Image Segmentation Techniques for Rapid Diagnostic Test Analysis on Smartphones
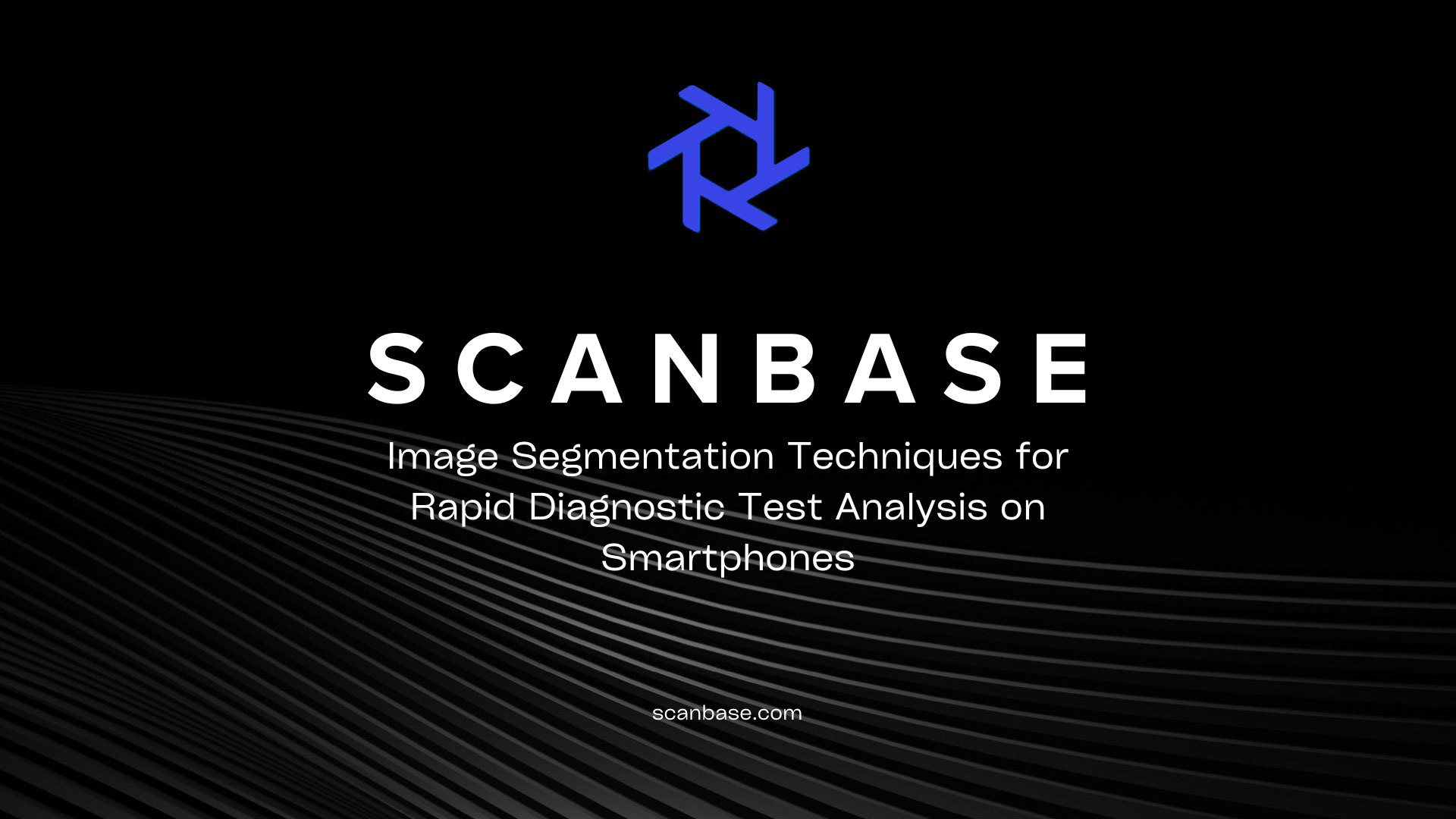
Healthcare is constantly evolving, and technology is at the forefront of that progress. Image segmentation techniques have been used in diagnostic test analysis for some time now, but the innovation in the field is moving towards implementing these techniques on smartphones to enable the delivery of rapid results.
Smartphone-based image segmentation offers a faster, more precise, and cost-effective way of analyzing diagnostic test images without the need for expensive equipment. With the use of computer vision for diagnostic test analysis, image segmentation on smartphones has the potential to revolutionize healthcare by enhancing the speed and accuracy of disease diagnosis.
Key Takeaways:
- Smartphone-based image segmentation is a cost-effective and efficient way of analyzing diagnostic test images.
- The technology offers faster and more precise results, thanks to the use of computer vision for diagnostic test analysis.
- Image segmentation on smartphones can revolutionize healthcare by enhancing the speed and accuracy of disease diagnosis.
The Significance of Diagnostic Test Analysis
Accurate and timely diagnostic test analysis is crucial to ensuring effective healthcare. Traditional analysis methods, however, often present challenges such as time-consuming procedures and limited accessibility, especially in remote areas. This is where rapid test image analysis on smartphones comes into play. By utilizing smartphone health analysis, healthcare professionals can obtain faster and more precise results, enabling quick interventions and treatments.
The significance of diagnostic test analysis cannot be overemphasized, especially with regard to infectious disease detection and management. Rapid test image analysis on smartphones can provide timely results in remote and resource-limited areas, allowing for more effective treatment and containment of diseases. The potential impact of smartphone-based analysis on healthcare is immense, and it is clear that this technology is transforming the healthcare industry.
Understanding Image Segmentation Techniques
Image segmentation techniques are crucial in diagnostic test analysis using smartphones. These techniques involve the process of separating the image into different segments or regions. The goal is to simplify the image analysis process, making it easier to identify and extract the features of interest in the image. One such technique is mobile image segmentation, which aims to segment images on mobile devices, such as smartphones or tablets.
Mobile image segmentation involves the use of computer vision for diagnostic test analysis, enabling smartphones to perform image analysis even with limited processing power. Computer vision is a field of study that focuses on enabling computers to interpret and understand visual data from the world around them, mimicking human perception.
There are various algorithms and methods used for segmenting diagnostic test images on smartphones. One common method is thresholding, which involves separating the image pixels into regions of interest and background regions based on a specific threshold value. Another method is edge-based segmentation, which involves detecting edges in the image and segmenting the regions between edges.
Overall, understanding image segmentation techniques is critical in enabling smartphones to provide fast, accurate, and cost-effective diagnostic test analysis. The use of mobile image segmentation and computer vision is set to revolutionize healthcare by making diagnostic tests more accessible and affordable, especially in developing countries where traditional methods are unavailable.
The Process of Rapid Test Image Analysis on Smartphones
The process of rapid test image analysis on smartphones involves several steps that enable accurate and efficient segmentation of diagnostic test results. The first step is image acquisition, which involves capturing a clear and high-quality image of the rapid diagnostic test strip using the smartphone's built-in camera. This image is then preprocessed to enhance the contrast and brightness, and to remove any noise or artifacts that could affect the segmentation process.
Next, the image is segmented using mobile image segmentation algorithms that have been specifically designed for diagnostic test analysis. These algorithms use computer vision techniques to identify and isolate different regions of the image, such as the test line and the control line, and to extract quantitative data from these regions. The resulting diagnostic test result segmentation is then analyzed to determine the presence or absence of the target analyte in the sample, such as a virus or a hormone.
While smartphone-based image processing offers numerous benefits, including speed, convenience, and cost-effectiveness, it also poses some challenges and limitations. For example, the processing power of smartphones may be limited, which can affect the speed and accuracy of the segmentation process. Additionally, the quality of the acquired image may vary depending on factors such as lighting conditions and camera settings, which can affect the reliability of the segmentation results.
Efforts are underway to address these challenges and improve the overall performance of smartphone-based image segmentation for rapid diagnostic test analysis. Ongoing research is focused on developing more advanced mobile image segmentation algorithms that can handle the variability in image quality and perform real-time segmentation on low-power devices.
Benefits of Smartphone-Based Image Segmentation
The utilization of smartphone-based image segmentation in diagnostic test analysis offers numerous benefits. One major advantage is speed, as the process can be performed almost instantaneously. The convenience of using smartphones for image segmentation makes it possible for healthcare practitioners to perform diagnostic tests remotely, without the need for a physical laboratory. The cost-effectiveness of the technology is another significant benefit, as specialized equipment or training are not required.
Perhaps most importantly, smartphone-based image segmentation offers a highly accurate and reliable method for diagnostic test result segmentation. The technology applies sophisticated algorithms and techniques to isolate and analyze specific data, producing precise and consistent results. This level of accuracy can be crucial in diagnosing and treating a variety of health conditions, from infectious diseases to chronic illnesses.
Applications of Digital Image Analysis in Healthcare
The application of digital image analysis in healthcare has been revolutionary, and smartphone-based image segmentation has greatly contributed to this field. With the ability to perform fast and accurate diagnostic test analysis, mobile image segmentation has made it possible to detect diseases and monitor health conditions more efficiently. Smartphone health analysis using digital image analysis has vast applications in various diagnostic tests, including blood tests, urine tests, and infectious disease detection.
One significant benefit of digital image analysis in healthcare is the ability to monitor and track treatment progress. Patients can use smartphone-based image segmentation to monitor their health conditions remotely and share results with their healthcare providers in real-time, leading to more accurate diagnosis and improved outcomes. Additionally, healthcare providers can use digital image analysis to diagnose diseases in remote areas or low-resource settings where traditional laboratory infrastructure is unavailable.
Smartphone-based digital image analysis is applicable not only in diagnosis and treatment monitoring but also in surgical procedures. For example, during minimally invasive surgeries, digital image analysis can help surgeons navigate through small body cavities and perform procedures with higher precision and accuracy. In the future, digital image analysis could potentially substitute for traditional optical imaging in surgical procedures, providing real-time image analysis and guidance to surgeons.
Overall, the impact of digital image analysis in healthcare using smartphone-based image segmentation has vast potential, transforming the way we approach disease diagnosis and treatment. With continued research and development, this technology could revolutionize healthcare and improve health outcomes for millions of people worldwide.
Advancements in Smartphone Image Segmentation
The field of smartphone-based image segmentation for diagnostic test analysis has shown impressive advancements in recent years. Innovations in computer vision and machine learning algorithms have greatly improved the accuracy and speed of diagnostic test result segmentation using smartphones.
One recent breakthrough in this field is the development of deep learning algorithms specifically designed for mobile image segmentation. These algorithms allow for faster processing of images and more accurate identification of objects in complex medical images. Additionally, advancements in smartphone camera technology, such as higher resolution and improved autofocus, have further enhanced the quality of diagnostic test images captured using smartphones.
Another significant advancement is the integration of cloud-based image processing with smartphone-based segmentation. This technology allows for more extensive image analysis and deeper insights into medical data. It also enables remote sharing of medical images and real-time analysis with healthcare professionals.
Overall, the future of smartphone-based image segmentation for diagnostic test analysis looks promising with ongoing research and development in this field. By leveraging the potential of computer vision and machine learning, we can expect further improvements in image segmentation accuracy, speed, and accessibility for healthcare professionals and patients.
Challenges and Limitations of Smartphone-Based Image Segmentation
Despite the numerous advantages of smartphone-based image segmentation, there are some challenges and limitations that need to be addressed. One of the significant challenges is the variability in image quality due to lighting conditions, camera resolution, and other factors, which can affect the accuracy of segmentation. Another issue is the limited processing power of smartphones, which can lead to longer processing times and reduced efficiency.
Privacy concerns are also a potential limitation, as smartphone-based image segmentation involves the transfer of sensitive medical information over the internet. Another limitation is the difficulty in maintaining consistency in mobile image segmentation, as there is no universal standard or protocol for implementing these techniques.
Despite these challenges and limitations, ongoing research and development are aimed at addressing these concerns. New algorithms and technologies are being developed to improve the accuracy and efficiency of mobile image segmentation, while efforts are underway to establish standards and protocols for consistent implementation.
Integration of Image Segmentation with Healthcare Systems
As the demand for rapid and accurate diagnostic test analysis continues to increase, there is a growing need for the integration of image segmentation technology with existing healthcare systems. Digital image analysis and smartphone health analysis can help healthcare professionals to analyze diagnostic test images in real-time and remotely monitor patients' health.
Smartphone-based image segmentation can facilitate seamless data transfer from the mobile device to healthcare systems, enabling centralized data storage and analysis. This feature can also enhance the accuracy and reliability of diagnostic test analysis, allowing healthcare professionals to make informed decisions regarding disease diagnosis and treatment.
The integration of image segmentation with healthcare systems can also lead to cost savings and improved patient outcomes. Remote patient monitoring through smartphone-based image segmentation can reduce the need for hospital visits and lower healthcare costs. Additionally, real-time analysis can allow healthcare professionals to detect and diagnose diseases at earlier stages, improving the chances of successful treatment.
Collaboration between technology developers and healthcare professionals is crucial in ensuring the seamless integration of image segmentation technology with existing healthcare systems. Healthcare professionals' inputs can help ensure that the technology meets their needs and is aligned with the healthcare system's requirements.
The integration of image segmentation with healthcare systems is an exciting development in healthcare technology. Smartphone-based image segmentation technology has the potential to revolutionize diagnostic test analysis and improve patient outcomes, making healthcare more efficient and accessible.
Conclusion
In conclusion, image segmentation techniques for rapid diagnostic test analysis on smartphones have the potential to transform healthcare as we know it. The significance of accurate and timely diagnostic test analysis cannot be overstated. Traditional analysis methods often face several challenges, but with innovative solutions like smartphone-based image segmentation, faster and more precise results can be achieved.
The process of rapid test image analysis on smartphones involves acquiring, pre-processing, and segmenting the images. While there are challenges and limitations associated with smartphone-based image segmentation, ongoing research and advancements in technology show promise in overcoming these obstacles.
The benefits of smartphone-based image segmentation include speed, convenience, and cost-effectiveness. The accuracy and reliability of diagnostic test result segmentation achieved through this technology is impressive, and the potential impact on disease diagnosis and treatment monitoring is immense.
Recent advancements and developments in smartphone image segmentation techniques for diagnostic test analysis are encouraging. Innovative algorithms and technologies are enhancing the accuracy and efficiency of diagnostic test image segmentation, and the potential for future advancements is vast.
Integrating image segmentation technology with existing healthcare systems can lead to seamless data transfer, real-time analysis, and remote monitoring of patients' health using smartphone-based image segmentation. Collaboration between technology developers and healthcare professionals is crucial in realizing the full potential of this technology.
In conclusion, the use of image segmentation techniques for rapid diagnostic test analysis on smartphones holds great promise for the future of healthcare. This technology has the potential to revolutionize healthcare delivery, making it faster, more precise, and more affordable for everyone.