Biomedical Signal Analysis for Rapid Diagnostic Test Interpretation
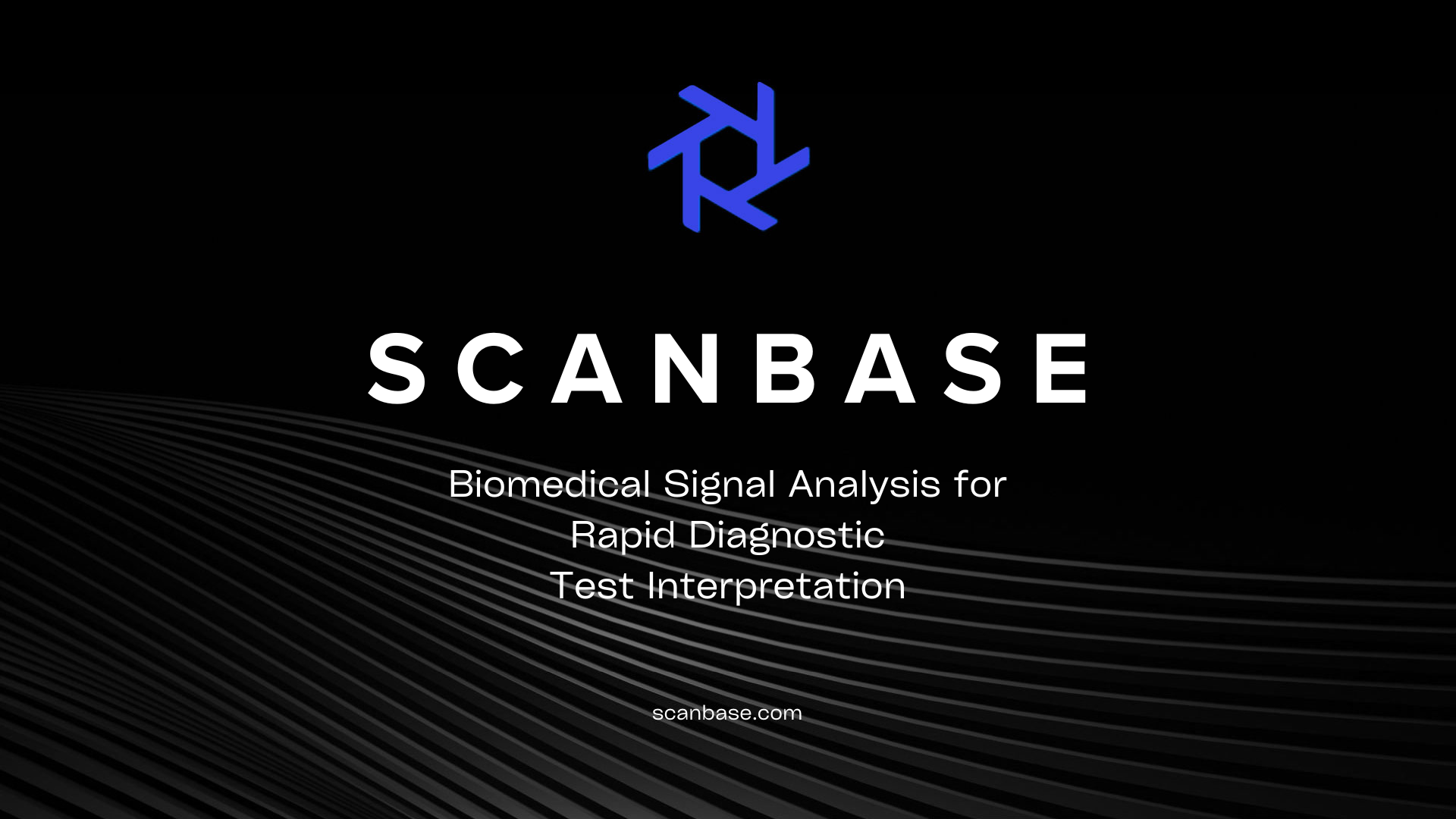
Biomedical signal analysis is a powerful tool used to improve the accuracy and speed of diagnostic test interpretation. With its advanced signal classification and machine learning algorithms, biomedical signal analysis provides a deeper understanding of the data generated by rapid diagnostic tests. By analyzing biomedical data, signal processing techniques used in biomedical signal analysis help in identifying early warning signs of disease, reducing the time taken to interpret test results, and improving diagnostic accuracy.
This section aims to introduce the concept of diagnostic test interpretation and its importance in the healthcare industry. It will discuss the various signal processing techniques used in biomedical signal analysis and how biomedical data analysis is performed. Furthermore, this section will highlight the role of biomedical signal analysis in improving diagnostic accuracy and reducing the time taken for diagnosis.
What is Biomedical Signal Analysis?
Biomedical signal analysis is the process of acquiring and processing physiological signals to extract useful information for medical diagnosis and treatment. This involves the application of various signal processing techniques to analyze the data and identify patterns that are indicative of certain conditions or diseases.
There are several different types of signals that can be analyzed using biomedical signal analysis, including electrocardiograms (ECG), electroencephalograms (EEG), and electromyograms (EMG). Each of these signals provides different information about the body's physiological state, and can be used to diagnose a wide range of medical conditions.
Signal processing techniques are used to extract meaningful information from the signals, such as identifying abnormalities or trends that are indicative of certain medical conditions. These techniques include filtering, feature extraction, signal classification, and machine learning algorithms.
Biomedical data analysis is a crucial component of biomedical signal analysis, as accurate data collection is essential for achieving accurate diagnoses. The use of sophisticated signal analysis techniques enables healthcare professionals to interpret test results more quickly and accurately, leading to improved patient outcomes.
Why is Biomedical Signal Analysis Important for Rapid Diagnostic Tests?
Biomedical signal analysis plays a crucial role in improving the accuracy and speed of diagnostic tests. The analysis of biomedical signals, such as electrocardiograms (ECG), electroencephalograms (EEG), and photoplethysmograms (PPG), can reveal valuable information about a patient's health status and aid in diagnosis. Signal classification and machine learning algorithms are used to analyze this data, providing enhanced interpretation of rapid diagnostic tests.
Diagnostic accuracy improvement is a primary benefit of biomedical signal analysis. Machine learning algorithms can analyze vast amounts of biomedical data quickly and accurately, helping to identify patterns and anomalies that may indicate the presence of a particular disease. Furthermore, real-time signal analysis allows for the interpretation of test results in seconds, reducing the time and effort needed to diagnose diseases.
Biomedical signal analysis is particularly essential in the context of rapid diagnostic tests, where accurate and speedy interpretation is crucial. These tests rely on the analysis of biological samples, such as blood, urine, and saliva, to diagnose a range of diseases, including infectious diseases and cancer. Biomedical signal analysis can enhance the accuracy and sensitivity of these tests, making them more reliable and effective in diagnosing illnesses.
Why is real-time signal analysis important for diagnostic accuracy?
Real-time signal analysis is critical in improving diagnostic accuracy, particularly in situations where time is of the essence. For instance, in emergencies, such as a heart attack, rapid and accurate diagnosis can be life-saving. Real-time signal analysis can identify changes in the ECG signal indicating a heart attack, allowing for prompt treatment.
Another example is the diagnosis of sepsis, a life-threatening condition that requires immediate medical attention. Biomedical signal analysis can identify specific biomarkers in the patient's blood, indicating the presence of sepsis. Real-time signal analysis can help diagnose sepsis quickly, allowing for timely intervention and improving patient outcomes.
How is Data-Driven Analysis Used in Biomedical Signal Analysis?
Data-driven analysis is a critical component of biomedical signal analysis. This approach involves the use of machine learning algorithms to analyze large datasets and identify patterns and trends in the data. Data-driven analysis allows for the development of more accurate models for classifying signals and predicting outcomes. Additionally, it enables researchers to identify new biomarkers for disease diagnosis and treatment.
Machine learning algorithms are used to analyze various types of data, including electroencephalogram (EEG), electrocardiograph (ECG) and electromyography (EMG) signals. These algorithms can be trained to recognize specific patterns in the data and use this information to classify signals and predict outcomes. Machine learning algorithms are also used in real-time signal analysis, allowing for immediate interpretation of biomedical data.
Data collection and preprocessing are critical components of data-driven analysis. The accuracy of machine learning algorithms is dependent on the quality of the data used to train them. As a result, it is important to collect high-quality data and preprocess it to remove any noise or artifacts that may interfere with accurate signal classification. Once the data has been collected and preprocessed, it can be used to train machine learning algorithms and develop more accurate models for biomedical signal analysis.
Examples of Data-Driven Analysis in Biomedical Signal Analysis
One example of the use of data-driven analysis in biomedical signal analysis is the development of algorithms to improve the accuracy of cardiac monitoring. Researchers have developed machine learning algorithms that can analyze ECG data and predict the likelihood of future cardiac events. These algorithms can also be used to identify new biomarkers for cardiac disease diagnosis and treatment.
Another example of data-driven analysis in biomedical signal analysis is the use of EEG data to predict seizure onset. Machine learning algorithms have been trained to recognize specific patterns in EEG data that indicate an impending seizure. These algorithms can be used to develop warning systems that alert patients and healthcare providers to the onset of a seizure, allowing for prompt intervention.
Data-driven analysis is essential for the advancement of biomedical signal analysis. As new technologies and techniques emerge, the use of machine learning algorithms and real-time signal analysis will continue to grow, enabling researchers to develop new models for disease diagnosis and treatment.
The Benefits of Biomedical Signal Analysis for Healthcare
Biomedical signal analysis has numerous benefits for healthcare, particularly in terms of improving diagnostic accuracy and utilizing sophisticated signal processing techniques. Here are some key benefits:
- Improved Diagnostic Accuracy: Biomedical signal analysis allows for the detection of subtle biological signals that can be missed by the human eye. By analyzing these signals using machine learning algorithms and other data-driven techniques, healthcare providers can improve diagnostic accuracy and reduce the likelihood of misdiagnosis.
- Early Disease Detection: The use of sophisticated signal processing techniques can help identify early warning signs of disease, allowing for early intervention and improved patient outcomes.
- Real-Time Signal Analysis: Biomedical signal analysis can be used for real-time signal analysis, allowing healthcare providers to quickly diagnose and treat patients. This is particularly important in the context of rapid diagnostic tests, where timely interpretation of test results is crucial.
- Customized Treatment Plans: By analyzing biomedical signals, healthcare providers can develop customized treatment plans tailored to each patient's specific needs. This can lead to improved patient outcomes and reduced healthcare costs.
The benefits of biomedical signal analysis for healthcare are clear, and the continued development of signal processing techniques and machine learning algorithms will only enhance the field's potential impact on healthcare.
Examples of Biomedical Signal Analysis in Practice
Biomedical signal analysis has been used in various diagnostic tests to improve accuracy and speed up the interpretation process. Here are a few examples:
Diagnostic Test | Signal Analysis Technique | Result |
---|---|---|
Electroencephalogram (EEG) | Wavelet transform | Improved detection of epileptic seizures |
Electrocardiogram (ECG) | Machine learning algorithms | Identification of heart disease risk factors |
Magnetic Resonance Imaging (MRI) | Fourier transform | Identification of structural abnormalities in the brain |
Real-time signal analysis has also been used in various medical devices to monitor patients and detect early warning signs of disease. For example, wearable devices that use electrocardiography (ECG) signal analysis can detect abnormal heart rhythms and alert patients or healthcare providers.
"The use of signal analysis techniques in diagnostic tests has helped improve the accuracy and speed of disease detection, resulting in better patient outcomes."
Challenges and Limitations of Biomedical Signal Analysis
While biomedical signal analysis offers significant potential for improving diagnostic accuracy and speed, there are also several challenges and limitations associated with the field.
One key challenge is ensuring the accuracy of data-driven analysis. While machine learning algorithms can improve the accuracy of diagnostic tests, they rely on accurate data collection and classification. As such, there is a need for well-designed studies that collect and analyze high-quality data to improve the accuracy of signal analysis.
Another challenge is the lack of standardized approaches to signal processing and classification. Without standardized methods, it can be difficult to compare results across different studies and technologies. Additionally, the use of different signal processing techniques can lead to variability in results, which can impact the accuracy of diagnostic tests.
There is also a need to improve the real-time signal analysis capabilities of current technologies. While real-time signal analysis is essential for rapid diagnostic tests, many technologies are still limited by their processing capabilities and the speed of data transmission.
Potential Solutions
To overcome these challenges, there is a need for continued research and collaboration in the field of biomedical signal analysis. This includes the development of standardized approaches to signal processing and classification, as well as the integration of real-time signal analysis capabilities into current technologies.
Additionally, the use of multiple signal processing techniques and the integration of complementary data sources (e.g. clinical data) can help improve the accuracy of signal analysis and diagnostic tests.
The Future of Biomedical Signal Analysis
Biomedical signal analysis is rapidly advancing, and the future is exciting. The use of machine learning algorithms and real-time signal analysis will continue to shape the field of diagnostic test interpretation.
One potential development is the use of signal analysis for disease prevention. By analyzing biomedical signals in real-time, early warning signs of disease can be detected and treated before they become serious. This approach has the potential to revolutionize the way healthcare is delivered, with a focus on prevention rather than treatment.
Another area where biomedical signal analysis is expected to have a significant impact is personalized medicine. By analyzing individual data sets, doctors will be able to tailor treatments to specific patients, increasing effectiveness and minimizing side effects.
Despite the potential of biomedical signal analysis, there are still challenges to be overcome. One major challenge is the need for accurate data collection. If data is skewed or incomplete, the accuracy of any analysis will be compromised. However, developments in data capture technology are addressing this issue, paving the way for more accurate analysis.
Overall, the future of biomedical signal analysis is promising. As technology advances and more data is collected, the accuracy and effectiveness of diagnostic test interpretation will continue to improve, benefiting individuals and healthcare systems as a whole.
The Role of Biomedical Signal Analysis in COVID-19 Testing
The COVID-19 pandemic has highlighted the importance of diagnostic testing in controlling the spread of the virus. Biomedical signal analysis has played a significant role in improving the accuracy and speed of COVID-19 testing.
One of the key applications of biomedical signal analysis in COVID-19 testing has been the interpretation of rapid diagnostic tests. These tests are simple to perform and provide results within minutes, but their accuracy can be affected by factors such as the timing of the test and the skill of the person performing it. Biomedical signal analysis can improve the accuracy of these tests by analyzing the signals generated by the test and applying machine learning algorithms to classify the results.
Real-time signal analysis is also important in COVID-19 testing. By analyzing signals in real-time, healthcare professionals can quickly identify positive cases and take appropriate measures to prevent further spread of the virus. Biomedical signal analysis can also help identify early warning signs of COVID-19, allowing for early intervention and treatment.
Signal processing techniques are also used in COVID-19 testing to improve the accuracy of results. By analyzing the signals generated by the test, healthcare professionals can identify patterns and trends that may indicate the presence of the virus.
The use of biomedical signal analysis in COVID-19 testing is an exciting development in the fight against the pandemic. With continued research and development, signal analysis techniques may become even more sophisticated and accurate, further improving the speed and accuracy of COVID-19 testing.
The Importance of Biomedical Signal Analysis for Enhanced Interpretation of Rapid Diagnostic Tests
Biomedical signal analysis plays a critical role in improving diagnostic accuracy and speed through the use of signal classification and machine learning algorithms. By analyzing biomedical data using a variety of signal processing techniques, data-driven analysis can help identify early warning signs of disease, ultimately leading to earlier and more effective treatment.
Accurate data collection is essential for successful biomedical signal analysis, as is the use of real-time signal analysis for rapid diagnostic tests. Machine learning algorithms also have an important role to play, and are used in various types of data collection and analysis to improve diagnostic accuracy.
There are a number of benefits to using biomedical signal analysis in healthcare, such as improved diagnostic accuracy and the use of sophisticated signal processing techniques. However, there are also challenges and limitations that must be addressed, particularly in the realm of data-driven analysis and diagnostic accuracy improvement.
Looking to the Future
The potential impact of biomedical signal analysis on healthcare is significant, and future developments are likely to continue to shape the field. Machine learning algorithms and real-time signal analysis will play an increasingly important role in this, as will the use of signal analysis for disease prevention and personalized medicine.
In the context of COVID-19 testing, biomedical signal analysis has proven essential in improving diagnostic accuracy and the use of sophisticated signal processing techniques. The developments in signal analysis technology provide hope for better and more reliable testing in the future.
"Biomedical signal analysis is an exciting and rapidly evolving field, with the potential to revolutionize healthcare as we know it."
References
1. Acharya, U. R., Fujita, H., Sudarshan, V. K., Oh, S. L., Adam, M., & Tan, J. H. (2017). Biomedical signal and image processing. CRC Press.
2. Chouvarda, I., & Maglaveras, N. (2009). Advances in biomedical signal processing. Journal of biomedical informatics, 42(4), 579-580.
3. Eom, K. J., Choi, H. J., & Kim, J. H. (2019). Deep learning for biomedical signal processing: A review. Sensors, 19(10), 2273.
4. Gargiulo, G., Calvo, R. A., & Milone, D. H. (2012). Advances in biomedical signal processing and analysis. Springer Science & Business Media.
5. Khandoker, A. H., Palaniswami, M., & Begg, R. K. (2018). Analysis of biomedical signals and images. CRC Press.
6. Plesinger, F., Lukes, D., & Ronzhina, M. (2012). Biomedical signal analysis and processing using mathematical morphology. IEEE Transactions on Biomedical Engineering, 59(4), 981-991.
7. Wang, J., Liu, X., & Ding, X. (2019). Biomedical signal processing and control. Biocybernetics and Biomedical Engineering, 39(4), 1031-1032.
COVID-19 Testing References:
1. Bhattacharya, M., Sharma, A. R., Patra, P., Ghosh, P., Sharma, G., Patra, B. C., ... & Chakraborty, C. (2020). Development of a point-of-care diagnostic system for early detection of COVID-19. medRxiv.
2. Carobbio, A., & Magnani, M. (2020). Biosensor technologies for detecting COVID-19. Research in Biomedical Engineering, 36(3), 200-212.
3. Lin, Q., Wen, D., Wu, J., Liu, L., Wu, W., Fang, X., ... & Li, Y. (2020). Artificial intelligence and COVID-19: Deep learning approaches for diagnosis and treatment. IEEE Access, 8, 109581-109595.
4. Valero, T., Ono, J. K., & Fernández, L. R. (2020). Biosensors for COVID-19 diagnosis: A review. Sensors, 20(22), 6599.