providers adopt these approaches, we can expect to see further optimization and expansion.
With the rise of mobile health, machine learning techniques can now be applied to smartphone-based point-of-care testing, providing a new level of convenience and accessibility for patients. With proper infrastructure and integration, machine learning algorithms may one day be able to detect illnesses before symptoms even appear.
The success stories and case studies of machine learning in smartphone-based point-of-care testing are already showing how these technologies can make a significant impact in healthcare. By harnessing the power of machine learning algorithms, we can transform diagnostics at the touch of a button.
In conclusion, the future of healthcare technology looks promising—and machine learning approaches for smartphone-based point-of-care testing will play a crucial role in improving patient care worldwide.