Exploring the Role of Deep Learning in Medical Diagnostics
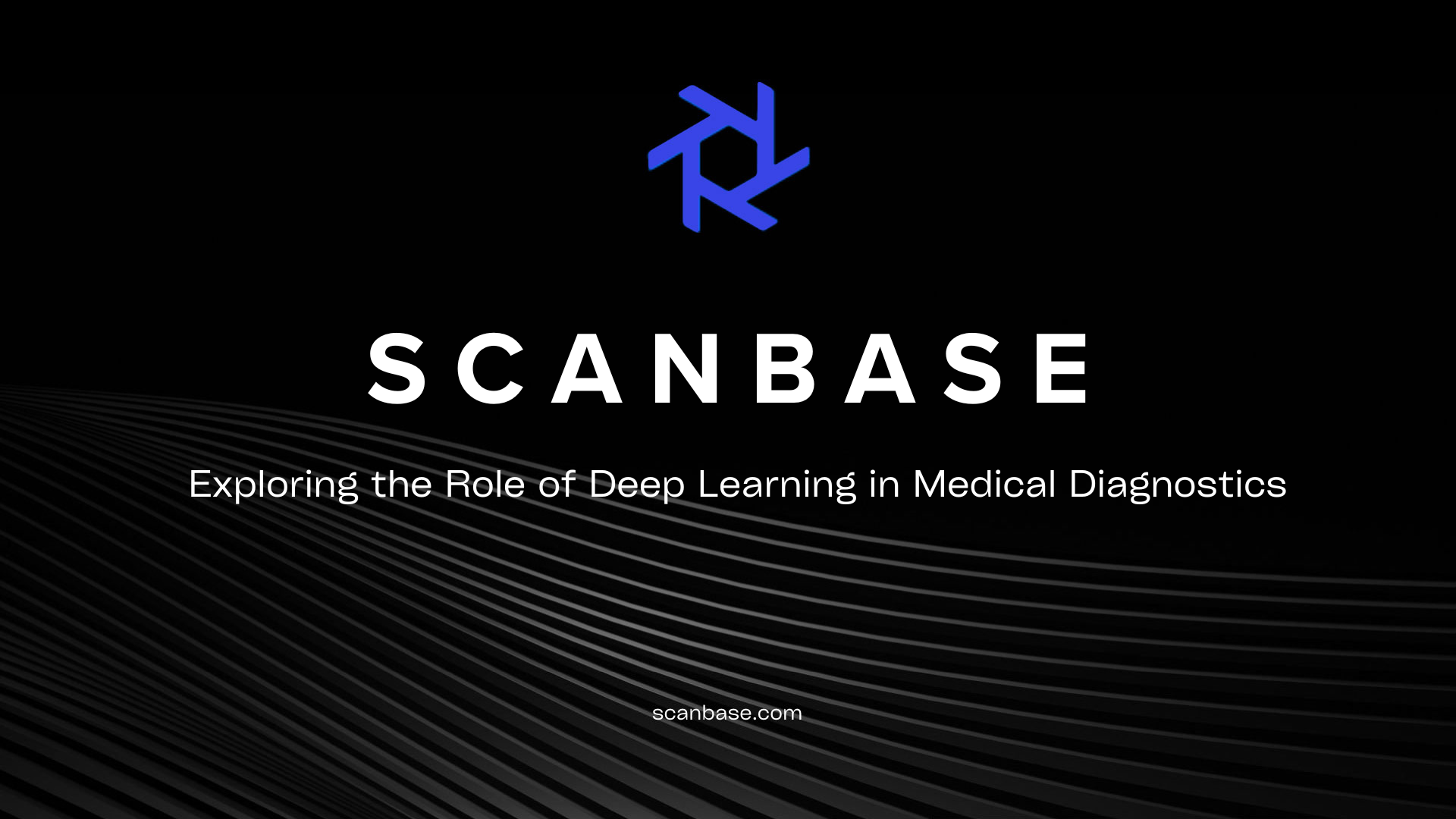
Welcome to our article where we delve into the fascinating world of deep learning and its applications in medical diagnostics. In recent years, deep learning techniques have revolutionized the healthcare industry, offering advanced solutions for disease diagnosis, drug discovery, and patient risk identification. By harnessing the power of artificial intelligence (AI) algorithms, deep learning has the potential to greatly improve accuracy and efficiency in medical diagnostics, ultimately benefiting both patients and healthcare providers.
Deep learning algorithms, ranging from machine learning to deep neural networks, have the ability to analyze complex medical data sources such as ultrasound, magnetic resonance imaging (MRI), mammography, genomics, and computed tomography scans. By accurately predicting and classifying various diseases including Alzheimer's, cancer, diabetes, chronic heart disease, tuberculosis, stroke, and hypertension, deep learning algorithms can assist in early detection and precise diagnosis.
Key Takeaways:
- Deep learning techniques have revolutionized medical diagnostics, offering advanced solutions for disease diagnosis, drug discovery, and patient risk identification.
- Deep learning algorithms analyze complex medical data sources such as ultrasound, MRI, mammography, genomics, and CT scans.
- Accurate prediction and classification of various diseases can be achieved, including Alzheimer's, cancer, diabetes, chronic heart disease, tuberculosis, stroke, and hypertension.
- Deep learning in medical diagnostics enhances accuracy and efficiency, leading to improved patient outcomes and more efficient healthcare delivery.
- Challenges in deep learning-based medical diagnostics include standardization, regulatory approval, and ethical considerations.
Benefits of Deep Learning in Medical Diagnostics
Deep learning has revolutionized the field of medical diagnostics, offering numerous benefits that have a significant impact on patient outcomes. One of the key advantages of deep learning is its ability to enhance the diagnostic process by providing more accurate and timely disease diagnoses. With the help of deep learning algorithms, medical data can be analyzed with high precision, leading to improved diagnostic accuracy and reduced human error.
Another major benefit of deep learning in medical diagnostics is its ability to enable faster processing of patient information, resulting in quicker diagnosis and treatment decisions. This speed can be critical in situations where time plays a crucial role, allowing healthcare providers to take immediate action and potentially save lives.
Furthermore, deep learning techniques provide valuable insights and predictions for disease prognosis, which can aid in the development of personalized treatment plans and preventive measures. By analyzing complex medical data, deep learning algorithms can uncover patterns and correlations that are not easily discernible to humans. This leads to more targeted and effective healthcare interventions, ultimately improving patient outcomes.
Table: Benefits of Deep Learning in Medical Diagnostics
Benefit | Description |
---|---|
Enhanced Diagnostic Accuracy | Deep learning algorithms analyze medical data with high precision, leading to improved accuracy in disease diagnosis. |
Reduced Human Error | By automating the diagnostic process, deep learning algorithms help minimize human error and improve overall diagnostic reliability. |
Quicker Diagnosis and Treatment Decisions | Deep learning enables faster processing of patient information, allowing for quicker diagnosis and timely treatment decisions. |
Insights and Predictions for Disease Prognosis | Deep learning algorithms provide valuable insights and predictions for disease prognosis, contributing to personalized treatment plans and preventive measures. |
Deep Learning Techniques in Medical Imaging
Deep learning techniques have revolutionized medical imaging, enabling more accurate and efficient diagnostic procedures. By utilizing convolutional neural networks (CNNs), deep learning algorithms can analyze various medical images, including X-rays, CT scans, and MRIs, to detect and classify abnormalities. These algorithms can learn complex patterns and features from the images, allowing for early detection of diseases such as cancer, lung nodules, and cardiovascular conditions.
Early Disease Detection with Deep Learning
One of the key advantages of deep learning in medical imaging is its ability to facilitate early disease detection. By analyzing medical images with high precision, deep learning algorithms can identify subtle abnormalities that might go unnoticed by human observers. This early detection can significantly improve patient outcomes by enabling timely intervention and treatment.
Furthermore, deep learning techniques in medical imaging also enable image segmentation, which allows for the precise delineation of structures and organs. This segmentation can be used for more targeted analysis and treatment planning, ensuring that healthcare providers have a better understanding of the disease and its impact on the patient's body.
Disease | Deep Learning Technique | Advantages |
---|---|---|
Cancer | Convolutional Neural Networks (CNNs) | - Early detection of tumors and malignancies - Improved accuracy in tumor classification |
Lung Nodules | Convolutional Neural Networks (CNNs) | - Early detection and characterization of lung nodules - Reduction in false positives and false negatives |
Cardiovascular Conditions | Convolutional Neural Networks (CNNs) | - Early detection of cardiovascular abnormalities - Identification of cardiac risk factors |
Future of Deep Learning in Diagnostics
Deep learning has emerged as a powerful tool in medical diagnostics, and its future holds tremendous promise for further advancements in healthcare. As technology continues to evolve, deep learning algorithms are expected to become even more sophisticated and accurate, enabling the development of more efficient diagnostic tools and systems.
One of the key areas of advancement lies in the improvement of hardware capabilities. High-performance GPUs, for instance, will enhance the processing power of deep learning algorithms, allowing for faster and more accurate analysis of complex medical data. This, combined with the increasing availability of large medical datasets, will facilitate the development and deployment of deep learning-based diagnostic solutions on a larger scale.
Advancements in Deep Learning Techniques
Advancements in deep learning techniques will also play a crucial role in the future of medical diagnostics. Researchers and engineers are constantly working on refining and optimizing deep learning algorithms, allowing them to learn from even larger and more diverse datasets. This will enable the algorithms to identify subtle patterns and correlations that can contribute to earlier and more accurate disease diagnoses.
Additionally, the integration of deep learning algorithms with other emerging technologies such as natural language processing and computer vision will further enhance their capabilities. By combining different modalities of medical data, including imaging, genomics, and clinical records, deep learning will be able to provide a more comprehensive analysis and personalized predictions for patients.
Implications for Healthcare
The future of deep learning in diagnostics has significant implications for healthcare. With improved accuracy and efficiency in medical diagnostics, healthcare providers will be able to make more informed decisions, resulting in better patient outcomes. Early detection of diseases through deep learning algorithms can lead to timely intervention and treatment, potentially saving lives. Personalized treatment plans based on deep learning analysis will also become more commonplace, ensuring that patients receive individualized care tailored to their specific needs.
However, it is important to address the challenges associated with deep learning-based medical diagnostics. Standardization of guidelines for algorithm development and evaluation, regulatory compliance, and ethical considerations surrounding data privacy and algorithm transparency must be addressed to ensure responsible and ethical implementation. By doing so, we can maximize the potential of deep learning in medical diagnostics and pave the way for a future where accurate and personalized healthcare is accessible to all.
Advancements in Deep Learning | Implications for Healthcare |
---|---|
Improved hardware capabilities | More efficient and accurate analysis of medical data |
Refined deep learning techniques | Early and accurate disease diagnosis |
Integration with other technologies | Comprehensive analysis and personalized predictions |
Challenges: Standardization, regulations, ethics | Responsible and ethical implementation |
Improving Accuracy in Medical Diagnostics with Deep Learning
Deep learning algorithms have emerged as a powerful tool in improving accuracy in medical diagnostics. By leveraging large amounts of medical data, these algorithms can detect intricate patterns and variations that may go unnoticed by human observers. This enhanced capability leads to more precise and reliable diagnoses, reducing the occurrence of false positives and false negatives. Furthermore, deep learning techniques enable the integration of multiple data sources, combining imaging and clinical data to provide a comprehensive analysis and more accurate predictions.
One of the key advantages of deep learning in medical diagnostics is its ability to continuously learn and adapt. These algorithms can be trained on vast datasets, enabling them to refine their performance over time. By learning from a diverse range of cases, deep learning algorithms can identify subtle disease markers and improve their diagnostic accuracy. This iterative learning process ensures that the algorithms stay up to date with the latest medical knowledge and advancements.
Deep learning algorithms also have the potential to revolutionize the field of radiology. By analyzing medical images such as X-rays, CT scans, and MRIs, these algorithms can assist radiologists in detecting and classifying abnormalities with greater accuracy. This not only speeds up the diagnostic process but also enhances overall patient care by enabling earlier detection of diseases such as cancer. In addition, deep learning algorithms enable precise segmentation of structures and organs within medical images, providing vital information for targeted treatment planning.
Table: Comparative Analysis of Deep Learning Algorithms in Medical Diagnostics
Algorithm | Accuracy | Speed | Applications |
---|---|---|---|
Convolutional Neural Networks (CNNs) | High | Fast | Medical imaging, disease detection |
Recurrent Neural Networks (RNNs) | Medium | Variable | Time series data analysis, disease prognosis |
Generative Adversarial Networks (GANs) | Medium | Variable | Medical image generation, anomaly detection |
As deep learning methodologies continue to advance and evolve, we can expect further improvements in the accuracy of medical diagnostics. With ongoing research and development, these algorithms have the potential to revolutionize healthcare by providing more precise and personalized treatment plans. However, it is essential to address challenges such as standardization, regulatory approval, and ethical considerations to ensure the responsible and ethical deployment of deep learning in medical diagnostics.
Advancements in Medical Diagnostics through Deep Learning
Deep learning has revolutionized medical diagnostics, contributing to significant advancements in the field. By leveraging deep learning algorithms, healthcare professionals can achieve more accurate and efficient diagnoses across various specialties. Deep learning applications have shown impressive results in ophthalmology, respiratory medicine, and breast imaging.
Deep Learning in Ophthalmology
In ophthalmology, deep learning algorithms have demonstrated high accuracy in diagnosing conditions such as diabetic retinopathy, age-related macular degeneration, and glaucoma. By analyzing retinal fundus photographs and optical coherence tomography scans, deep learning enables early detection and timely intervention, improving patient outcomes.
Deep Learning in Respiratory Medicine
Deep learning algorithms have also made significant strides in respiratory medicine. By analyzing chest X-rays and CT scans, these algorithms can detect lung nodules and identify lung cancer at early stages. This early detection can lead to more effective treatment strategies and improved survival rates for patients.
Deep Learning in Breast Imaging
Deep learning has enhanced the accuracy of breast cancer diagnosis on mammograms, ultrasounds, MRIs, and digital breast tomosynthesis. By analyzing these imaging modalities, deep learning algorithms can detect breast abnormalities, aiding in early detection and personalized treatment planning.
Specialty | Deep Learning Applications |
---|---|
Ophthalmology | Diabetic retinopathy, age-related macular degeneration, glaucoma |
Respiratory Medicine | Lung nodules, lung cancer |
Breast Imaging | Breast cancer detection and diagnosis |
These advancements in medical diagnostics through deep learning hold immense potential for improving patient care. By leveraging the power of deep learning algorithms, healthcare professionals can achieve earlier and more accurate diagnoses, leading to better treatment outcomes and ultimately, saving lives.
AI-Specific Challenges in Deep Learning-based Medical Diagnostics
As we explore the role of deep learning in medical diagnostics, it is important to acknowledge the challenges that come with its implementation. One of the main challenges is the lack of standardized guidelines for developing and evaluating deep learning algorithms in healthcare. The use of diverse datasets, variation in methodology, and heterogeneity in reporting can impact the generalizability and reproducibility of research findings. It is crucial to establish clear guidelines to ensure the accuracy and reliability of deep learning-based diagnostic tools.
Another challenge is the integration of deep learning algorithms into existing clinical workflows. This poses logistical and ethical considerations that need to be addressed. Regulatory approval, data privacy, and algorithm explainability are important factors to consider when implementing deep learning in medical diagnostics. Ensuring patient privacy and data security, as well as transparency in decision-making processes, are crucial for responsible and ethical practices.
Furthermore, deep learning algorithms heavily rely on large datasets for training and validation. While the availability of big data is increasing, there is still a need for more standardized and comprehensive datasets in medical diagnostics. Access to reliable and diverse datasets is essential to train accurate and robust deep learning models.
Table: Challenges in Deep Learning-based Medical Diagnostics
Challenges | Solutions |
---|---|
Lack of standardized guidelines | Establish clear guidelines for developing and evaluating deep learning algorithms in healthcare. |
Integration into clinical workflows | Address logistical and ethical considerations, including regulatory approval, data privacy, and algorithm explainability. |
Reliance on large datasets | Ensure access to reliable and diverse datasets for training and validation. |
Overcoming these challenges will be crucial to harness the full potential of deep learning in medical diagnostics. Collaboration between researchers, healthcare professionals, and regulatory bodies is essential to address these challenges and pave the way for the successful implementation of deep learning-based diagnostic tools. With continued advancements and concerted efforts, we can leverage the power of artificial intelligence to revolutionize medical diagnostics and improve patient outcomes.
Deep Learning and Personalized Healthcare
The advancements in deep learning have opened up exciting possibilities for personalized healthcare. By analyzing vast amounts of patient data, including medical images, genomic information, and clinical records, deep learning algorithms can generate personalized insights and predictions that can greatly impact patient care. With this technology, healthcare providers can develop tailored treatment plans, detect diseases at earlier stages, and effectively manage chronic conditions.
Deep learning algorithms excel at extracting complex patterns and features from diverse datasets, enabling the identification of subtle variations that may be missed by human observers. By integrating different types of data, such as imaging and clinical records, deep learning algorithms can provide comprehensive analyses, leading to more accurate predictions and personalized treatment recommendations. This approach allows healthcare providers to take a proactive stance in patient care, identifying high-risk individuals and intervening at an early stage to prevent or manage disease progression.
Additionally, deep learning algorithms have the potential to improve patient outcomes by enhancing treatment decision-making. By analyzing a patient's unique characteristics and medical history, these algorithms can assist healthcare providers in selecting the most appropriate treatment options. This personalized approach to healthcare not only improves patient satisfaction but also increases treatment efficacy and reduces healthcare costs.
Examples of Deep Learning in Personalized Healthcare
To illustrate the impact of deep learning in personalized healthcare, here are a few notable examples:
- Cancer Diagnosis: Deep learning algorithms have been successfully applied to analyze medical images and detect various types of cancer, such as breast, lung, and skin cancer. These algorithms can assist in early detection and accurate diagnosis, leading to more targeted and effective treatment plans.
- Genomic Analysis: Deep learning techniques can analyze genomic information and identify genetic markers associated with specific diseases. This information can be used to assess an individual's risk of developing certain conditions and guide preventive measures.
- Predictive Analytics: Deep learning algorithms can analyze patient data to predict disease progression and treatment response. This information can help healthcare providers optimize treatment plans and monitor patients more effectively.
As deep learning continues to advance, the possibilities for personalized healthcare are continuously expanding. However, it is important to address ethical considerations, such as patient privacy and algorithm explainability, to ensure responsible and ethical implementation of deep learning in medical diagnostics.
Advantages of Deep Learning in Personalized Healthcare | Challenges for Implementation |
---|---|
|
|
Ethical and Legal Considerations in Deep Learning-based Medical Diagnostics
As we delve deeper into the realm of deep learning-based medical diagnostics, it is crucial to address the ethical and legal considerations associated with this innovative technology. One of the foremost concerns is patient privacy and data security. With deep learning algorithms requiring access to sensitive medical information, it is imperative that proper consent is obtained and robust data protection measures are in place to safeguard patient confidentiality. We must ensure that patient data is handled responsibly and with the utmost care to maintain trust and ethical standards.
Transparency and explainability are also key ethical considerations in deep learning-based medical diagnostics. Healthcare providers and patients need to understand how decisions are being made by these algorithms. Transparent implementation allows for greater trust and accountability, ensuring that diagnoses and treatment plans are reliable and valid. Furthermore, explainability enables healthcare professionals to explain the reasoning behind the algorithms' decisions, enhancing communication and fostering patient confidence in the diagnostic process.
Addressing Bias and Fairness
Another ethical consideration is the need to address bias and ensure fairness in deep learning-based medical diagnostics. These algorithms learn from existing datasets, which may contain inherent biases due to demographic, socioeconomic, or regional factors. As a result, the algorithms may inadvertently perpetuate these biases, leading to disparities in diagnosis or treatment. It is essential to actively mitigate bias in the development and evaluation of deep learning algorithms to ensure fair and equitable healthcare outcomes for all patients.
From a legal standpoint, the implementation of deep learning in medical diagnostics must adhere to existing regulations and guidelines. The use of deep learning algorithms in clinical practice requires regulatory approval to ensure patient safety and efficacy. Additionally, considerations such as intellectual property rights, liability, and accountability need to be addressed in the context of deep learning-based medical diagnostics. Legal frameworks should be in place to govern the responsible use and implementation of this technology, protecting the rights and interests of both patients and healthcare providers.
Ethical Considerations in Deep Learning-based Medical Diagnostics | Legal Considerations in Deep Learning-based Medical Diagnostics |
---|---|
|
|
Conclusion
In conclusion, deep learning has revolutionized the field of medical diagnostics, offering advanced solutions for disease diagnosis, treatment, and patient care. With its sophisticated algorithms and ability to analyze complex medical data, deep learning has the potential to greatly improve the accuracy and efficiency of medical diagnostics.
By utilizing deep learning techniques, healthcare providers can benefit from enhanced diagnostic processes, leading to more accurate and timely disease diagnosis. Deep learning algorithms can analyze large amounts of medical data with high precision, reducing human error and improving diagnostic accuracy. This enables faster processing of patient information and quicker diagnosis, ultimately improving patient outcomes.
The future of deep learning in medical diagnostics holds tremendous promise. As technology continues to advance and deep learning algorithms become more sophisticated, we can expect even more efficient diagnostic tools and systems. With the increasing availability of large medical datasets and advancements in hardware, deep learning-based diagnostic solutions can be developed and deployed on a larger scale.
Despite the potential benefits, there are challenges to address, such as standardization, regulatory approval, and ethical considerations. Standardized guidelines for the development and evaluation of deep learning algorithms in healthcare are necessary to ensure generalizability and reproducibility of research findings. Additionally, ethical principles such as transparency, accountability, and patient privacy need to be upheld throughout the implementation of deep learning in medical diagnostics.