Deep Learning Models for Phenotypic Analysis Explained
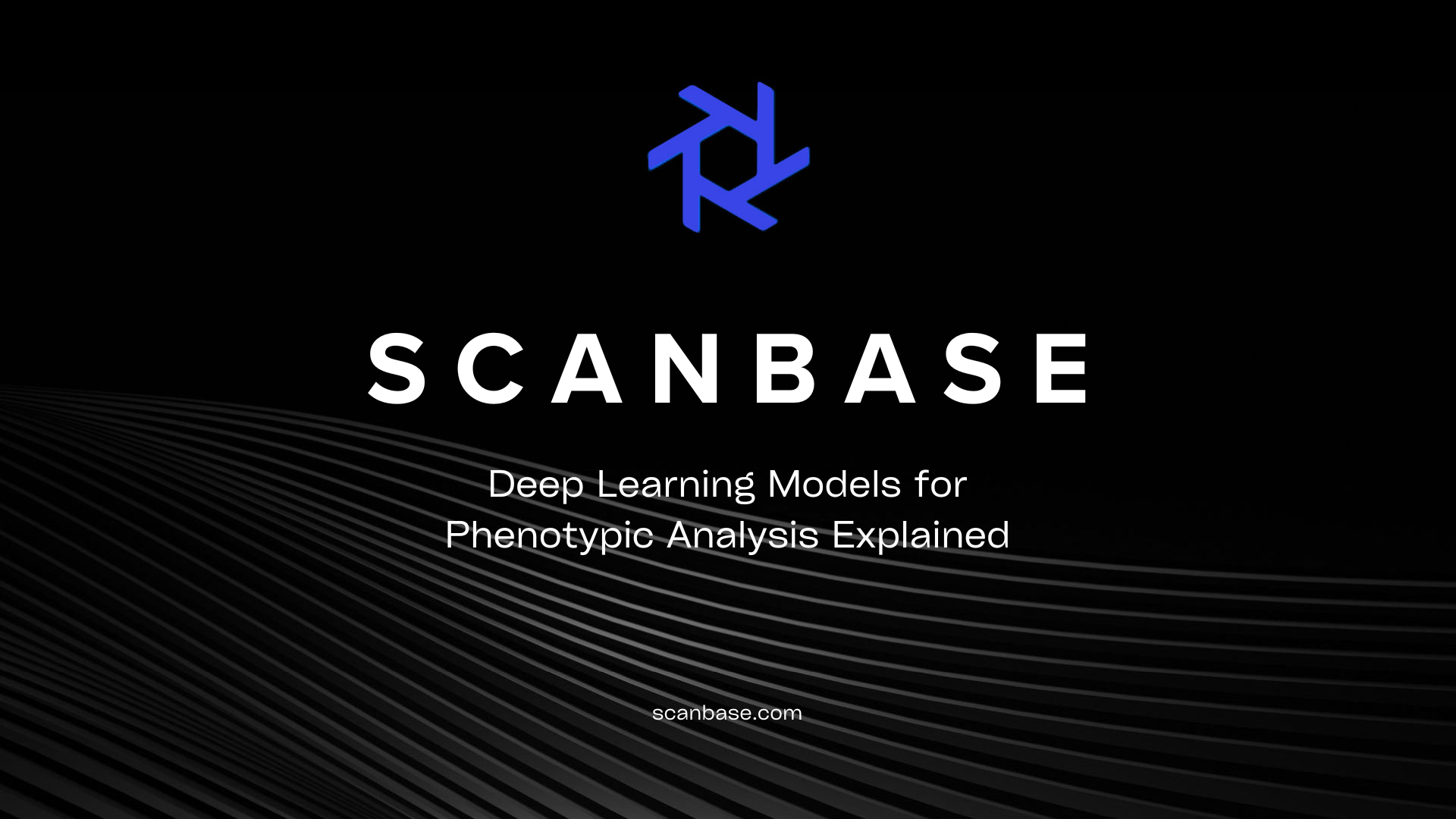
Welcome to our article on deep learning models for phenotypic analysis. In this section, we will introduce what deep learning is and how it is used in this specific context. Deep learning is a subset of machine learning that involves training artificial neural networks to recognize patterns and make decisions.
Phenotypic analysis, on the other hand, refers to the study of an organism's observable traits or characteristics. It is a crucial aspect of biotechnology and medical research that enables scientists to identify and understand genetic differences and their effects on different organisms.
Deep learning models are increasingly being used in phenotypic analysis to automate image analysis and biological data processing. These models are designed to recognize patterns and identify specific traits in images, allowing researchers to analyze large volumes of data quickly and accurately. In the following sections, we will explore the importance of phenotypic analysis in biotechnology, the role of deep learning models, and how they are used to analyze biological data.
Phenotypic Analysis and Its Importance in Biotechnology
Phenotypic analysis is the study of observable characteristics, or phenotypes, of an organism that result from the interaction between its genes and the environment. These characteristics can range from physical traits, such as height or eye color, to more complex traits, such as behavior and disease susceptibility.
In biotechnology, phenotypic analysis is critical for understanding the function of genes and identifying potential drug targets for disease treatment. It also plays a crucial role in genetic engineering and crop improvement by allowing researchers to selectively breed plants and animals with desired traits.
Phenotypic analysis has applications in a variety of industries, including healthcare, agriculture, and environmental science. For example, it can be used to identify markers for disease diagnosis and prognosis, track the spread of infectious diseases, and measure the impact of environmental factors on plant growth and development.
Introduction to Deep Learning
Deep learning is a subset of machine learning, a type of artificial intelligence that allows computers to learn and improve from experience without being explicitly programmed. Deep learning models are designed to mimic the way the human brain works, with layers of interconnected nodes that process information and extract features from data.
These models are trained on large datasets and use complex algorithms to learn patterns and relationships within the data. They are particularly useful for analyzing complex data, such as images or biological data, and can be used to identify and classify specific phenotypic traits.
Computer Vision and Biological Data Analysis
Computer vision is a critical tool in phenotypic analysis, especially when it comes to analyzing biological data. The technology allows researchers to identify and extract critical data points from images, making it easier to find and assess patterns.
By using computer vision in combination with deep learning models, researchers can identify specific characteristics, such as the morphology of a cell or the shape of a tissue sample. This information can then be used to identify specific phenotypic traits, such as disease progression or drug efficacy.
One of the major advantages of using computer vision in biological data analysis is the ability to process large amounts of data quickly and accurately. This can help reduce the time and cost involved in conducting research, making it easier to identify new treatments and cures for diseases.
Overall, the combination of computer vision and deep learning models has revolutionized the field of phenotypic analysis, allowing researchers to identify new patterns and insights that were previously impossible to find. As the technology continues to advance, we can expect to see even more significant breakthroughs in biotechnology and medical research.
Image Analysis and Its Role in Phenotypic Analysis
Image analysis plays a crucial role in phenotypic analysis, as it is used to identify and quantify specific phenotypic traits in biological data. Through the use of image analysis, researchers can extract meaningful information from complex biological images that would be difficult or impossible to discern with the naked eye.
For example, image analysis can be used to quantify the size and shape of individual cells or to identify the presence of specific proteins within a cell. By analyzing these images, researchers can gain insight into various biological processes and identify patterns or trends that may be indicative of disease or other abnormalities.
In the field of genomics, image analysis is used to identify genetic sequences and map their locations within the genome. This information can be used to study the effects of genetic variations on phenotypic traits and to identify potential targets for drug development.
Overall, image analysis is a powerful tool for phenotypic analysis, providing researchers with the ability to extract detailed information from complex biological data and uncover insights that would be impossible to discern through other means.
Artificial Intelligence in Phenotypic Analysis
Artificial intelligence (AI) has revolutionized the field of phenotypic analysis. It has enabled researchers to process and analyze large datasets quickly and accurately, identifying patterns and making predictions that would have been impossible with traditional methods.
AI algorithms can be trained to recognize specific phenotypic traits by analyzing large datasets of images and identifying common features. This allows researchers to quickly and accurately identify mutations, genetic variations, and other factors that affect phenotype.
AI can also be used to identify new phenotypic traits that were previously unknown or difficult to identify using traditional methods. For example, AI algorithms have been used to identify patterns in medical images that are indicative of specific diseases or conditions.
The use of AI in phenotypic analysis has the potential to accelerate medical and biological research, leading to new treatments and cures for diseases. However, it is important to ensure that these technologies are developed and used ethically and responsibly.
The Advantages of Deep Learning Models in Phenotypic Analysis
Deep learning models have revolutionized the field of phenotypic analysis, offering several advantages over traditional methods. Some of the key benefits include:
Advantage | Description |
---|---|
Accuracy | Deep learning models can accurately identify and classify phenotypic traits, reducing errors and ensuring data accuracy. |
Speed | These models can process large amounts of biological and medical data quickly, allowing researchers to analyze data more efficiently and effectively. |
Automation | Deep learning models can automate the analysis process, reducing the need for manual labor and freeing up researchers to focus on other areas of research. |
Flexibility | These models can adapt and learn from new data, allowing researchers to refine their analysis methods and improve accuracy over time. |
The advantages of deep learning models in phenotypic analysis are clear, and their potential for advancing biotechnology and medical research is immense. By accurately and efficiently analyzing biological and medical data, these models can help researchers develop new treatments, improve patient outcomes, and unlock the mysteries of the natural world.
Pattern Recognition in Deep Learning Models
Deep Learning models rely on complex algorithms that can identify patterns within large datasets. This process, known as pattern recognition, is crucial in phenotypic analysis, as it enables researchers to identify specific traits and characteristics of organisms.
Deep Learning models use artificial neural networks, which are modeled after the neurons in the human brain. These networks consist of multiple layers, each of which performs a specific task, such as identifying edges or shapes within an image. By analyzing these individual components, the network can piece together a complete understanding of the image and identify specific features.
Pattern recognition is particularly important in identifying complex traits, such as those found in medical imaging. For example, by training a Deep Learning model to analyze medical images, researchers can identify subtle changes in tissue density or structure that may indicate the presence of a disease.
Overall, pattern recognition is a crucial component of Deep Learning models and has revolutionized the field of phenotypic analysis. By enabling researchers to identify specific traits and characteristics within large datasets, these models have opened up new avenues for medical and biological research.
Medical Image Processing and Its Role in Deep Learning Models for Phenotypic Analysis
Medical image processing plays a critical role in the application of deep learning models for phenotypic analysis. This process involves enhancing and analyzing medical images to extract meaningful data that can be used for phenotypic analysis. The use of deep learning models in medical image processing has significantly improved the accuracy and efficiency of the analysis process.
One of the key advantages of using deep learning models for medical image processing is their ability to identify specific phenotypic traits accurately. These traits can range from certain patterns in the image to specific shapes and sizes. With the help of deep learning models, researchers can extract this data accurately, providing important information for various biological and medical research fields.
Application | Description |
---|---|
Medical Diagnosis | Deep learning models can be used to analyze medical images and detect anomalies with high accuracy, providing critical information for diagnosis and treatment planning. |
Drug Discovery | Phenotypic analysis using deep learning models can help researchers identify potential drug targets and develop targeted therapies that can be used to treat various diseases. |
Genetic Research | Deep learning models can be used to analyze genetic data and identify specific genetic traits associated with certain phenotypic traits, providing important insights into disease susceptibility and prevention. |
The future of deep learning models in medical image processing and phenotypic analysis is promising. Advancements in deep learning technology and increased availability of medical data are driving innovation in this field, leading to more accurate and efficient analysis methods. As a result, the applications of deep learning models in phenotypic analysis are rapidly expanding, providing important insights for biological and medical research.
The Future of Deep Learning Models in Phenotypic Analysis
As technology continues to advance, we can expect deep learning models for phenotypic analysis to play an increasingly important role in biotechnology and medical research. One potential area of growth is the use of deep learning models in drug discovery, where they can help researchers identify new compounds that could be used to treat a variety of diseases.
Another area where deep learning models could prove particularly useful is in the analysis of complex biological data. By using machine learning algorithms to analyze large amounts of data, researchers can gain a better understanding of how different phenotypic traits are related to one another, and potentially identify new genetic markers for disease.
Moreover, as deep learning models become more sophisticated and better able to process and analyze complex visual data, they could have a transformative impact on medical imaging. With the ability to quickly identify and analyze patterns in medical images, deep learning models could help doctors diagnose diseases earlier and more accurately, potentially leading to better patient outcomes.
Overall, the future of deep learning models in phenotypic analysis is bright. With their ability to process large amounts of complex data, identify patterns and relationships between traits, and quickly analyze visual data like medical images, they have the potential to revolutionize many areas of biotechnology and medical research.
Conclusion
Phenotypic analysis has been an integral part of biotechnology and medical research for many years. However, with the advent of deep learning models, the field has seen a remarkable transformation. These models use artificial intelligence and pattern recognition to identify specific traits, enabling researchers to obtain more accurate and detailed results.
The advantages of deep learning models in phenotypic analysis are numerous. From computer vision to image analysis, these models have revolutionized the way we approach biological data analysis. Additionally, the role of medical image processing cannot be overlooked, as it has proven to be an essential tool in identifying and understanding complex medical conditions.
Looking towards the future, the potential advancements of deep learning models in phenotypic analysis are incredibly promising. By improving accuracy and efficiency, these models can significantly impact biotechnology and medical research, leading to new breakthroughs in disease diagnosis and treatment.
Final thoughts
Overall, deep learning models have transformed phenotypic analysis and provided researchers with a powerful tool for understanding biological data. By combining artificial intelligence, computer vision, and image analysis, these models have enhanced the accuracy and efficiency of phenotypic analysis, paving the way for significant advancements in biotechnology and medical research.