Explore Multi-Modal Fusion Techniques for Smartphone-based Rapid Diagnostic Test Analysis
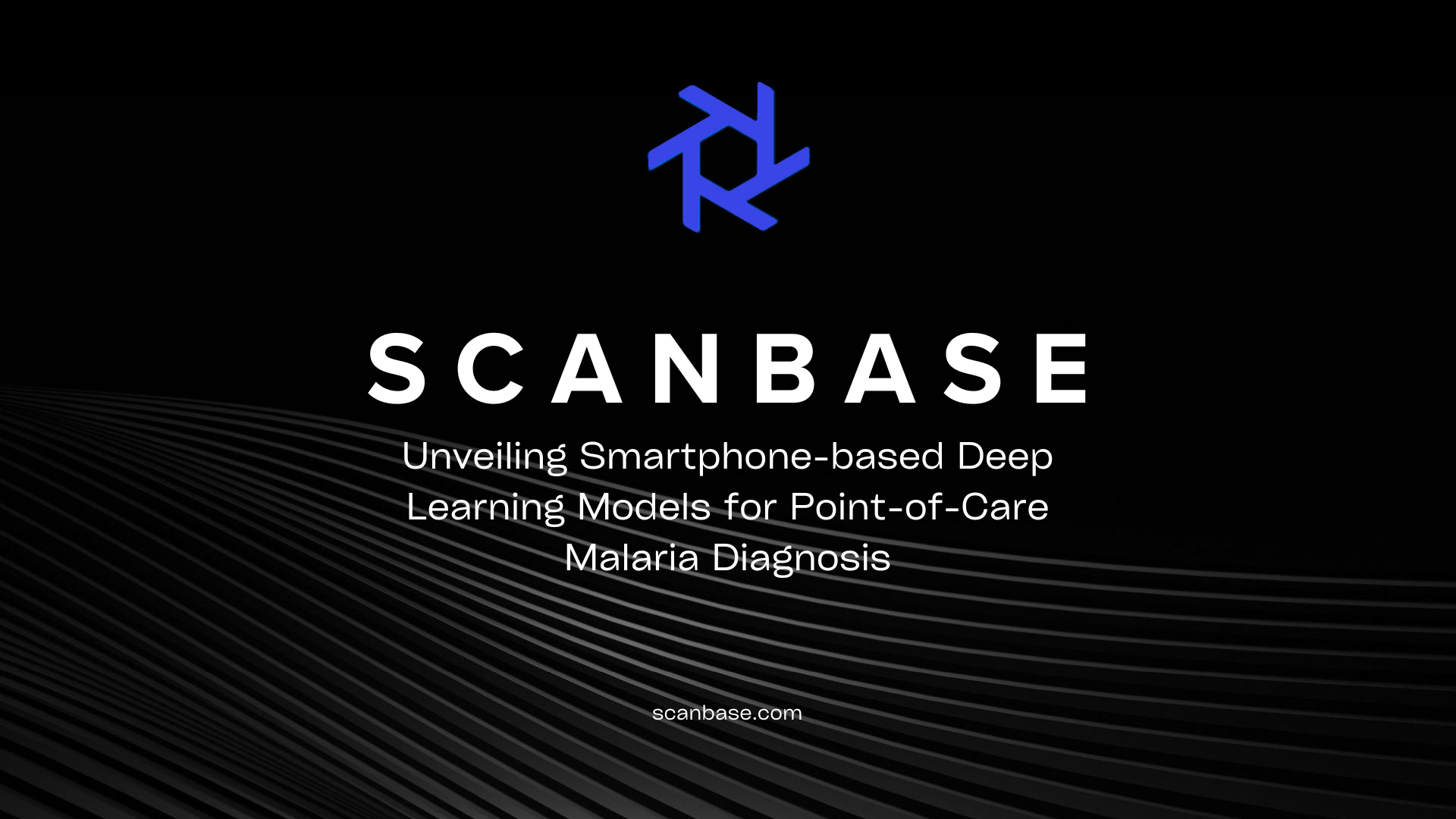
Rapid diagnostic tests (RDTs) have revolutionized disease diagnosis, providing a quick and cost-effective solution for healthcare providers. However, traditional RDT analysis methods are limited in their ability to provide comprehensive results. Multi-modal fusion techniques offer a promising solution to this challenge, providing a more comprehensive analysis of RDT data. By combining different types of data, such as imaging and sensor data, through smartphones, multi-modal fusion techniques can significantly improve the accuracy and reliability of RDT analysis.
Key Takeaways:
- Multi-Modal Fusion Techniques allow for more comprehensive analysis of Rapid Diagnostic Tests
- Smartphones can be used for Comprehensive Smartphone-based RDT Analysis
Understanding Smartphone-based RDTs
Smartphone-based rapid diagnostic tests (RDTs) are a type of diagnostic technology designed to quickly and conveniently perform medical tests on smartphones. These tests use a combination of sensors and software to analyze biological samples, such as blood or urine, and provide diagnostic results in real-time.
Comprehensive analysis is critical in the field of diagnostic testing, and smartphone-based RDTs offer a promising platform for achieving it. With the ability to integrate multiple data modalities, including visual, textual, and sensor data, these tests can provide an accurate and holistic analysis of diagnostic results.
Introduction to Multi-Modal Fusion Techniques
Multi-modal fusion techniques are a powerful approach to analyzing rapid diagnostic tests (RDTs) using smartphones. The integration of multiple modalities, such as visual, audio, and textual data, can provide a more comprehensive analysis of RDTs, leading to greater accuracy and reliability of diagnostic results.
A key advantage of multi-modal fusion techniques is their ability to combine data from different sources to produce a more comprehensive analysis. By integrating multiple modalities, fusion techniques can provide a more accurate diagnosis than relying on a single modality alone. This approach can help reduce false positives and false negatives, resulting in more accurate and reliable diagnoses.
The use of multi-modal fusion techniques in smartphone-based RDT analysis has significant potential in healthcare and medical research. It can provide a quick and affordable way to diagnose diseases in settings with limited resources, such as remote or low-income areas. In addition, the technology can support personalized medicine, allowing for tailored treatment plans based on an individual's unique needs.
Overall, the integration of multi-modal fusion techniques in smartphone-based RDT analysis represents a promising step toward achieving comprehensive and accurate diagnostic results.
Leveraging Natural Language Processing (NLP)
Multi-modal fusion techniques are not limited to image and sensor data integration. Natural language processing (NLP) algorithms can also contribute to comprehensive analysis by extracting valuable information from textual data.
In smartphone-based RDT analysis, NLP can be used to analyze patient history, symptoms, and other relevant medical records. This information can augment image and sensor data, providing a more complete view of the patient’s health status.
NLP can also aid in the interpretation of test results by extracting relevant information from diagnostic reports and medical literature. This can improve the accuracy and precision of smartphone-based RDT analysis, contributing to more personalized and effective healthcare.
The integration of NLP with other modalities in multi-modal fusion techniques can enable a more comprehensive analysis of rapid diagnostic tests, taking into consideration both patient-related information, as well as quantitative measurements.
However, NLP usage in comprehensive analysis through multi-modal fusion techniques faces challenges, including the variability and complexity of textual data, the need for sophisticated algorithms, and the processing power of smartphones. As research progresses, these challenges are becoming less significant, and NLP is increasingly becoming an integral part of multi-modal fusion techniques for smartphone-based RDT analysis.
Integration of Imaging and Sensor Data
Fusion techniques play a vital role in smartphone-based RDT analysis, especially when it comes to integrating imaging and sensor data. Smartphones are equipped with powerful cameras that can capture high-resolution images of RDTs, while sensors such as temperature and pressure detectors can provide additional data that is critical for diagnostic accuracy. By combining both visual and sensor data, multi-modal fusion techniques can enable a more comprehensive and efficient analysis of rapid diagnostic tests.
One of the key advantages of integrating imaging and sensor data is that it allows for real-time monitoring of RDT results. As smartphones are portable and readily accessible, healthcare professionals can quickly capture images of RDTs and obtain sensor data, allowing for immediate analysis and diagnosis. This can be especially beneficial in remote or low-resource settings where traditional diagnostic methods may not be available.
Multi-modal fusion techniques can also help to alleviate some of the limitations associated with relying on visual analysis alone. For example, RDT images may be affected by factors such as lighting and camera angle, which can impact the accuracy of results. However, by incorporating sensor data, fusion techniques can corroborate or refine visual analysis and provide a more robust analysis of RDTs.
Fusion Techniques | Benefits |
---|---|
Image-Sensor Fusion | Real-time monitoring, more accurate results |
Feature-Level Fusion | Improved feature extraction and matching |
Decision-Level Fusion | Increased reliability and diagnostic accuracy |
There are various types of fusion techniques that can be applied to the integration of imaging and sensor data, including image-sensor fusion, feature-level fusion, and decision-level fusion. Each technique offers its own unique benefits, such as real-time monitoring, improved feature extraction and matching, and increased diagnostic accuracy.
Overall, the integration of imaging and sensor data through multi-modal fusion techniques is a powerful tool in achieving comprehensive smartphone-based RDT analysis. By leveraging the capabilities of smartphone hardware and sophisticated algorithms, healthcare professionals can obtain accurate and efficient diagnostic results that can potentially save lives.
Challenges and Limitations
While multi-modal fusion techniques hold great potential for smartphone-based RDT analysis, there are several challenges and limitations that must be addressed to ensure accurate and reliable diagnostic results.
One of the major challenges is data variability. The data obtained from RDTs can vary depending on factors such as the type of test, the condition of the sample, and the environmental conditions. This variability can make it difficult to develop algorithms that can accurately integrate and analyze the data from different modalities.
Another challenge is the complexity of the algorithms required for comprehensive analysis. As the number of modalities increases, the algorithms become more complex, requiring significant computational power and expertise to develop and implement effectively.
The hardware limitations of smartphones can also be a limiting factor in achieving accurate and reliable diagnosis. While smartphone cameras and sensors have improved significantly in recent years, they may not be able to capture all the necessary data required for comprehensive analysis. This could result in incomplete or inaccurate diagnostic results.
It is also important to consider the ethical implications of comprehensive smartphone-based RDT analysis. The responsible use of this technology requires careful consideration of privacy concerns and data security, particularly in healthcare and research settings.
In summary, while multi-modal fusion techniques offer significant potential for smartphone-based RDT analysis, addressing the challenges and limitations associated with this technology is critical to ensuring accurate and reliable diagnostic results.
Future Research Directions
As the field of smartphone-based RDT analysis continues to evolve, there are several promising research directions that could help advance multi-modal fusion techniques for comprehensive analysis. For instance, researchers may explore ways to further integrate different types of data, such as auditory and tactile information, to enhance the accuracy and granularity of results. Additionally, development of algorithms that can adapt to the variability of RDT data could help overcome some limitations of current approaches.
Moreover, hardware improvements in smartphones, such as the incorporation of specialized sensors or lenses, could facilitate more diverse data integration and analysis. Furthermore, the combination of smartphone-based RDT analysis with other emerging technologies, such as artificial intelligence or blockchain, could further enhance the diagnostic capabilities of this approach.
Ultimately, continued research and development are necessary to overcome the challenges and limitations associated with multi-modal fusion techniques for comprehensive smartphone-based RDT analysis. With these advancements, this diagnostic technology has the potential to improve disease diagnosis, treatment, and monitoring, transforming healthcare and enhancing patient outcomes.
Potential Applications in Healthcare
The integration of multi-modal fusion techniques in smartphone-based RDT analysis holds immense potential in various healthcare domains. The ability to perform comprehensive analysis using smartphones enables quicker and more accurate diagnosis, which can be especially beneficial in remote or resource-limited settings.
One potential application is disease diagnosis, where smartphone-based RDT analysis can accurately detect and identify specific diseases. This technology can also aid in personalized medicine, allowing physicians to develop individualized treatment plans based on comprehensive analysis results.
In addition, multi-modal fusion techniques for smartphone-based RDT analysis can support remote healthcare monitoring. Patients can perform diagnostic tests using their smartphones in the comfort of their own homes, without the need for frequent doctor visits. This technology can also aid in healthcare research, enabling the collection of comprehensive data for large-scale studies.
In summary, the integration of multi-modal fusion techniques in smartphone-based RDT analysis has the potential to revolutionize healthcare by providing accurate, efficient, and cost-effective diagnostic solutions. As this technology develops further, the possibilities for its applications in healthcare are endless.
Ethical Considerations
While multi-modal fusion techniques for smartphone-based RDT analysis offer great potential for comprehensive diagnostic testing, it is important to consider the ethical implications associated with this technology. As diagnostic testing becomes increasingly digital and data-driven, it is crucial to ensure that patient privacy and data security are protected.
Comprehensive analysis of smartphone-based RDTs involves the collection and storage of large amounts of personal health data. This information must be handled with care and only used for its intended purpose. Additionally, it is essential to consider the responsible use of this technology to avoid potential biases and discrimination in healthcare and research settings.
Smartphone-based diagnostic technology must comply with legal and ethical standards to ensure that patient confidentiality is maintained. The use of secure and encrypted communication channels can minimize the risk of data breaches and unauthorized access to personal health information.
It is important for healthcare providers and researchers to recognize the potential impact of comprehensive analysis on patient trust and willingness to participate in diagnostic testing. Clear communication and informed consent processes are necessary to ensure that patients are fully aware of the technology and its implications.
In summary, while multi-modal fusion techniques for smartphone-based RDT analysis offer great potential for improving healthcare outcomes, it is vital to prioritize patient privacy, data security, and ethical considerations to ensure the responsible use of this technology.
Adoption and Integration Challenges
The potential benefits of comprehensive smartphone-based RDT analysis are significant, but the widespread adoption and integration of this technology into healthcare systems present several challenges.
One of the main hurdles is cost. Developing multi-modal fusion techniques and incorporating them into smartphone-based RDT analysis may require significant investments in research, development, and infrastructure. Additionally, the cost of the technology may be a barrier for healthcare institutions and patients, limiting access to comprehensive diagnostic testing.
Infrastructure is another obstacle to the successful adoption and integration of comprehensive smartphone-based RDT analysis. Healthcare systems must have the necessary equipment, software, and personnel to support the technology. Moreover, the data generated from comprehensive analysis may require specialized storage and processing capabilities.
User acceptance is also crucial for the adoption of comprehensive smartphone-based RDT analysis. Patients and healthcare professionals may be hesitant to trust the accuracy and reliability of the technology, especially if they are unfamiliar with or skeptical of using smartphones for diagnostic testing.
Despite these challenges, the potential benefits of comprehensive smartphone-based RDT analysis may ultimately overcome these obstacles. As research and development continue to advance the technology, the cost and infrastructure requirements may decrease while user acceptance and trust increase. The integration of this technology into existing healthcare systems has the potential to revolutionize disease diagnosis and treatment, leading to better patient outcomes and improved healthcare delivery.
Conclusion
In conclusion, multi-modal fusion techniques have the potential to revolutionize smartphone-based RDT analysis, enabling comprehensive and accurate diagnostic results. As discussed in this article, the integration of imaging and sensor data, as well as natural language processing algorithms, can enhance the accuracy and efficiency of diagnostic technology.
However, there are several challenges and limitations associated with the implementation of these techniques. Factors such as data variability, algorithm complexity, and potential hardware limitations must be addressed to ensure the reliability and accuracy of comprehensive analysis.
Despite these challenges, the potential applications of multi-modal fusion techniques in healthcare are vast. Disease diagnosis, personalized medicine, and remote healthcare monitoring are just a few examples of domains that can benefit from this technology.
Future Directions
Further research and development are necessary to overcome these challenges and ensure the successful integration of multi-modal fusion techniques into healthcare systems. Advancements in data integration, algorithm development, and hardware improvements could enhance the accuracy and efficiency of diagnostic technology.
Overall, the potential of comprehensive smartphone-based RDT analysis using fusion techniques is significant. With careful consideration of ethical concerns and adoption challenges, this technology has the potential to improve healthcare outcomes and transform the medical industry.