Exploring Challenges and Opportunities in Smartphone-based Rapid Diagnostic Test Interpretation
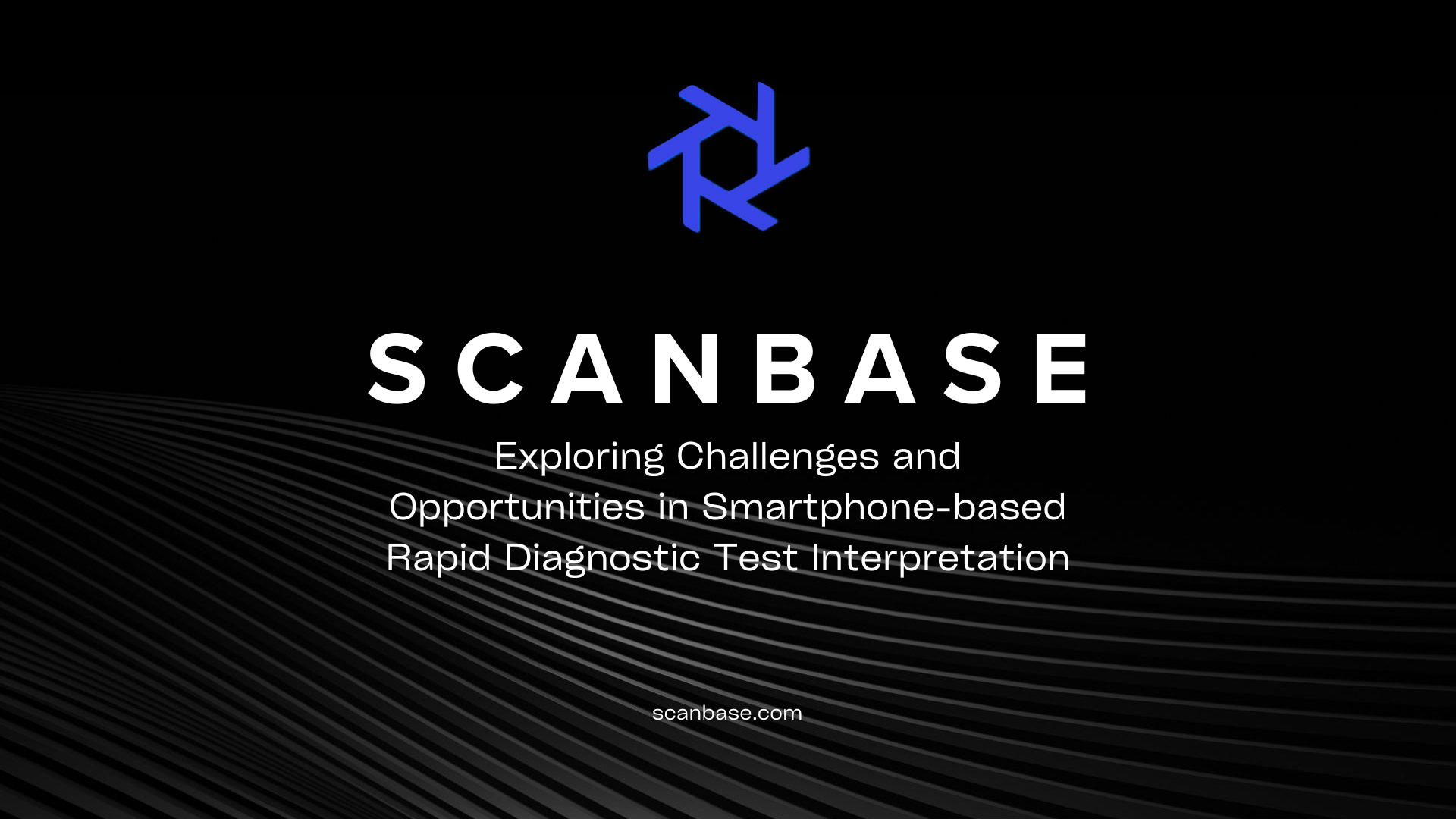
In recent years, there has been much excitement around the potential of smartphone-based rapid diagnostic tests (RDTs) to revolutionize healthcare. These tests, which can be administered and interpreted using a smartphone, have the potential to improve access to diagnostic testing, particularly in low-resource settings, and enhance the speed and accuracy of disease diagnoses.
However, the emerging field of smartphone-based RDT interpretation is not without its challenges. Issues such as image quality, user error, and the need for standardization in interpreting RDT results must be addressed to ensure the technology's effectiveness and reliability.
Despite these challenges, there are also many exciting opportunities associated with smartphone-based RDT interpretation. Artificial intelligence (AI) and machine learning algorithms can be utilized to accurately and efficiently interpret RDT results, while natural language processing (NLP) techniques can assist in extracting relevant information from textual components of RDTs. Additionally, the integration of smartphone-based RDT interpretation into mobile health and digital health initiatives has the potential to greatly enhance healthcare delivery and empower individuals to manage their health more effectively.
Key Takeaways:
- Smartphone-based RDT interpretation has the potential to revolutionize healthcare.
- Challenges such as image quality, user error, and the need for standardization must be addressed.
- AI, machine learning, and NLP techniques can aid in accurate and efficient RDT interpretation.
- The integration of smartphone-based RDT interpretation into mobile health and digital health initiatives has many exciting possibilities.
Understanding Smartphone-based Rapid Diagnostic Tests (RDTs)
Smartphone-based rapid diagnostic tests (RDTs) are a type of digital health technology that allows individuals to test for certain medical conditions using their smartphones. RDTs are designed to be easy to use and interpret, making them ideal for use in mobile health (mHealth) settings. The tests are typically designed to be administered at home, without the need for a healthcare professional.
The digital health industry has seen significant growth in recent years, with smartphone-based RDTs being one of the most promising developments. This technology has the potential to revolutionize healthcare delivery, especially in areas where access to medical services is limited. The ease of use and accessibility offered by smartphone-based RDTs make them an attractive option for individuals who might otherwise struggle to access diagnostic tests.
The Role of Artificial Intelligence in RDT Interpretation
Artificial intelligence (AI) has emerged as a promising technology for improving the accuracy and efficiency of RDT interpretation. Machine learning algorithms, a subset of AI, can quickly analyze large amounts of data and identify patterns that can be used to interpret RDT results.
One of the major advantages of using AI in RDT interpretation is its ability to detect subtle differences in test results that might be missed by the human eye. This can lead to more accurate diagnosis and treatment.
However, the use of AI in RDT interpretation is not without its challenges. One of the primary issues is the lack of standardization in RDTs, which can make it difficult to train algorithms to recognize different types of tests. Additionally, data privacy concerns and the need for regulatory oversight must be addressed before AI can be widely used in healthcare.
Despite these challenges, the use of AI in RDT interpretation is expected to grow in the coming years. Ongoing research is focused on developing better algorithms and improving the accuracy of AI-based RDT interpretation. As healthcare technology continues to evolve, AI is poised to play an increasingly important role in improving the accuracy and efficiency of RDT interpretation.
Image Analysis Techniques for Smartphone-based RDT Interpretation
One of the key components of smartphone-based RDT interpretation is image analysis. This involves using healthcare technology to analyze the images captured by the smartphone camera and extract relevant diagnostic information. There are several different image analysis techniques used in RDT interpretation, ranging from simple color thresholding to more complex machine learning algorithms.
Color thresholding involves setting a threshold value for the intensity of each color channel in the image. If the intensity of a particular channel is above the threshold, it is considered a positive result for that color. This technique is relatively simple and can be effective for RDTs with only a few color channels. However, it may not work as well for more complex RDTs or those with overlapping color channels.
Machine learning algorithms can also be used for RDT interpretation. These algorithms can learn to recognize patterns in RDT images and make accurate diagnoses based on those patterns. However, machine learning algorithms require training data to learn from, and developing accurate and representative training datasets can be a challenge. Additionally, these algorithms may not be as interpretable as simpler techniques like color thresholding, which can make it difficult to understand how they are making their diagnoses.
Overall, image analysis techniques are a critical component of smartphone-based RDT interpretation, providing a way to extract diagnostic information from the images captured by the smartphone camera. As healthcare technology continues to advance, it is likely that more sophisticated image analysis techniques will be developed, further enhancing the accuracy and efficiency of smartphone-based RDT interpretation.
Challenges in Smartphone-based RDT Interpretation
The use of smartphone-based RDT interpretation presents several challenges. One of the primary issues is the quality of the images captured by the smartphone camera. If the image is blurry or low resolution, it can be difficult to accurately interpret the results. Additionally, user error can occur when capturing the image or interpreting the results, which can lead to incorrect diagnoses.
Another challenge is the need for standardization in interpreting RDT results. Without clear guidelines for interpretation, there may be inconsistencies in the way results are read, leading to disparities in diagnoses. Standardization is particularly important when using smartphone-based RDTs in remote or low-resource settings.
Overall, while smartphone-based RDT interpretation has the potential to revolutionize healthcare diagnostics, there are challenges that must be overcome to ensure accurate and reliable results.
Opportunities in Smartphone-based RDT Interpretation
Smartphone-based RDT interpretation presents a range of exciting opportunities in the field of digital health. With the potential to improve access to diagnostic testing, this technology has the power to enhance healthcare delivery and empower individuals to take control of their health.
One significant opportunity afforded by smartphone-based RDT interpretation is its ability to overcome geographical barriers to healthcare. With the increasing availability of affordable smartphones, individuals in remote or underserved areas can benefit from accurate and timely diagnostic testing. This is particularly important for diseases that require urgent treatment, such as malaria or HIV.
In addition to providing access to testing, smartphone-based RDT interpretation can also enable self-testing and self-monitoring, empowering individuals to take charge of their health. This can be particularly impactful for those with chronic illnesses, who require regular monitoring and may struggle to access traditional healthcare services.
The integration of smartphone-based RDT interpretation into healthcare systems also has the potential to improve overall healthcare delivery. By reducing the need for laboratory testing and streamlining diagnostic processes, healthcare professionals can provide faster and more accurate diagnoses.
Furthermore, smartphone-based RDT interpretation can enable real-time data collection and analysis, allowing for more effective disease surveillance and outbreak response. This can be particularly important in the context of infectious diseases, where rapid detection is crucial for preventing the spread of disease.
Overall, smartphone-based RDT interpretation presents a range of exciting opportunities for digital health. By improving access to diagnostic testing, empowering individuals, and streamlining healthcare processes, this technology has the potential to revolutionize healthcare delivery and improve health outcomes.
NLP and its Role in Smartphone-based RDT Interpretation
Natural Language Processing (NLP) is a branch of artificial intelligence (AI) that focuses on the interaction between computers and humans using natural language. In the context of smartphone-based RDT interpretation, NLP techniques can assist in extracting relevant information from the textual components of RDTs, such as the patient's name and date of birth, as well as any other pertinent details.
In addition to extracting textual information, NLP can also aid in the interpretation of RDT results by analyzing the language used in accompanying instructions or explanations. For example, NLP algorithms can be trained to identify keywords and phrases that indicate a positive or negative test result, or to detect any potential errors in the interpretation process.
The use of NLP in smartphone-based RDT interpretation has the potential to improve the accuracy and efficiency of the diagnostic process, as well as to facilitate the integration of RDTs into digital health platforms. By automating aspects of RDT interpretation that were previously reliant on human interpretation, NLP can help to reduce the risk of errors and inconsistencies, while also enabling faster and more widespread access to diagnostic testing.
The Future of Smartphone-based RDT Interpretation
As technology advances and healthcare evolves, the future of smartphone-based RDT interpretation looks promising. With the increasing use of digital health and mobile health initiatives, smartphone-based RDTs have the potential to revolutionize the way diagnostic testing is performed.
One area where significant progress is being made is in the use of artificial intelligence and machine learning algorithms. As these technologies continue to improve, they will enable faster and more accurate interpretation of RDT results, improving healthcare delivery and patient outcomes.
In addition, ongoing research is exploring new image analysis techniques and approaches for interpreting RDT images. This will enhance the reliability and accuracy of RDT interpretation, enabling more widespread adoption of this technology in healthcare settings.
The future of smartphone-based RDT interpretation also holds promise for improved accessibility and convenience for patients. With the ability to perform diagnostic testing on a smartphone, individuals will have greater control over their healthcare, and access to testing will be more widespread, particularly in remote or underserved areas.
As with any emerging technology, there are still challenges to be addressed. The standardization of RDT interpretation and the need for high-quality images remain significant hurdles. However, it is clear that smartphone-based RDT interpretation has the potential to revolutionize healthcare and improve patient outcomes in the years to come.
Conclusion
In conclusion, smartphone-based rapid diagnostic test (RDT) interpretation presents both challenges and opportunities for healthcare technology. While there are challenges such as image quality and user error, the potential benefits of this technology are vast.
Smartphone-based RDT interpretation has the potential to improve access to diagnostic testing, enhance healthcare delivery, and empower individuals to manage their health effectively. Artificial intelligence, particularly machine learning algorithms, and image analysis techniques hold significant promise in accurately and efficiently interpreting RDT results.
Furthermore, natural language processing (NLP) techniques can assist in extracting relevant information from textual components of RDTs. As ongoing research and potential advancements continue to shape the field, we can expect to see further opportunities for smartphone-based RDT interpretation.
Overall, the challenges and opportunities in smartphone-based RDT interpretation showcase the potential impact this technology can have on healthcare diagnostics, providing a more accessible and efficient way to diagnose and treat illnesses.