Deep Learning-based Classification of Rapid Diagnostic Tests Explained
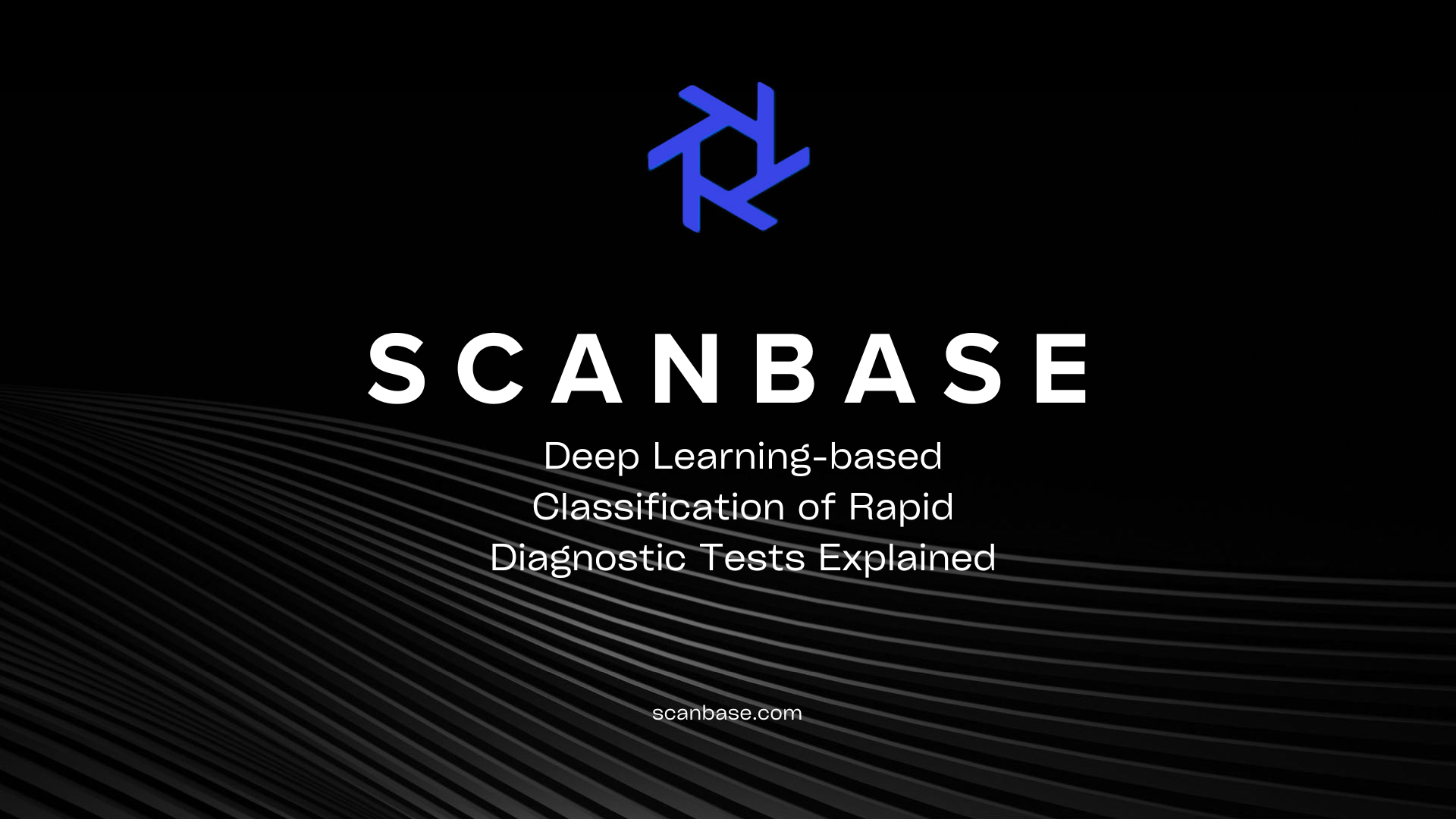
In recent years, the field of medical diagnostics has witnessed exciting advancements in technology, with deep learning-based classification being one of the most promising. Deep learning-based classification involves the use of artificial intelligence and machine learning to analyze and interpret data from rapid diagnostic tests to provide accurate and reliable results.
In this article, we will explore the applications of deep learning-based classification in medical diagnostics, its potential benefits, and impact on healthcare outcomes. We will also discuss the challenges and limitations faced in implementing this technology and its ethical considerations.
Key Takeaways
- Deep learning-based classification involves the use of artificial intelligence and machine learning to analyze and interpret data from rapid diagnostic tests.
- This technology has the potential to improve the accuracy and reliability of medical diagnostics and advance healthcare outcomes.
- Challenges and limitations exist in implementing deep learning-based classification in medical diagnostics, and ethical considerations must be taken into account.
Understanding Rapid Diagnostic Tests
Rapid diagnostic tests are medical diagnostic tools that are designed to provide quick and accurate results. These tests are an important part of modern healthcare as they can help healthcare providers to identify and manage illnesses in a timely manner. Rapid diagnostic tests can be used to detect a wide range of conditions, from infectious diseases to cancer.
One of the main advantages of rapid diagnostic tests is that they can be performed quickly and easily, often without the need for specialized equipment or training. This makes them an ideal tool for use in resource-limited settings where access to traditional diagnostic methods may be limited.
Introduction to Deep Learning and Artificial Intelligence
Deep learning is a subset of machine learning and a type of artificial intelligence that involves training deep neural networks to recognize patterns in data. By using a complex system of algorithms and models, deep learning can analyze vast amounts of data and identify patterns that humans might miss. This technology has revolutionized many fields, including healthcare and medical diagnostics.
Artificial intelligence refers to the simulation of human intelligence in machines that are trained to think and learn like humans. AI systems use algorithms and statistical models to analyze data and make predictions or decisions without explicit human instruction. Medical diagnostics is one of many areas where AI is making significant strides in improving patient outcomes.
Deep Learning in Medical Diagnostics
Deep learning is a form of machine learning that draws inspiration from the structure and function of the human brain to analyze and interpret complex data. In medical diagnostics, deep learning has emerged as a powerful tool to analyze and interpret data from rapid diagnostic tests. The ability of deep learning models to understand patterns and relationships in large datasets makes them ideal for analyzing medical data.
One of the biggest advantages of deep learning in medical diagnostics is its potential to detect patterns that may not be visible to the human eye. For example, deep learning models can detect subtle variations in color or texture that may indicate the presence of a particular disease or condition.
Deep learning algorithms can also be trained to recognize specific features in medical images, such as tumors or lesions. This enables them to accurately classify images and diagnose diseases with a high level of accuracy.
Another advantage of deep learning in medical diagnostics is its ability to process large amounts of data quickly and accurately. This makes it an ideal tool for rapid testing, where speed and accuracy are crucial for effective diagnosis and treatment.
Overall, the application of deep learning in medical diagnostics has shown promising results in improving the accuracy and speed of diagnosis. Its ability to analyze and interpret complex data from rapid diagnostic tests makes it an important tool in the healthcare technology landscape.
Deep Neural Networks and Image Processing Techniques
Deep neural networks and image processing techniques are at the core of deep learning-based classification of rapid diagnostic tests. These technologies enable the automation of the classification process, enabling faster and more accurate results.
Deep neural networks are modeled after the structure and function of the human brain. They consist of layers of interconnected nodes that process and analyze data, enabling them to recognize patterns and make predictions. In the context of rapid diagnostic tests, deep neural networks are used to analyze images of test results and classify them based on their characteristics and features.
Image Processing Techniques | Examples |
---|---|
Thresholding | Separating the foreground and background of an image by setting a threshold value |
Segmentation | Dividing an image into regions with similar characteristics |
Feature Extraction | Identifying and isolating specific features within an image |
Image processing techniques are used to enhance the images of rapid diagnostic tests, enabling deep neural networks to analyze them more accurately. These techniques include thresholding, segmentation, and feature extraction, among others. By isolating and identifying specific features within an image, image processing techniques enable deep neural networks to make accurate and reliable predictions.
Overall, the combination of deep neural networks and image processing techniques is an effective approach to the classification of rapid diagnostic tests. By automating and improving the accuracy of the classification process, these technologies have the potential to revolutionize medical diagnostics and improve healthcare outcomes.
Pattern Recognition and Accuracy Improvement
Deep learning algorithms are designed to recognize complex patterns in data, allowing for accurate interpretation and analysis of rapid diagnostic tests. Pattern recognition is a critical feature of this technology, enabling the system to identify specific markers or features in the data that are indicative of a certain disease or condition.
By utilizing deep learning-based classification, accuracy and reliability of rapid diagnostic tests can be significantly improved. Traditional diagnostic tests are often limited in their ability to detect subtle changes in biological markers, leading to false negatives or false positives. Deep learning algorithms, on the other hand, can identify even minor changes in the data that may not be visible to the human eye.
Benefits of Deep Learning in Accuracy Improvement | Limitations of Deep Learning-based Classification |
---|---|
|
|
Despite its limitations, deep learning-based classification has the potential to revolutionize medical diagnostics by improving accuracy and reducing the time required for diagnosis. By identifying patterns and biomarkers that may not be discernible to humans, this technology can lead to earlier detection and treatment of diseases, ultimately improving patient outcomes.
Challenges and Limitations of Deep Learning-based Classification
While deep learning-based classification has shown significant promise in improving the accuracy and efficiency of rapid diagnostic tests, there are several challenges and limitations that must be considered when implementing this technology.
One of the major challenges is ensuring that the deep neural network is trained on a diverse dataset that accurately reflects the population being tested. If the dataset is biased or does not represent a wide range of patients, the accuracy of the classification may be compromised.
Another challenge is the interpretability of the results. Deep neural networks operate in a black box fashion, making it difficult to understand how and why a certain classification was made. This lack of transparency can be problematic when it comes to making important medical decisions based on the test results.
In addition, the cost and technical requirements of implementing deep learning-based classification may be prohibitive for some healthcare facilities, particularly those in low-resource settings. There may also be concerns about data privacy and security when it comes to using sensitive medical information for training and testing purposes.
Exploring Potential Solutions
Despite these challenges and limitations, there are several potential solutions that can be explored to improve the reliability and accessibility of deep learning-based classification in rapid diagnostic tests.
One approach is to improve the transparency and interpretability of deep neural networks. This can be done by implementing techniques such as attention mechanisms, which highlight the regions of an image that are most important for making a certain classification.
Another solution is to develop more diverse and representative datasets for training and testing purposes. This can be achieved by partnering with healthcare facilities and organizations in different regions to gather data from a wider range of patients.
Finally, efforts can be made to reduce the cost and technical requirements of implementing deep learning-based classification. This can involve developing simpler and more user-friendly interfaces, as well as leveraging cloud-based platforms for data storage and analysis.
Overall, while there are certainly challenges and limitations to implementing deep learning-based classification in rapid diagnostic tests, there are also many potential solutions and opportunities for improvement. By continuing to explore and refine this technology, we can work towards more accurate, efficient, and accessible medical diagnostics for all.
Future Prospects and Applications
Deep learning-based classification of rapid diagnostic tests heralds a new era of healthcare technology innovation and development. The rapid evolution of artificial intelligence (AI) and machine learning algorithms presents exciting opportunities for improving medical diagnostics and transforming patient outcomes. Below are some of the potential benefits and applications of deep learning-based classification in rapid diagnostic tests:
- Improved accuracy and efficiency of medical diagnostics, enabling earlier disease detection and treatment
- Cost-effective and scalable diagnostic solutions, particularly in resource-limited settings
- Reduction in the need for human interpretation and diagnosis of test results, minimizing the risk of errors and variability
- Expanded access to healthcare for underserved populations through mobile and point-of-care testing devices
- Enhanced drug development and clinical trial design, enabling more precise patient stratification and targeted therapy
As the technology continues to advance and be refined, the potential impact on healthcare outcomes worldwide is significant.
Case Studies and Success Stories
Deep learning-based classification has been successfully applied in several medical diagnostic tests, improving accuracy and reducing diagnostic time. Here are some examples of success stories:
Test Type | Application | Results |
---|---|---|
Rapid Malaria Testing | Identification of malaria parasites in blood samples | Accuracy improved from 80% to 95%, reducing the number of false-positives and false-negatives |
Pregnancy Testing | Detection of human chorionic gonadotropin (hCG) hormone in urine samples | Accuracy improved from 92% to 98%, reducing the need for confirmatory testing and increasing confidence in results |
Blood Glucose Testing | Measurement of glucose levels in blood samples | Accuracy improved from 87% to 95%, reducing the risk of incorrect medication dosing and improving diabetes management |
These success stories demonstrate the potential of deep learning-based classification in improving the accuracy and efficiency of rapid diagnostic tests, leading to better healthcare outcomes.
Challenges and Limitations of Deep Learning-based Classification
While deep learning-based classification has shown great promise in improving the accuracy and reliability of rapid diagnostic tests, there are also challenges and limitations that must be considered.
One major challenge is the requirement for large amounts of high-quality data to train deep neural networks. In some cases, obtaining such data can be difficult or expensive, particularly in resource-limited settings where many rapid diagnostic tests are performed.
Another limitation is the potential for algorithmic bias, where the data used to train deep learning models may not be representative of the entire population. This can result in inaccurate or unreliable classification of certain subgroups.
Additionally, there is a need for transparent and rigorous regulatory frameworks to ensure the safe and ethical use of deep learning-based classification in healthcare. This involves ensuring that algorithms are transparent and interpretable, protecting patient privacy, and preventing unintended consequences such as increased healthcare disparities.
Despite these challenges and limitations, ongoing research and development in this field offer significant potential for improving the accuracy, speed, and accessibility of medical diagnostics through the use of deep learning-based classification.
Potential Benefits and Impact on Healthcare
The implementation of deep learning-based classification in rapid diagnostic tests offers significant potential benefits for healthcare. By providing accurate and timely diagnoses, these tests can lead to faster and more effective treatments, resulting in better patient outcomes.
One of the key advantages of deep learning-based classification is its ability to analyze and interpret large amounts of data quickly and accurately. This can help healthcare professionals make informed and evidence-based decisions, leading to more precise and personalized treatment plans. Additionally, the use of rapid diagnostic tests reduces the need for more invasive and time-consuming procedures, which not only improves patient comfort but also helps to reduce healthcare costs.
Moreover, the implementation of deep learning-based classification in rapid diagnostic tests promotes the development of healthcare technology. As new applications and technologies are developed, the potential for improving healthcare outcomes becomes even greater.
Overall, the integration of deep learning-based classification in rapid diagnostic tests has the potential to significantly impact the healthcare industry, leading to improved patient care and outcomes, increased efficiency and accuracy in diagnosis, and the development of new and innovative healthcare technologies.
Conclusion
The use of Deep Learning-based Classification in Rapid Diagnostic Tests has the potential to revolutionize the medical diagnostics industry. This cutting-edge technology, backed by Artificial Intelligence and Machine Learning, can accurately analyze and interpret complex data from rapid diagnostic tests, improving their reliability and efficiency.
While the technology is still in its infancy, it has already shown promising results in various medical fields. Deep Neural Networks and Image Processing techniques, coupled with Pattern Recognition algorithms, have significantly increased the accuracy of rapid diagnostic tests.
The Potential Benefits
The potential benefits of this technology are immense. Rapid diagnostic tests can help in the early detection of diseases, saving valuable time and resources. The accuracy and efficiency of these tests will also ensure that treatments are provided promptly, potentially saving lives.
The technology can also eliminate the need for trained professionals to interpret and diagnose test results, reducing the cost and time required for medical testing. This can make medical diagnostics and treatment more accessible to people who live in areas with limited healthcare facilities, thereby improving overall healthcare outcomes.
The Impact on Healthcare
The impact of Deep Learning-based Classification in Rapid Diagnostic Tests on the healthcare industry is already evident. The technology is transforming the way diagnoses are made, making them faster, more accurate, and cost-effective.
The potential applications of this technology in various medical fields are vast. It can help detect and diagnose diseases in real-time, reducing the need for complicated laboratory procedures. It can also help in the development of personalized medicines, customized for each patient's unique genetic makeup. This can potentially lead to the development of more effective treatments with fewer side effects.
Final Thoughts
Deep Learning-based Classification in Rapid Diagnostic Tests is a fascinating and rapidly evolving technology. While it is not without its limitations and challenges, the positive impact it can have on the healthcare industry is undeniable. It is up to us to ensure that the technology is developed responsibly, with due consideration for ethical and regulatory frameworks, but we can look forward to a future where medical diagnoses are quick, accurate, and accessible to all.