Advances in Smartphone-based Computer Vision for Rapid Diagnostic Test Interpretation
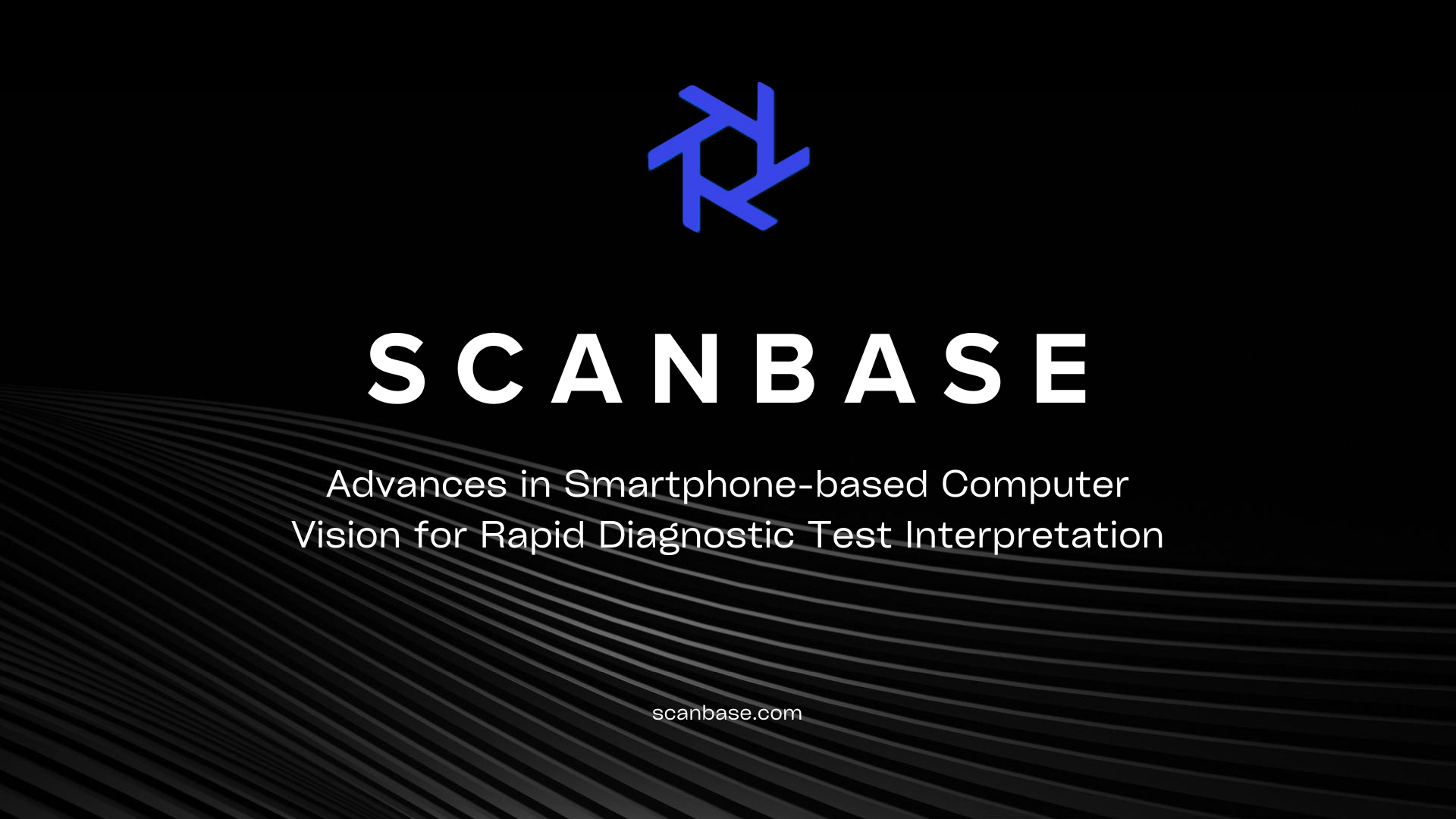
Introduction
In the era of advanced technology and mobile connectivity, smartphones have become an integral part of our daily lives. With their powerful processors, high-resolution cameras, and various sensors, smartphones have evolved into versatile tools that can be utilized for a wide range of applications beyond communication and entertainment. One such application that has gained significant attention is computer vision, particularly in the field of rapid diagnostic test (RDT) interpretation.
Overview of Rapid Diagnostic Tests (RDTs)
Rapid diagnostic tests (RDTs) are simple and cost-effective tools used in healthcare settings to detect the presence of specific diseases or conditions. These tests provide quick results, often within minutes, enabling healthcare professionals to make timely decisions for diagnosis, treatment, and patient management. RDTs are commonly used for infectious diseases such as malaria, HIV/AIDS, dengue fever, and influenza, as well as for pregnancy tests and drug testing.
RDTs typically involve a sample collection, which could be a blood sample, urine sample, or other bodily fluids, and a visual interpretation of the results based on the appearance of color changes or the presence of specific markers. However, the interpretation of RDTs can be subjective and prone to human error, especially in cases where the test results are faint or difficult to discern.
Importance of Rapid Diagnostic Test Interpretation
Accurate and timely interpretation of RDTs is crucial for effective healthcare management. It determines the appropriate course of action, such as the initiation of treatment, referral for further testing, or monitoring of disease progression. Misinterpretation or delayed interpretation can lead to incorrect diagnoses, delayed treatments, and potential adverse health outcomes for patients.
Furthermore, in resource-limited settings where access to well-equipped laboratories and skilled healthcare professionals may be limited, the accurate interpretation of RDTs becomes even more critical. It enables point-of-care testing, allowing healthcare providers to make immediate decisions and provide appropriate care without relying on centralized testing facilities.
Role of Computer Vision in RDT Interpretation
Computer vision, a field of artificial intelligence (AI) and image processing, involves the extraction, analysis, and interpretation of information from visual data, such as images or videos. In the context of RDT interpretation, computer vision techniques can be applied to automate and enhance the accuracy of result interpretation, reducing the reliance on human visual inspection.
The integration of computer vision with smartphones has opened up new possibilities for RDT interpretation. By leveraging the high-resolution cameras and computational capabilities of smartphones, computer vision algorithms can analyze the images of RDTs captured by the smartphone camera and provide automated interpretations. This not only reduces the potential for human error but also enables real-time reporting and data analytics for improved healthcare decision-making.
In the following sections, we will explore the advancements in smartphone-based computer vision for RDT interpretation. We will discuss the integration of smartphone cameras with RDTs, the image acquisition and preprocessing techniques, the automated image analysis algorithms employed, the utilization of deep learning approaches, and the real-time interpretation and reporting capabilities. Additionally, we will delve into the challenges and limitations of smartphone-based computer vision, the applications and impact of this technology in healthcare settings, and the future directions for its development.
Advancements in Smartphone-based Computer Vision for RDT Interpretation
Smartphone-based computer vision has revolutionized the interpretation of rapid diagnostic tests (RDTs) by providing automated and accurate analysis of test results. In this section, we will explore the various advancements in smartphone-based computer vision for RDT interpretation, including the integration of smartphone cameras with RDTs, image acquisition and preprocessing techniques, automated image analysis algorithms, deep learning approaches, and real-time interpretation and reporting capabilities.
Integration of Smartphone Cameras with RDTs
The integration of smartphone cameras with RDTs has been a game-changer in the field of RDT interpretation. By using the smartphone's built-in camera, users can capture high-resolution images of the test strip, enabling precise analysis of the test results. This eliminates the need for specialized imaging equipment and makes RDT interpretation accessible in diverse healthcare settings, including resource-limited areas.
Moreover, the integration of smartphone cameras allows for standardization of image acquisition conditions, ensuring consistent quality and eliminating variations caused by human visual inspection. This standardization enhances the accuracy and reliability of RDT interpretation, leading to more effective healthcare management.
Image Acquisition and Preprocessing Techniques
To ensure optimal image quality and facilitate accurate RDT interpretation, various image acquisition and preprocessing techniques are employed in smartphone-based computer vision systems. These techniques aim to enhance the visibility of the test lines, reduce noise, and improve overall image quality.
Some common image acquisition techniques include adjusting the camera settings, such as exposure and white balance, to optimize image contrast and color accuracy. Additionally, techniques like autofocus and image stabilization help capture clear and focused images, even in challenging lighting conditions or when the user's hand is not steady.
Preprocessing techniques involve image enhancement and noise reduction algorithms. These algorithms can improve the visibility of faint test lines, correct color imbalances, and remove artifacts or unwanted elements from the image. Preprocessing plays a crucial role in ensuring accurate and reliable RDT interpretation, especially in cases where the test results are subtle or difficult to discern.
Automated Image Analysis Algorithms
Automated image analysis algorithms are at the core of smartphone-based computer vision systems for RDT interpretation. These algorithms analyze the captured images and extract relevant information, such as the presence and intensity of test lines, to determine the test result.
Various image processing and pattern recognition techniques are employed in these algorithms. These include edge detection, line segmentation, thresholding, and template matching. These algorithms enable the identification and quantification of test lines, even in complex and overlapping patterns, ensuring accurate interpretation of RDT results.
Furthermore, these algorithms can also handle multiple test zones and interpret different markers simultaneously, allowing for the detection of multiple diseases or conditions in a single RDT. This multi-marker capability enhances the efficiency and cost-effectiveness of RDT interpretation, as it eliminates the need for separate tests for each condition.
Deep Learning Approaches in RDT Interpretation
Deep learning, a subfield of machine learning, has emerged as a powerful approach in smartphone-based computer vision for RDT interpretation. Deep learning algorithms, specifically convolutional neural networks (CNNs), have demonstrated remarkable performance in image analysis tasks, including object recognition and segmentation.
In the context of RDT interpretation, CNNs can be trained to recognize and classify different test lines and markers, leveraging large datasets of labeled RDT images. By learning from vast amounts of data, these networks can generalize and accurately interpret RDT results, even in challenging scenarios.
Deep learning approaches offer several advantages in RDT interpretation. They can handle variations in test line appearance, accommodate different RDT designs, and adapt to changes in lighting conditions. Moreover, deep learning models can be updated and fine-tuned with new data, allowing for continuous improvement in performance and adaptability to emerging RDT technologies.
Real-time Interpretation and Reporting
One of the key advantages of smartphone-based computer vision for RDT interpretation is the ability to provide real-time interpretation and reporting of results. After capturing the image of the RDT, the computer vision system processes the image and generates an automated interpretation within seconds. This immediate feedback enables healthcare professionals to make timely decisions and take appropriate actions, such as initiating treatment or referring the patient for further testing.
Real-time interpretation and reporting also facilitate remote patient monitoring and telemedicine applications. With the integration of smartphone-based computer vision systems, patients can capture and transmit their RDT images to healthcare providers, who can remotely interpret the results and provide necessary guidance or interventions. This remote monitoring capability enhances healthcare accessibility, particularly in underserved or remote areas.
In the next section, we will discuss the challenges and limitations associated with smartphone-based computer vision for RDT interpretation. Despite its numerous advantages, there are certain factors that need to be considered to ensure reliable and effective implementation of this technology.
Challenges and Limitations in Smartphone-based Computer Vision
While smartphone-based computer vision has shown immense potential in rapid diagnostic test (RDT) interpretation, there are several challenges and limitations that need to be addressed for its successful implementation. In this section, we will discuss the key challenges and limitations associated with smartphone-based computer vision and their impact on the accuracy, reliability, and usability of RDT interpretation.
Variability in Image Quality
One of the primary challenges in smartphone-based computer vision for RDT interpretation is the variability in image quality. The quality of images captured by smartphone cameras can be influenced by factors such as lighting conditions, camera settings, user technique, and the presence of artifacts or obstructions. These variations in image quality can affect the accuracy and reliability of RDT interpretation, leading to potential errors or misinterpretations.
To mitigate this challenge, image acquisition techniques and preprocessing algorithms are employed to optimize image quality. However, it is essential to standardize these techniques and algorithms across different smartphone models and settings to ensure consistent image quality and interpretation results.
Complexity of RDT Interpretation
Rapid diagnostic tests (RDTs) can exhibit varying levels of complexity in terms of the number and arrangement of test lines, the presence of control lines, and the interpretation rules for different markers. While computer vision algorithms can handle these complexities to a certain extent, there are cases where the interpretation may be challenging or prone to errors.
For instance, overlapping or faint test lines, variations in line intensity, or the presence of interfering substances can complicate the interpretation process. Additionally, the interpretation rules may differ across different RDT manufacturers or specific test kits, requiring customization or adaptation of the computer vision algorithms.
Addressing the complexity of RDT interpretation requires the development of robust algorithms that can handle a wide range of scenarios and adapt to different test designs. Collaboration between computer vision experts and RDT manufacturers is crucial to ensure accurate and reliable interpretation algorithms that can accommodate the intricacies of various RDTs.
Limited Processing Power and Memory
Smartphones have finite processing power and memory capabilities compared to dedicated imaging equipment or computers. This limitation poses challenges in implementing computationally intensive computer vision algorithms for real-time RDT interpretation.
Complex image processing and deep learning algorithms may require substantial computational resources, which can strain the limited processing power and memory of smartphones. This may result in longer processing times, decreased responsiveness, or even system crashes.
To overcome this limitation, optimization techniques such as algorithm simplification, parallel processing, and model compression can be employed. These techniques aim to reduce the computational requirements of computer vision algorithms without compromising the accuracy and reliability of RDT interpretation. Furthermore, advancements in smartphone hardware, including faster processors and increased memory capacities, can help alleviate these limitations in the future.
Privacy and Security Concerns
The integration of smartphone-based computer vision in healthcare settings raises privacy and security concerns. RDT images captured by smartphones contain sensitive patient information, including personal health data and potentially identifiable information.
To ensure patient privacy and comply with data protection regulations, robust security measures must be implemented. This includes encryption of data transmission, secure storage of images, and adherence to strict access control policies. Additionally, healthcare providers and developers of computer vision systems need to establish and follow ethical guidelines to protect patient privacy and ensure responsible use of RDT interpretation data.
Furthermore, it is important to address the potential risks associated with data breaches or unauthorized access to RDT interpretation systems. Regular security audits, vulnerability assessments, and adherence to industry best practices can help mitigate these risks and instill confidence in the privacy and security of smartphone-based computer vision systems.
In the next section, we will explore the applications and impact of smartphone-based computer vision in RDT interpretation. Despite the challenges and limitations, this technology has the potential to revolutionize healthcare accessibility, point-of-care testing, and public health surveillance.
Applications and Impact of Smartphone-based Computer Vision in RDT Interpretation
Smartphone-based computer vision has vast applications and a significant impact on the interpretation of rapid diagnostic tests (RDTs). This technology has the potential to revolutionize healthcare accessibility, point-of-care testing, remote patient monitoring, public health surveillance, and the affordability of healthcare services. In this section, we will explore these applications and discuss the impact of smartphone-based computer vision in RDT interpretation.
Point-of-Care Testing in Resource-Limited Settings
One of the key applications of smartphone-based computer vision in RDT interpretation is in resource-limited settings. These settings often lack well-equipped laboratories and skilled healthcare professionals, making it challenging to access timely and accurate diagnostic testing. Smartphone-based computer vision systems can bridge this gap by enabling point-of-care testing, where RDTs can be interpreted on-site using smartphones.
The integration of computer vision algorithms with smartphones allows healthcare providers in remote or underserved areas to interpret RDT results rapidly and accurately. This immediate feedback empowers them to make informed decisions regarding patient care, such as initiating appropriate treatment, referring patients for further testing, or providing counseling and education. Point-of-care testing using smartphone-based computer vision improves healthcare accessibility, reduces the burden on centralized testing facilities, and enhances patient outcomes in resource-limited settings.
Remote Patient Monitoring
Smartphone-based computer vision also facilitates remote patient monitoring, particularly in situations where regular monitoring of specific diseases or conditions is necessary. Patients can use their smartphones to capture images of RDTs and transmit them to healthcare providers for interpretation. This allows healthcare professionals to remotely monitor disease progression, treatment effectiveness, and patient compliance.
Remote patient monitoring using smartphone-based computer vision enhances patient convenience and reduces the need for frequent visits to healthcare facilities. It enables timely interventions and adjustments to treatment plans, improving patient outcomes and quality of life. Moreover, it can be especially beneficial for patients living in rural or geographically isolated areas, as well as those with limited mobility or chronic conditions requiring long-term monitoring.
Public Health Surveillance
The integration of smartphone-based computer vision in RDT interpretation has significant implications for public health surveillance. By leveraging the ubiquity of smartphones, public health agencies can collect real-time data on disease prevalence and outbreaks, enabling timely interventions and public health responses.
Through smartphone apps or dedicated platforms, individuals can capture and submit images of their RDT results, providing valuable information about the spread of infectious diseases or the prevalence of certain conditions. These images can be automatically analyzed using computer vision algorithms, allowing for rapid and accurate surveillance of diseases such as malaria, HIV/AIDS, or influenza.
Public health surveillance using smartphone-based computer vision enhances early detection and response to outbreaks, facilitates targeted interventions, and supports evidence-based decision-making. It enables public health agencies to monitor disease trends, track the effectiveness of interventions, and allocate resources efficiently to areas with the greatest need.
Healthcare Accessibility and Affordability
Smartphone-based computer vision has the potential to improve healthcare accessibility and affordability by reducing the reliance on specialized equipment and skilled personnel. The integration of computer vision algorithms with smartphones transforms these devices into portable and cost-effective tools for RDT interpretation.
By eliminating the need for dedicated imaging equipment, smartphone-based computer vision reduces the cost and complexity of diagnostic testing. This not only makes healthcare services more affordable for individuals but also enables the expansion of healthcare services in resource-limited settings where access to expensive laboratory equipment is limited.
Furthermore, smartphone-based computer vision systems can be easily scalable, allowing for widespread adoption and deployment in diverse healthcare settings. The affordability and accessibility of this technology have the potential to democratize diagnostic testing, ensuring that individuals from all walks of life have access to accurate and timely healthcare services.
In the next section, we will explore the future directions and emerging technologies in smartphone-based computer vision for RDT interpretation. The continuous development and innovation in this field hold immense promise for further advancements in healthcare diagnostics and patient care.
Future Directions and Conclusion
The field of smartphone-based computer vision for rapid diagnostic test (RDT) interpretation is constantly evolving, with ongoing advancements and emerging technologies. In this final section, we will explore the future directions and potential developments in this field, as well as provide a summary of the key points discussed in this blog post.
Emerging Technologies and Innovations
The future of smartphone-based computer vision for RDT interpretation holds tremendous potential for further advancements and innovations. Here are some emerging technologies and areas of focus that are shaping the future of this field:
Augmented Reality (AR) and Virtual Reality (VR): The integration of AR and VR technologies with smartphone-based computer vision can enhance the visualization and interpretation of RDT results. These immersive technologies can overlay digital information onto real-world RDT images, providing additional context and guidance for interpretation.
Multi-sensor Integration: Besides cameras, smartphones are equipped with various sensors such as accelerometers, gyroscopes, and ambient light sensors. The integration of data from these sensors with computer vision algorithms can enhance the accuracy and reliability of RDT interpretation by considering additional contextual information.
Internet of Things (IoT) Integration: The integration of smartphone-based computer vision with IoT devices, such as wearable sensors or connected diagnostic devices, can enable seamless data collection, analysis, and interpretation. This integration can enhance the monitoring and management of chronic diseases or remote patient care.
Cloud-based Processing: As smartphone hardware continues to advance, cloud-based processing can offload computationally intensive tasks from smartphones to powerful remote servers. This approach can further enhance the speed and performance of computer vision algorithms, enabling real-time interpretation and analysis of RDT results.
Ethical Considerations
While the future of smartphone-based computer vision for RDT interpretation is promising, it is crucial to address ethical considerations to ensure responsible and equitable deployment of this technology. Some key ethical considerations include:
Data Privacy and Security: As smartphone-based computer vision systems generate and process sensitive health data, it is essential to implement robust privacy and security measures to protect patient information. Adhering to data protection regulations, encryption protocols, and secure storage practices is crucial in maintaining patient privacy.
Algorithm Bias and Fairness: Computer vision algorithms are susceptible to biases inherent in the training data. It is important to ensure that these algorithms are trained on diverse and representative datasets to avoid biased interpretations that may disproportionately affect certain populations or lead to inequitable care.
Transparency and Explainability: As computer vision algorithms become more complex, it is imperative to ensure transparency and explainability in their decision-making processes. Healthcare professionals and patients should have access to understandable explanations of how the algorithms interpret RDT results to build trust and facilitate informed decision-making.
Conclusion
Smartphone-based computer vision has revolutionized the interpretation of rapid diagnostic tests, enabling automated and accurate analysis of test results. The integration of computer vision algorithms with smartphone cameras has transformed these devices into powerful tools for point-of-care testing, remote patient monitoring, public health surveillance, and improved healthcare accessibility.
Through advancements such as image acquisition and preprocessing techniques, automated image analysis algorithms, deep learning approaches, and real-time interpretation and reporting capabilities, smartphone-based computer vision has overcome many challenges associated with RDT interpretation.
However, there are still challenges to address, including image quality variability, the complexity of interpretation, limited processing power and memory, and privacy and security concerns. Overcoming these challenges will require continued research, collaboration between technology developers and healthcare professionals, and the establishment of ethical guidelines.
Looking ahead, the future of smartphone-based computer vision for RDT interpretation holds immense promise. Emerging technologies such as augmented reality, multi-sensor integration, cloud-based processing, and IoT integration are shaping the future of this field. It is crucial to consider ethical considerations, including data privacy, algorithm bias, and transparency, to ensure the responsible and equitable deployment of this technology.
In conclusion, smartphone-based computer vision has the potential to revolutionize healthcare diagnostics, improve patient care, and enhance public health surveillance. By harnessing the power of smartphones and artificial intelligence, we can unlock new possibilities for rapid and accurate interpretation of diagnostic test results, ultimately leading to better healthcare outcomes for individuals and communities worldwide.