Exploring the Potential of Mobile Health for Rapid Diagnostic Test Analysis
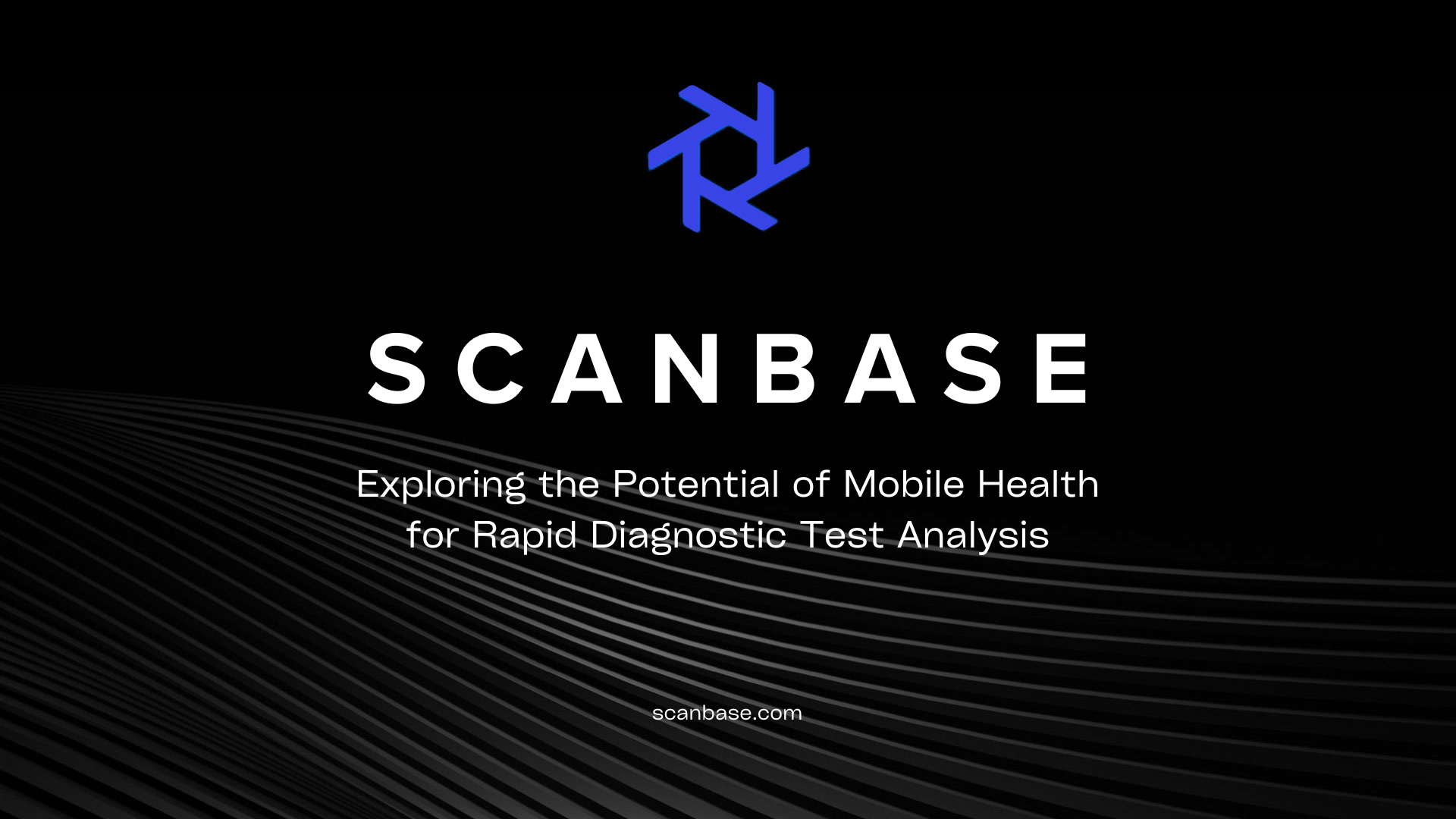
Section 1: Introduction to Mobile Health and Rapid Diagnostic Tests
In recent years, mobile health (mHealth) has emerged as a promising field that utilizes mobile devices, such as smartphones and tablets, to deliver healthcare services and information remotely. With the advancement of technology, mHealth has the potential to revolutionize the way rapid diagnostic tests (RDTs) are conducted and analyzed. In this section, we will explore the concept of mobile health, the significance of rapid diagnostic tests, and the need for efficient RDT analysis.
1.1 What is Mobile Health?
Mobile health, also known as mHealth, refers to the use of mobile devices and wireless technologies in healthcare delivery. It encompasses a wide range of applications, including health education, remote patient monitoring, disease surveillance, and diagnostic support. By leveraging the ubiquity of mobile devices, mHealth aims to improve access to healthcare services, enhance health outcomes, and empower individuals to take control of their own health.
Mobile health technologies can include mobile apps, wearable devices, sensors, and other portable devices that enable the collection, transmission, and analysis of health-related data. These technologies have the potential to bridge the gap between healthcare providers and patients, enabling remote consultations, real-time monitoring, and timely interventions.
1.2 What are Rapid Diagnostic Tests?
Rapid diagnostic tests (RDTs) are point-of-care tests designed to provide quick and accurate results for the diagnosis of various medical conditions. Unlike traditional laboratory-based tests that require specialized equipment and trained personnel, RDTs are often simple, easy to use, and deliver results within minutes. They are particularly valuable in resource-limited settings, where access to traditional laboratory facilities may be limited.
RDTs are available for a wide range of diseases, including infectious diseases such as malaria, HIV, tuberculosis, and COVID-19. These tests typically involve the detection of specific biomarkers, such as antigens or antibodies, in a patient's blood, urine, or saliva sample. The simplicity and portability of RDTs make them suitable for use in a variety of healthcare settings, including clinics, community health centers, and even remote areas where access to healthcare infrastructure is limited.
1.3 The Need for Rapid Diagnostic Test Analysis
Efficient and accurate analysis of RDT results is crucial for timely diagnosis, treatment, and disease surveillance. Traditionally, RDT analysis has been performed manually by visual interpretation of test lines, which is subjective and prone to human error. Moreover, the interpretation of RDT results can be challenging for healthcare personnel with limited training or in complex cases where multiple lines need to be analyzed.
Mobile health has the potential to address these challenges and improve the analysis of RDT results. By leveraging mobile devices, advanced imaging technologies, and artificial intelligence (AI) algorithms, mHealth can enable automated and objective interpretation of RDT results. This would not only minimize errors but also enhance the accuracy and reliability of diagnosis, especially in resource-constrained settings.
In the next section, we will delve into the various mobile health applications and technologies that can be used for rapid diagnostic test analysis. We will explore the benefits and challenges associated with the implementation of mHealth in this context, and discuss real-life case studies that demonstrate the potential of mobile health in RDT analysis.
Section 2: Mobile Health Applications for Rapid Diagnostic Test Analysis
Mobile health (mHealth) offers a wide range of applications and technologies that can revolutionize the analysis of rapid diagnostic tests (RDTs). In this section, we will explore the various mHealth applications and technologies used for RDT analysis, discussing their benefits and limitations.
2.1 Mobile Health Technologies for Rapid Diagnostic Test Analysis
2.1.1 Smartphone-Based Imaging
One of the key applications of mobile health in RDT analysis is smartphone-based imaging. By utilizing the high-resolution cameras present in modern smartphones, healthcare providers can capture images of RDTs and analyze them using specialized apps or software. These apps employ computer vision algorithms to interpret the visual signals from the RDT and provide objective and accurate results.
Smartphone-based imaging offers several advantages in RDT analysis. Firstly, it eliminates the subjectivity associated with manual interpretation, ensuring consistent and reliable results. Secondly, it enables remote analysis, allowing healthcare providers to transmit RDT images to experts for consultation or quality control purposes. Lastly, smartphone-based imaging can be integrated with electronic health record systems, ensuring seamless data management and traceability.
2.1.2 Portable Spectrophotometers
Another mobile health technology that shows promise in RDT analysis is portable spectrophotometers. These handheld devices utilize optical techniques to measure the intensity of specific wavelengths of light emitted or absorbed by the RDT. The device then quantifies the results and provides objective measurements, eliminating the need for visual interpretation.
Portable spectrophotometers offer several advantages in RDT analysis. They provide quantitative measurements, allowing for more precise analysis and monitoring of disease progression. Additionally, these devices are compact, lightweight, and easy to use, making them suitable for point-of-care settings. However, their cost and availability may limit their widespread adoption in resource-constrained areas.
2.2 Benefits of Using Mobile Health for Rapid Diagnostic Test Analysis
The integration of mobile health technologies in RDT analysis brings numerous benefits to healthcare providers, patients, and public health systems.
2.2.1 Improved Accuracy and Reliability
Mobile health technologies offer automated and objective analysis of RDT results, minimizing errors associated with manual interpretation. This leads to improved accuracy and reliability in diagnosing various medical conditions. The elimination of subjectivity ensures consistent results, especially in cases where multiple lines need to be analyzed.
2.2.2 Enhanced Access to Healthcare
By utilizing mobile devices, mHealth enables rapid diagnostic test analysis in remote and underserved areas. This improves access to healthcare services and contributes to early detection and treatment of diseases. Patients in remote locations can receive timely diagnosis and appropriate care, reducing the burden on healthcare systems and improving health outcomes.
2.2.3 Real-time Data Transmission and Analysis
Mobile health technologies facilitate real-time transmission of RDT results to healthcare providers and experts. This enables remote consultation, quality control, and data analysis. Healthcare providers can seek expert opinions and guidance, enhancing their decision-making process. Public health systems can also benefit from real-time data transmission, allowing for improved disease surveillance and response strategies.
2.3 Challenges and Limitations of Mobile Health for Rapid Diagnostic Test Analysis
While mobile health shows great potential in RDT analysis, there are several challenges and limitations that need to be addressed for successful implementation.
2.3.1 Technical Infrastructure and Connectivity
The effectiveness of mobile health technologies relies on a robust technical infrastructure and reliable connectivity. In resource-constrained areas with limited internet access or unstable networks, the seamless transmission and analysis of RDT results may be compromised. Efforts must be made to improve infrastructure and connectivity to ensure the widespread adoption of mobile health for RDT analysis.
2.3.2 Training and Education
Healthcare providers need proper training and education to effectively utilize mobile health technologies for RDT analysis. They must be familiar with the operation of the devices and software, understand the interpretation algorithms, and be aware of potential limitations or pitfalls. Continuous training programs and educational initiatives are essential to ensure the successful integration of mobile health in RDT analysis.
In the next section, we will delve into real-life case studies that highlight the application of mobile health in rapid diagnostic test analysis for diseases such as malaria, HIV, and COVID-19. We will examine the outcomes and challenges faced in these case studies, shedding light on the potential of mobile health in improving healthcare delivery.
Section 3: Case Studies of Mobile Health in Rapid Diagnostic Test Analysis
Real-life case studies demonstrate the potential of mobile health (mHealth) in revolutionizing rapid diagnostic test (RDT) analysis. In this section, we will explore three case studies that highlight the application of mobile health in the analysis of RDTs for diseases such as malaria, HIV, and COVID-19. These case studies provide valuable insights into the benefits and challenges of using mHealth for RDT analysis.
3.1 Case Study 1: Mobile Health for Malaria Rapid Diagnostic Test Analysis
Malaria continues to be a major global health concern, particularly in regions with limited access to healthcare infrastructure. Mobile health technologies have been employed to improve the accuracy and efficiency of malaria RDT analysis.
In a study conducted in rural areas of sub-Saharan Africa, community health workers were trained to use smartphones equipped with specialized apps for malaria RDT analysis. These apps utilized image processing algorithms to analyze the RDT results captured by the smartphone camera. The results were then transmitted to healthcare experts for remote validation and consultation.
The use of mobile health technologies in malaria RDT analysis proved to be highly effective. The automated interpretation of RDT results using smartphone-based imaging reduced errors and improved the accuracy of diagnosis. The real-time transmission of results facilitated prompt treatment and enhanced disease surveillance. The study demonstrated the potential of mHealth in overcoming the challenges faced in diagnosing and managing malaria in resource-limited settings.
3.2 Case Study 2: Mobile Health for HIV Rapid Diagnostic Test Analysis
HIV is a global health crisis, requiring efficient and accurate diagnosis for effective management and treatment. Mobile health has emerged as a valuable tool in HIV rapid diagnostic test (HIV RDT) analysis, particularly in resource-limited settings with a high burden of HIV.
In a study conducted in rural areas of sub-Saharan Africa, community healthcare workers used mobile devices equipped with portable spectrophotometers to analyze HIV RDT results. The portable spectrophotometers measured the intensity of specific wavelengths of light emitted or absorbed by the RDT, providing objective measurements for diagnosis.
The integration of mobile health technologies in HIV RDT analysis yielded promising results. The use of portable spectrophotometers improved the accuracy and reliability of HIV diagnosis by providing quantitative measurements. The real-time transmission of results to healthcare experts enabled remote consultation and quality control. The study demonstrated the potential of mHealth in enhancing access to HIV testing and improving the accuracy of diagnosis, ultimately contributing to better patient outcomes.
3.3 Case Study 3: Mobile Health for COVID-19 Rapid Diagnostic Test Analysis
The COVID-19 pandemic highlighted the urgent need for rapid and accurate diagnostic testing. Mobile health technologies played a crucial role in the analysis of COVID-19 rapid diagnostic tests (COVID-19 RDTs) to facilitate timely diagnosis and effective containment strategies.
In a pilot program conducted in a densely populated urban area, healthcare providers used smartphone-based imaging to analyze COVID-19 RDTs. They captured images of the RDTs using smartphones equipped with specialized apps that employed AI algorithms for automated interpretation. The results were transmitted to a centralized system for analysis and reporting.
The use of mobile health technologies in COVID-19 RDT analysis proved to be highly beneficial. The automated analysis of RDT results using smartphone-based imaging reduced errors and improved efficiency. The real-time transmission of results enabled prompt reporting and facilitated contact tracing efforts. The study demonstrated the potential of mHealth in supporting rapid and accurate COVID-19 diagnosis, contributing to effective control and prevention strategies.
In the next section, we will explore future trends and innovations in mobile health for rapid diagnostic test analysis, including the integration of artificial intelligence, wearable devices, and the integration with electronic health records. These advancements have the potential to further enhance the capabilities of mHealth in RDT analysis and improve healthcare delivery.
Section 4: Future Trends and Innovations in Mobile Health for Rapid Diagnostic Test Analysis
The field of mobile health (mHealth) for rapid diagnostic test (RDT) analysis continues to evolve with technological advancements and innovative approaches. In this section, we will explore several future trends and innovations that have the potential to revolutionize the capabilities of mHealth in RDT analysis.
4.1 Artificial Intelligence and Machine Learning in Rapid Diagnostic Test Analysis
Artificial intelligence (AI) and machine learning (ML) have the potential to significantly enhance the accuracy and efficiency of RDT analysis. By training AI algorithms on large datasets of RDT results, these technologies can learn to detect subtle patterns and variations that may not be easily discernible to the human eye.
AI and ML algorithms can automate the interpretation of RDT results, improving the speed and consistency of analysis. They can also assist in the detection of complex or rare conditions by identifying specific patterns or biomarkers. Furthermore, AI algorithms can continuously learn and adapt, improving their performance over time.
The integration of AI and ML in mHealth for RDT analysis holds immense promise. It can lead to faster and more accurate diagnoses, especially in cases where multiple lines or complex patterns need to be analyzed. Additionally, AI and ML can enhance disease surveillance capabilities by detecting trends and patterns in RDT data, contributing to early detection and rapid response strategies.
4.2 Integration of Mobile Health with Electronic Health Records
The integration of mobile health technologies with electronic health records (EHRs) can revolutionize the way RDT results are managed, analyzed, and shared. By seamlessly integrating RDT data into existing EHR systems, healthcare providers can access a comprehensive view of a patient's health information, including their RDT history.
This integration enables improved continuity of care, as healthcare providers can access an individual's complete medical history, including their RDT results, in real-time. It also facilitates data analysis and population health management by providing a wealth of aggregated RDT data for research and surveillance purposes.
The integration of mobile health with EHRs also enhances data security and privacy. By leveraging existing EHR infrastructure, the transmission and storage of RDT data can adhere to established security protocols and regulations, ensuring the confidentiality of patient information.
4.3 Wearable Devices and Sensors for Rapid Diagnostic Test Analysis
Wearable devices and sensors offer exciting possibilities in the field of mHealth for RDT analysis. These devices can continuously monitor various physiological parameters, such as heart rate, temperature, and respiratory rate, providing valuable insights into an individual's health status.
By incorporating RDT analysis capabilities into wearable devices and sensors, real-time monitoring and analysis of RDT results can be achieved. For example, wearable devices equipped with sensors could detect and measure specific biomarkers associated with RDT analysis, providing instant feedback on the presence or progression of a disease.
The integration of wearable devices and sensors with mHealth platforms can enable remote monitoring and personalized healthcare. Patients can perform RDTs at home and transmit the results to healthcare providers for analysis and consultation. This approach enhances convenience, reduces the need for physical clinic visits, and allows for early intervention in case of abnormal RDT results.
In conclusion, future trends and innovations in mHealth for RDT analysis, such as AI and ML integration, the integration with EHRs, and the use of wearable devices and sensors, hold great potential to advance the field. These advancements can lead to improved accuracy, efficiency, and accessibility in RDT analysis, ultimately enhancing healthcare delivery and improving patient outcomes.
In the final section, we will summarize the key points discussed throughout this blog post and provide a concluding remark on the potential of mobile health in rapid diagnostic test analysis.
Section 5: Conclusion
In this comprehensive blog post, we have explored the potential of mobile health (mHealth) in revolutionizing the analysis of rapid diagnostic tests (RDTs). We began by introducing the concept of mHealth and the significance of RDTs in healthcare. We then delved into the various applications and technologies of mHealth for RDT analysis, discussing the benefits and limitations of each.
Mobile health technologies, such as smartphone-based imaging and portable spectrophotometers, offer automated and objective analysis of RDT results, improving accuracy and reliability. These technologies enable remote analysis, real-time data transmission, and integration with electronic health record systems, enhancing access to healthcare and facilitating data management.
Through real-life case studies, we witnessed the successful application of mHealth in RDT analysis for diseases like malaria, HIV, and COVID-19. These case studies demonstrated the effectiveness of mobile health technologies in improving diagnosis accuracy, enhancing access to testing, and supporting disease surveillance efforts.
Looking towards the future, we explored the potential of artificial intelligence and machine learning in RDT analysis. These technologies can enhance the speed and accuracy of analysis, contributing to faster and more precise diagnoses. We also discussed the integration of mHealth with electronic health records, enabling seamless data management and improved continuity of care. Additionally, the use of wearable devices and sensors offers the possibility of real-time monitoring and personalized healthcare.
In conclusion, the potential of mobile health for rapid diagnostic test analysis is vast. By leveraging mHealth technologies, healthcare providers can overcome the limitations of traditional manual interpretation, improve accuracy and efficiency, and extend healthcare services to remote and underserved areas. The integration of artificial intelligence, electronic health records, and wearable devices will further enhance the capabilities of mHealth in RDT analysis, shaping the future of healthcare delivery.
As technology continues to advance and healthcare systems evolve, the potential for mobile health in rapid diagnostic test analysis will only grow. It is an exciting field that holds promise for improving healthcare outcomes and transforming the way we approach diagnostics. By embracing and exploring the potential of mobile health, we can pave the way for a future where rapid diagnostic test analysis is more accurate, accessible, and efficient than ever before.